大模型之Agent的初步了解 原創(chuàng)
前言
電影《鋼鐵俠》中的智能助手J.A.R.V.I.S.是一位得力的助手,它不但有強大的理解能力,而且還具備執(zhí)行行動的能力。隨著技術(shù)的不斷進(jìn)步,類似于賈維斯的Agent正在逐步從銀幕走進(jìn)現(xiàn)實。本文將探討Agent的產(chǎn)生背景,并結(jié)合一些代碼示例理解Agent。
Agent的產(chǎn)生背景
一個例子
# 引入Qwen大模型
from utils import get_qwen_models
llm , chat, _ = get_qwen_models()
chat.invoke("現(xiàn)在幾點了?")
運行結(jié)果:
AIMessage(content='我是一個AI模型,無法實時獲取當(dāng)前時間。請您查看您的設(shè)備或詢問周圍的人來獲取準(zhǔn)確的時間。',
response_metadata={'model_name':'qwen-max',
'finish_reason':'stop',
'request_id':'cc11822c-605c-9b94-b443-85d30c9b6c0f',
'token_usage':{'input_tokens':12,'output_tokens':24,'total_tokens':36}},
id='run-bb389bae-6801-4e53-a67c-5d41a53aba8c-0')
通過與大模型的交流,我們會發(fā)現(xiàn)大模型無法回答實時類的問題,例如:
- 今天是幾月幾號?
- 北京現(xiàn)在的天氣是多少?
- ......
大模型可以接受輸入,可以分析&推理、可以輸出文字\代碼\媒體。然而,它無法像人類一樣,擁有規(guī)劃思考能力、運用各種工具與物理世界互動,以及擁有人類的記憶能力。
如何給大模型配備上與物理世界互動的能力,那么會怎樣呢?
Agent的實例
定義工具函數(shù)
第一步:實現(xiàn)一個獲取當(dāng)前時間的函數(shù):
# 定義一個獲取當(dāng)前時間的函數(shù)
def get_datetime() -> str:
"""
跟時期或時間查詢相關(guān)的問題,請調(diào)用此方法
注意:
- 此方法沒有入?yún)? - 返參是字符串形式的日期
"""
# 調(diào)用該函數(shù)
get_datetime()
運行結(jié)果:
'2024-08-29 20:39:34'
定義Prompt模板
第二步:定義使用工具的Prompt模板
from langchain.prompts import PromptTemplate
prompt = PromptTemplate.from_template("""
Answer the following questions as best you can. You have access to the following tools:
{tools}
Use the following format:
Question: the input question you must answer
Thought: you should always think about what to do
Action: the action to take, should be one of [{tool_names}]
Action Input: the input to the action
Observation: the result of the action
... (this Thought/Action/Action Input/Observation can repeat N times)
Thought: I now know the final answer
Final Answer: the final answer to the original input question
Begin!
Question: {input}
Thought:{agent_scratchpad}
""")
創(chuàng)建Agent并調(diào)用
第三步:創(chuàng)建Agent并調(diào)用Agent
from langgraph.prebuilt import create_react_agent
from langchain_core.messages importHumanMessage
agent = create_react_agent(model=chat, tools=[get_datetime])
# 調(diào)用代理
try:
response = agent.invoke({"messages":[HumanMessage(content="現(xiàn)在幾點了?")]})
print(response)
exceptKeyErroras e:
print(f"KeyError: {e}")
exceptExceptionas e:
print(f"發(fā)生錯誤: {e}")
運行結(jié)果:
{'messages':
[
HumanMessage(content='現(xiàn)在幾點了?',id='1e807299-fb54-4fd9-ba16-71b2c45dab98'),
AIMessage(content='', additional_kwargs={
'tool_calls':[{
'function':{
'name':'get_datetime',
'arguments':'{}'},
'index':0,
'id':'call_d21bf57fd5df4314941b9e',
'type':'function'
}]},
response_metadata={
'model_name':'qwen-max',
'finish_reason':'tool_calls',
'request_id':'95c8bf84-3105-91c7-988f-430ef4f3bb84',
'token_usage':{'input_tokens':180,'output_tokens':12,'total_tokens':192}},id='run-9b8c496f-4e2a-4698-bb6d-9fec655c3e37-0',
tool_calls=[{
'name':'get_datetime',
'args':{},
'id':'call_d21bf57fd5df4314941b9e',
'type':'tool_call'}]),
ToolMessage(content='2024-08-30 14:52:29',
name='get_datetime',
id='ce53e86f-252a-4c6d-b33b-1589732ebbbb',
tool_call_id='call_d21bf57fd5df4314941b9e'),
AIMessage(content='現(xiàn)在的時間是14點52分29秒。',
response_metadata={
'model_name':'qwen-max',
'finish_reason':'stop',
'request_id':'adb16577-6a8e-937d-8c13-0d6ba44e5082',
'token_usage':{'input_tokens':220,'output_tokens':17,'total_tokens':237}},
id='run-fd7835ae-b7f2-41d2-b7f9-4a33a51cd67b-0')
]
}
通過上述代碼,可以看到大模型通過Agent被賦予了調(diào)用 ??get_datetime()?
