輕量級級表格識別算法模型-SLANet 原創(chuàng)
前言
前面文檔介紹了文檔智能上多種思路及核心技術(shù)實(shí)現(xiàn)《??【文檔智能 & RAG】RAG增強(qiáng)之路:增強(qiáng)PDF解析并結(jié)構(gòu)化技術(shù)路線方案及思路??》,
表格識別作為文檔智能的重要組成部分,面臨著復(fù)雜結(jié)構(gòu)和多樣化格式的挑戰(zhàn)。本文介紹的輕量級的表格識別算法模型——SLANet,旨在在保證準(zhǔn)確率的同時提升推理速度,方便生產(chǎn)落地。SLANet綜合了PP-LCNet作為基礎(chǔ)網(wǎng)絡(luò),采用CSP-PAN進(jìn)行特征融合,并引入Attention機(jī)制以實(shí)現(xiàn)結(jié)構(gòu)與位置信息的精確解碼。通過這一框架,SLANet不僅有效減少了計(jì)算資源的消耗,還增強(qiáng)了模型在實(shí)際應(yīng)用場景中的適用性與靈活性。
PP-LCNet
PP-LCNet是一種一種輕量級的CPU卷積神經(jīng)網(wǎng)絡(luò),在圖像分類的任務(wù)上表現(xiàn)良好,具有很高的落地意義。PP-LCNet的準(zhǔn)確度顯著優(yōu)于具有相同推理時間的先前網(wǎng)絡(luò)結(jié)構(gòu)。
模型細(xì)節(jié)
網(wǎng)絡(luò)架構(gòu)
- DepthSepConv塊: 使用MobileNetV1中的DepthSepConv作為基本塊,該塊沒有快捷操作,減少了額外的拼接或逐元素相加操作,從而提高了推理速度。
- 更好的激活函數(shù):將BaseNet中的ReLU激活函數(shù)替換為H-Swish,提升了網(wǎng)絡(luò)性能,同時推理時間幾乎沒有變化。
- SE模塊的適當(dāng)位置: 在網(wǎng)絡(luò)的尾部添加SE模塊,以提高特征權(quán)重,從而實(shí)現(xiàn)更好的準(zhǔn)確性和速度平衡。SE 模塊是 SENet 提出的一種通道注意力機(jī)制,可以有效提升模型的精度。但是在 Intel CPU 端,該模塊同樣會帶來較大的延時,如何平衡精度和速度是我們要解決的一個問題。雖然在 MobileNetV3 等基于 NAS 搜索的網(wǎng)絡(luò)中對 SE 模塊的位置進(jìn)行了搜索,但是并沒有得出一般的結(jié)論,我們通過實(shí)驗(yàn)發(fā)現(xiàn),SE 模塊越靠近網(wǎng)絡(luò)的尾部對模型精度的提升越大。
PP-LCNet 中的 SE 模塊的位置選用了表格中第三行的方案。
- 更大的卷積核: 在網(wǎng)絡(luò)的尾部使用5x5卷積核替代3x3卷積核,以在低延遲和高準(zhǔn)確性之間取得平衡。
實(shí)驗(yàn)表明,更大的卷積核放在網(wǎng)絡(luò)的中后部即可達(dá)到放在所有位置的精度,與此同時,獲得更快的推理速度。PP-LCNet 最終選用了表格中第三行的方案。
- 更大的1x1卷積層: 在全局平均池化(GAP)層后添加一個1280維的1x1卷積層,以增強(qiáng)模型的擬合能力,同時推理時間增加不多。在 GoogLeNet 之后,GAP(Global-Average-Pooling)后往往直接接分類層,但是在輕量級網(wǎng)絡(luò)中,這樣會導(dǎo)致 GAP 后提取的特征沒有得到進(jìn)一步的融合和加工。如果在此后使用一個更大的 1x1 卷積層(等同于 FC 層),GAP 后的特征便不會直接經(jīng)過分類層,而是先進(jìn)行了融合,并將融合的特征進(jìn)行分類。這樣可以在不影響模型推理速度的同時大大提升準(zhǔn)確率。
PP-LCNet系列效果
圖像分類
與其他輕量級網(wǎng)絡(luò)的性能對比
目標(biāo)檢測
CSP-PAN
PP-PicoDet
PAN結(jié)構(gòu)圖:相比于原始的FPN多了自下而上的特征金字塔。
PAN
CSPNet是一種處理的思想,可以和ResNet、ResNeXt和DenseNet結(jié)合。用 CSP 網(wǎng)絡(luò)進(jìn)行相鄰 feature maps 之間的特征連接和融合。
CSP-PAN的引入主要有下面三個目的:
- 增強(qiáng)CNN的學(xué)習(xí)能力
- 減少計(jì)算量
- 降低內(nèi)存占用
SLANet
SLANet結(jié)構(gòu)
原理:
從上圖看,SLANet主要由PP-LCNet + CSP-PAN + Attention組合得到。
- PP-LCNet:CPU 友好型輕量級骨干網(wǎng)絡(luò)
- CSP-PAN:輕量級高低層特征融合模塊
- SLAHead:結(jié)構(gòu)與位置信息對齊的特征解碼模塊,模型預(yù)測兩個值,一是structure_pobs,表格結(jié)構(gòu)的html代碼,二是loc_preds,回歸單元格四個點(diǎn)坐標(biāo)。
核心代碼實(shí)現(xiàn)
import torch
from torch import nn
from torch.nn import functional as F
class SLAHead(nn.Module):
def __init__(self, in_channels=96, is_train=False) -> None:
super().__init__()
self.max_text_length = 500
self.hidden_size = 256
self.loc_reg_num = 4
self.out_channels = 30
self.num_embeddings = self.out_channels
self.is_train = is_train
self.structure_attention_cell = AttentionGRUCell(in_channels,
self.hidden_size,
self.num_embeddings)
self.structure_generator = nn.Sequential(
nn.Linear(self.hidden_size, self.hidden_size),
nn.Linear(self.hidden_size, self.out_channels)
)
self.loc_generator = nn.Sequential(
nn.Linear(self.hidden_size, self.hidden_size),
nn.Linear(self.hidden_size, self.loc_reg_num)
)
def forward(self, fea):
batch_size = fea.shape[0]
# 1 x 96 x 16 x 16 → 1 x 96 x 256
fea = torch.reshape(fea, [fea.shape[0], fea.shape[1], -1])
# 1 x 256 x 96
fea = fea.permute(0, 2, 1)
# infer 1 x 501 x 30
structure_preds = torch.zeros(batch_size, self.max_text_length + 1,
self.num_embeddings)