?? 的能力,從而可以回答實時類問題:??現(xiàn)在幾點了??
?
完整代碼如下:
import datetime
from langchain.prompts importPromptTemplate
from langgraph.prebuilt import create_react_agent
from langchain_core.messages importHumanMessage
from utils import get_qwen_models
# 連接大模型
llm , chat, _ = get_qwen_models()
# 定義調(diào)用函數(shù)
defget_datetime()->str:
"""
獲取當(dāng)前時間
"""
now = datetime.datetime.now()
formatted_date = now.strftime("%Y-%m-%d %H:%M:%S")
return formatted_date
# 給大模型綁定工具
bined_chat = chat.bind_tools(tools=[get_datetime])
# 創(chuàng)建使用工具的prompt
prompt =PromptTemplate.from_template("""
Answer the following questions as best you can. You have access to the following tools:
{tools}
Use the following format:
Question: the input question you must answer
Thought: you should always think about what to do
Action: the action to take, should be one of [{tool_names}]
Action Input: the input to the action
Observation: the result of the action
... (this Thought/Action/Action Input/Observation can repeat N times)
Thought: I now know the final answer
Final Answer: the final answer to the original input question
Begin!
Question: {input}
Thought:{agent_scratchpad}
""")
# 創(chuàng)建Agent
agent = create_react_agent(model=chat, tools=[get_datetime])
# 調(diào)用Agent
try:
response = agent.invoke({"messages":[HumanMessage(content="現(xiàn)在幾點了?")]})
print(response)
exceptKeyErroras e:
print(f"KeyError: {e}")
exceptExceptionas e:
print(f"發(fā)生錯誤: {e}")
Agent的簡介
概念
??LLM Agent?
? 是一種能產(chǎn)出不單是簡單文本的 AI 系統(tǒng),它作為一種人工智能體,具備環(huán)境感知能力、自主理解、決策制定及執(zhí)行行動能力的智能實體。簡而言之,它是構(gòu)建于大模型之上的計算機程序,能夠模擬獨立思考過程,靈活調(diào)用各類工具,逐步達(dá)成預(yù)設(shè)目標(biāo)的智能存在。
構(gòu)成
如圖所示,在基于 LLM 的智能體中,LLM 的充當(dāng)著智能體的“大腦”的角色,同時還有 3 個關(guān)鍵部分:
- ?
?規(guī)劃(Planning)?
?: 智能體會把大型任務(wù)分解為子任務(wù),并規(guī)劃執(zhí)行任務(wù)的流程;智能體會對任務(wù)執(zhí)行的過程進(jìn)行思考和反思,從而決定是繼續(xù)執(zhí)行任務(wù),或判斷任務(wù)完結(jié)并終止運行。 - ?
?記憶(Memory)?
?: 短期記憶,是指在執(zhí)行任務(wù)的過程中的上下文,會在子任務(wù)的執(zhí)行過程產(chǎn)生和暫存,在任務(wù)完結(jié)后被清空。長期記憶是長時間保留的信息,一般是指外部知識庫,通常用向量數(shù)據(jù)庫來存儲和檢索。 - ?
?工具使用(Tool use)?
?: 為智能體配備工具 API,比如:計算器、搜索工具、代碼執(zhí)行器、數(shù)據(jù)庫查詢工具等。有了這些工具 API,智能體就可以是物理世界交互,解決實際的問題。
Agent的一些示例
示例1:數(shù)據(jù)庫查詢工具
第一步:使用已封裝好的 ??utils?
? 連接大模型
# 連接大模型
from utils import get_qwen_models
llm, chat, embed = get_qwen_models()
第二步:連接數(shù)據(jù)庫
# 連接數(shù)據(jù)庫
from langchain_community.utilities import SQLDatabase
db = SQLDatabase.from_uri("sqlite:///博金杯比賽數(shù)據(jù).db")
數(shù)據(jù)庫可以從魔塔社區(qū)上拉取到。
第三步:初始化SQL工具包
from langchain_community.agent_toolkits import SQLDatabaseToolkit
# 初始化數(shù)據(jù)庫工具包,傳入數(shù)據(jù)庫連接對象 db 和語言模型 llm
toolkit = SQLDatabaseToolkit(db=db, llm=llm)
# 從工具包中獲取可用的工具,并將其存儲在 tools 變量中
tools = toolkit.get_tools()
第四步:構(gòu)建Prompt
from langchain_core.messages import SystemMessage
SQL_PREFIX = """You are an agent designed to interact with a SQL database.
Given an input question, create a syntactically correct SQLite query to run, then look at the results of the query and return the answer.
Unless the user specifies a specific number of examples they wish to obtain, always limit your query to at most 5 results.
You can order the results by a relevant column to return the most interesting examples in the database.
Never query for all the columns from a specific table, only ask for the relevant columns given the question.
You have access to tools for interacting with the database.
Only use the below tools. Only use the information returned by the below tools to construct your final answer.
You MUST double check your query before executing it. If you get an error while executing a query, rewrite the query and try again.
DO NOT make any DML statements (INSERT, UPDATE, DELETE, DROP etc.) to the database.
To start you should ALWAYS look at the tables in the database to see what you can query.
Do NOT skip this step.
Then you should query the schema of the most relevant tables."""
system_message = SystemMessage(cnotallow=SQL_PREFIX)
以上Prompt可以從https://smith.langchain.com/hub 查詢 ?
?langchain-ai/sql-agent-system-prompt?
? 得到。
第五步:創(chuàng)建Agent
from langgraph.prebuilt import create_react_agent
# 創(chuàng)建Agent,傳入 chat、工具 tools 和 第四步的 prompt
agent_executor = create_react_agent(chat, tools, messages_modifier=system_message)
第六步:調(diào)用Agent并打印執(zhí)行過程
# 查詢
example_query = "請幫我查詢出20210415日,建筑材料一級行業(yè)漲幅超過5%(不包含)的股票數(shù)量"
# 流式處理事件
events = agent_executor.stream(
{"messages": [("user", example_query)]},
stream_mode="values",
)
# 打印流式事件的消息
for event in events:
event["messages"][-1].pretty_print()
執(zhí)行結(jié)果:
================================ HumanMessage=================================
請幫我查詢出20210415日,建筑材料一級行業(yè)漲幅超過5%(不包含)的股票數(shù)量
==================================AiMessage==================================
ToolCalls:
sql_db_list_tables (call_c14a5fc51d324381926311)
Call ID: call_c14a5fc51d324381926311
Args:
tool_input:
=================================ToolMessage=================================
Name: sql_db_list_tables
A股公司行業(yè)劃分表, A股票日行情表,基金份額持有人結(jié)構(gòu),基金債券持倉明細(xì),基金可轉(zhuǎn)債持倉明細(xì),基金基本信息,基金日行情表,基金股票持倉明細(xì),基金規(guī)模變動表,港股票日行情表
==================================AiMessage==================================
ToolCalls:
sql_db_schema (call_f9acd6019db64e93a74987)
Call ID: call_f9acd6019db64e93a74987
Args:
table_names: A股公司行業(yè)劃分表, A股票日行情表
=================================ToolMessage=================================
Name: sql_db_schema
CREATE TABLE "A股公司行業(yè)劃分表"(
"股票代碼" TEXT,
...
[(74,)]
==================================AiMessage==================================
在2021年04月15日,建筑材料一級行業(yè)漲幅超過5%(不包含)的股票數(shù)量為74只。
最終,大模型借助SQL工具,查到了結(jié)果:2021年04月15日,建筑材料一級行業(yè)漲幅超過5%(不包含)的股票數(shù)量為74只。
完整代碼:
from langchain_community.utilities importSQLDatabase
from langchain_community.agent_toolkits importSQLDatabaseToolkit
from langchain_core.messages importSystemMessage
from langgraph.prebuilt import create_react_agent
from utils import get_qwen_models
# 連接大模型
llm, chat, embed = get_qwen_models()
# 連接數(shù)據(jù)庫
db =SQLDatabase.from_uri("sqlite:///博金杯比賽數(shù)據(jù).db")
# 初始化SQL工具包
toolkit =SQLDatabaseToolkit(db=db, llm=llm)
tools = toolkit.get_tools()
# 構(gòu)建Prompt
SQL_PREFIX ="""You are an agent designed to interact with a SQL database.