# 1 x 501 x 4
loc_preds = torch.zeros(batch_size, self.max_text_length + 1,
self.loc_reg_num)
hidden = torch.zeros(batch_size, self.hidden_size)
pre_chars = torch.zeros(batch_size, dtype=torch.int64)
loc_step, structure_step = None, None
for i in range(self.max_text_length + 1):
hidden, structure_step, loc_step = self._decode(pre_chars,
fea, hidden)
pre_chars = structure_step.argmax(dim=1)
structure_preds[:, i, :] = structure_step
loc_preds[:, i, :] = loc_step
if not self.is_train:
structure_preds = F.softmax(structure_preds, dim=-1)
# structure_preds: 1 x 501 x 30
# loc_preds: 1 x 501 x 4
return structure_preds, loc_preds
def _decode(self, pre_chars, features, hidden):
emb_features = F.one_hot(pre_chars, num_classes=self.num_embeddings)
(output, hidden), alpha = self.structure_attention_cell(hidden,
features,
emb_features)
structure_step = self.structure_generator(output)
loc_step = self.loc_generator(output)
return hidden, structure_step, loc_step
class AttentionGRUCell(nn.Module):
def __init__(self, input_size, hidden_size, num_embedding) -> None:
super().__init__()
self.i2h = nn.Linear(input_size, hidden_size, bias=False)
self.h2h = nn.Linear(hidden_size, hidden_size)
self.score = nn.Linear(hidden_size, 1, bias=False)
self.gru = nn.GRU(input_size=input_size + num_embedding,
hidden_size=hidden_size,)
self.hidden_size = hidden_size
def forward(self, prev_hidden, batch_H, char_onehots):
# 這里實(shí)現(xiàn)參考論文https://arxiv.org/pdf/1704.03549.pdf
batch_H_proj = self.i2h(batch_H)
prev_hidden_proj = torch.unsqueeze(self.h2h(prev_hidden), dim=1)
res = torch.add(batch_H_proj, prev_hidden_proj)
res = F.tanh(res)
e = self.score(res)
alpha = F.softmax(e, dim=1)
alpha = alpha.permute(0, 2, 1)
context = torch.squeeze(torch.matmul(alpha, batch_H), dim=1)
concat_context = torch.concat([context, char_onehots], 1)
cur_hidden = self.gru(concat_context, prev_hidden)
return cur_hidden, alpha
class SLALoss(nn.Module):
def __init__(self) -> None:
super().__init__()
self.loss_func = nn.CrossEntropyLoss()
self.structure_weight = 1.0
self.loc_weight = 2.0
self.eps = 1e-12
def forward(self, pred):
structure_probs = pred[0]
structure_probs = structure_probs.permute(0, 2, 1)
# 1 x 30 x 501
# 1 x 501
structure_target = torch.empty(1, 501, dtype=torch.long).random_(30)
structure_loss = self.loss_func(structure_probs, structure_target)
structure_loss = structure_loss * self.structure_weight
loc_preds = pred[1] # 1 x 501 x 4
loc_targets = torch.randn(1, 501, 4)
loc_target_mask = torch.randn(1, 501, 1)
loc_loss = F.smooth_l1_loss(loc_preds * loc_target_mask,
loc_targets * loc_target_mask,
reductinotallow='mean')
loc_loss *= self.loc_weight
loc_loss = loc_loss / (loc_target_mask.sum() + self.eps)
total_loss = structure_loss + loc_loss
return total_loss
參考文獻(xiàn)
1. PP-LCNet: A Lightweight CPU Convolutional Neural Network,https://arxiv.org/pdf/2109.15099.pdf
2. https://github.com/PaddlePaddle/PaddleClas
3. PP-PicoDet: A Better Real-Time Object Detector on Mobile Devices,??https://arxiv.org/abs/2111.00902??4.https://github.com/PaddlePaddle/PaddleOCR/blob/133d67f27dc8a241d6b2e30a9f047a0fb75bebbe/ppstructure/table/README_ch.md
本文轉(zhuǎn)載自公眾號大模型自然語言處理 作者:余俊暉
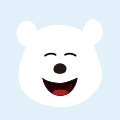