Given an input question, create a syntactically correct SQLite query to run, then look at the results of the query and return the answer.
Unless the user specifies a specific number of examples they wish to obtain, always limit your query to at most 5 results.
You can order the results by a relevant column to return the most interesting examples in the database.
Never query for all the columns from a specific table, only ask for the relevant columns given the question.
You have access to tools for interacting with the database.
Only use the below tools. Only use the information returned by the below tools to construct your final answer.
You MUST double check your query before executing it. If you get an error while executing a query, rewrite the query and try again.
DO NOT make any DML statements (INSERT, UPDATE, DELETE, DROP etc.) to the database.
To start you should ALWAYS look at the tables in the database to see what you can query.
Do NOT skip this step.
Then you should query the schema of the most relevant tables."""
system_message =SystemMessage(cnotallow=SQL_PREFIX)
# 創(chuàng)建Agent
agent_executor = create_react_agent(chat, tools, messages_modifier=system_message)
# 查詢
example_query ="請幫我查詢出20210415日,建筑材料一級行業(yè)漲幅超過5%(不包含)的股票數(shù)量"
events = agent_executor.stream(
{"messages":[("user", example_query)]},
stream_mode="values",
)
# 查看工具調(diào)用過程
for event in events:
event["messages"][-1].pretty_print()
示例2:維基百科搜索工具
通過對 ??示例1?
? 的分析,我們可以在Langchain官網(wǎng)上找到不少tools工具,接下來我們實現(xiàn)一個維基百科搜索工具。
第一步:安裝依賴包
pip install wikipedia
第二步:執(zhí)行代碼
from langchain_community.tools importWikipediaQueryRun
from langchain_community.utilities importWikipediaAPIWrapper
from langchain_core.messages importSystemMessage
from langgraph.prebuilt import create_react_agent
from utils import get_qwen_models
# 連接大模型
llm, chat, embed = get_qwen_models()
wikipedia =WikipediaQueryRun(api_wrapper=WikipediaAPIWrapper())
tools =[wikipedia]
# 構(gòu)建Prompt
wiki_PREFIX ="""你是一個搜索專家,可以根據(jù)用戶提出的問題,為用戶搜索相應(yīng)的內(nèi)容.
你可以使用維基百科工具來搜索相關(guān)的內(nèi)容。
如果搜索不到對應(yīng)的內(nèi)容,你可以對問題進(jìn)行相關(guān)性引申3個問題,來豐富查詢過程。
搜索后的結(jié)果,請使用markdown的方式返回。
"""
system_message =SystemMessage(cnotallow=wiki_PREFIX)
# 創(chuàng)建Agent
agent_executor = create_react_agent(chat, tools, messages_modifier=system_message)
# 查詢
example_query ="請幫我查詢出美國林肯號航母的相關(guān)信息"
events = agent_executor.stream(
{"messages":[("user", example_query)]},
stream_mode="values",
)
# 查看工具調(diào)用過程
for event in events:
event["messages"][-1].pretty_print()
運行結(jié)果:
請幫我查詢出美國林肯號航母的相關(guān)信息
==================================AiMessage==================================
ToolCalls:
wikipedia (call_78e651d21ea44eafa47741)
Call ID: call_78e651d21ea44eafa47741
Args:
query: USS AbrahamLincoln(CVN-72)
=================================ToolMessage=================================
Name: wikipedia
Page: USS AbrahamLincoln(CVN-72)
Summary: USS AbrahamLincoln(CVN-72) is the fifth Nimitz-class aircraft carrier in the UnitedStatesNavy.She is the third Navy ship to have been named after the former PresidentAbrahamLincoln.Her home port is NAS NorthIsland,SanDiego,California; she is a member of the UnitedStatesPacificFleet.She is administratively responsible to Commander,NavalAirForcesPacific, and operationally serves as the flagship of CarrierStrikeGroup3 and host to CarrierAirWingNine.She was returned to the fleet on 12May2017, marking the successful completion of her Refueling and ComplexOverhaul(RCOH) carried out at NewportNewsShipyard.As of August10,2024, USS AbrahamLincoln and her strike group are being deployed to the MiddleEast as part of the U.S. response to the escalation of tensions between Iran and Israel.
Page: USS AbrahamLincoln
Summary:Two ships have borne the name AbrahamLincoln,in honor of the 16thPresident of the UnitedStates.
USS AbrahamLincoln(SSBN-602), a ballistic missile submarine in service from 1961 to 1981
USS AbrahamLincoln(CVN-72), an aircraft carrier commissioned in1989 and currently in service
Page:CarrierStrikeGroup9
Summary:CarrierStrikeGroup9(CSG-9 or CARSTRKGRU 9) is a U.S.Navy carrier strike group.CommanderCarrierStrikeGroup9(COMCARSTRKGRU 9 or CCSG 9) is responsible for unit-level training, integrated training, and material readiness for the ships and aviation squadrons assigned to the group.The group reports to Commander, U.S.ThirdFleet,which also supervises its pre-deployment training and certification that includes CompositeUnitTrainingExercises.
It is currently assigned to the U.S.PacificFleet.TheNimitz-class aircraft carrier USS TheodoreRoosevelt(CVN-71) is the group's current flagship. Other group units include Carrier Air Wing 11, the Ticonderoga-class cruiser USS Lake Erie (CG-70), and the Arleigh Burke-class destroyer's USS John S.McCain(DDG-56)USS Halsey(DDG-97), and the USS DanielInouye(DDG-118).
The strike group traces its history to Cruiser-DestroyerGroup3, created on 30June1973, by the re-designation of CruiserDestroyerFlotilla11.From2004, the strike group has made multiple MiddleEast deployments providing air forces over Afghanistan and Iraq, as well as conducting MaritimeSecurityOperations.The strike group received the HumanitarianServiceMedalin recognition of its disaster relief efforts inIndonesia during OperationUnifiedAssistancein2004–05.
==================================AiMessage==================================
USS亞伯拉罕·林肯號(USS AbrahamLincoln,舷號CVN-72)是美國海軍第五艘尼米茲級核動力航空母艦,也是第三艘以美國第16任總統(tǒng)亞伯拉罕·林肯命名的軍艦。她的母港位于加利福尼亞州圣迭戈的北島海軍航空站,隸屬于太平洋艦隊。作為航母打擊群3(CSG-3)的旗艦,她搭載了第9艦載機聯(lián)隊(CarrierAirWingNine)。林肯號在完成于紐波特紐斯船廠的燃料補給及復(fù)雜大修(RCOH)后,于2017年5月12日重新歸隊。截至2024年8月10日,亞伯拉罕·林肯號及其打擊群被部署至中東地區(qū),作為美國對伊朗和以色列之間緊張局勢升級應(yīng)對的一部分。
另外,還有兩艘以前總統(tǒng)亞伯拉罕·林肯命名的艦艇:
- USS亞伯拉罕·林肯號(SSBN-602),一艘1961年至1981年間在役的彈道導(dǎo)彈潛艇;
- USS亞伯拉罕·林肯號(CVN-72),即目前仍在服役的航空母艦。
而航母打擊群9(CSG-9)曾是亞伯拉罕·林肯號所屬的打擊群,但目前該打擊群的旗艦為另一艘尼米茲級航母USS西奧多·羅斯福號(USS TheodoreRoosevelt,CVN-71)。
內(nèi)容小結(jié)
- 大模型可以接受輸入,可以分析&推理、可以輸出文字\代碼\媒體,但是對于實時類的問題,沒有能力處理。
- ?
?LLM Agent?
? 是一種能產(chǎn)出不單是簡單文本的 AI 系統(tǒng),它作為一種人工智能體,具備環(huán)境感知能力、自主理解、決策制定及執(zhí)行行動能力的智能實體。 - 創(chuàng)建Agent的大體步驟是:
1. 連接大模型
2. 定義工具函數(shù)
3. 給大模型綁定工具
4. 構(gòu)建工具的prompt
5. 創(chuàng)建Agent
6. 調(diào)用Agent
?
本文轉(zhuǎn)載自公眾號一起AI技術(shù) 作者:熱情的Dongming
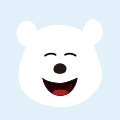