Agno框架介紹:用于構(gòu)建多模態(tài)智能體的輕量庫 原創(chuàng)
在開發(fā)代理式AI時,開發(fā)者往往需要在速度、靈活性和資源利用率間反復(fù)權(quán)衡。本文將向大家介紹Agno——一款用于構(gòu)建多模態(tài)智能體的輕量化框架。其速度號稱比LangGraph快上萬倍,內(nèi)存使用量則僅為1/50。
事實(shí)上,Agno與LangGraph在使用體驗(yàn)上也有很大區(qū)別。本文將以親身體會為基礎(chǔ)對二者進(jìn)行比較,具體介紹它們的不同之處、各自亮點(diǎn)與Agno的獨(dú)特優(yōu)勢。
概述?
- 構(gòu)建TriSage與Marketing Analyst營銷分析師智能體。?
- 如果要求高速、低內(nèi)存占用、多模態(tài)功能以及靈活的模型/工具選項(xiàng),請選擇Agno。?
- 如果偏向基于流程的邏輯或結(jié)構(gòu)化執(zhí)行路徑,或者已經(jīng)與LangChain生態(tài)系統(tǒng)緊密關(guān)聯(lián),請選擇LangGraph。?
Agno有何作用??
基于性能和極簡主義設(shè)計(jì)而成的Agno,從本質(zhì)上講是一款開源且模型中立的智能體框架,專為多模態(tài)任務(wù)而打造——即可以原生處理文本、圖像、音頻和視頻。它的獨(dú)特之處在于便捷快速,在應(yīng)對大量智能體并面對高內(nèi)存用量、工具和向量存儲復(fù)雜度時仍保持良好性能。
核心優(yōu)勢:
- 超快的實(shí)例化速度:Agno中的智能體創(chuàng)建速度平均僅為2微秒,約為LangGraph的1萬倍。?
- 輕量化內(nèi)存占用:Agno智能體平均內(nèi)存占用量為3.75 KiB,僅相當(dāng)于LangGraph智能體的1/50。?
- 原生支持多模態(tài):無需調(diào)整或插件,Agno可直接與各種媒體類型實(shí)現(xiàn)無縫協(xié)作。?
- 模型中立:Agno不關(guān)心用戶使用的是OpenAI、Claude、Gemini抑或是開源大模型,因此避免了特定供應(yīng)商或運(yùn)行時鎖定的問題。?
- 實(shí)時監(jiān)控:可以通過Agno實(shí)時觀察智能體會話及性能,使得調(diào)試和優(yōu)化更加流暢。?
Agno上手實(shí)踐:構(gòu)建TriSage智能體?
Agno的使用體驗(yàn)非常高效,啟動的智能體隊(duì)列不僅可以并行運(yùn)行,還能共享內(nèi)存、工具和知識庫。這些智能體可以專門化并分組為多智能體團(tuán)隊(duì),且內(nèi)存層支持將會話和狀態(tài)存儲在持久數(shù)據(jù)庫當(dāng)中。
真正令人印象深刻的,是Agno如何在不犧牲性能的前提下管理復(fù)雜性。它可以處理現(xiàn)實(shí)世界的智能體編排(如工具鏈、基于RAG檢索或者結(jié)構(gòu)化輸出生成),保證不引發(fā)性能瓶頸。
如果大家用過LangGraph或者類似框架,就會意識到Agno大大降低了啟動延遲與資源消耗。這也成為規(guī)模化應(yīng)用場景下良好性能的實(shí)現(xiàn)關(guān)鍵。下面開始構(gòu)建TriSage智能體。
安裝必要庫
!pip install -U agno
!pip install duckduckgo-search
!pip install openai
!pip install pycountry
以上為安裝必要Python包的shell命令:
- agno: 用于定義和運(yùn)行AI智能體的核心框架。?
- duckduckgo-search: 讓智能體使用DuckDuckGo搜索網(wǎng)絡(luò)。?
- openai: 用于同OpenAI模型(如GPT-4或GPT-3.5)交互。?
必要導(dǎo)入
from agno.agent import Agent
from agno.models.openai import OpenAIChat
from agno.tools.duckduckgo import DuckDuckGoTools
from agno.tools.googlesearch import GoogleSearchTools
from agno.tools.dalle import DalleTools
from agno.team import Team
from textwrap import dedent
API密鑰設(shè)置
from getpass import getpass
OPENAI_KEY = getpass('Enter Open AI API Key: ')
import os
os.environ['OPENAI_API_KEY'] = OPENAI_KEY
- getpass(): 輸入API密鑰的安全方式(不可見)。?
- 將密鑰存儲在環(huán)境內(nèi),以便agno框架在調(diào)用OpenAI API時進(jìn)行獲取。?
web_agent – Searches the Web, writer_agent – Writes the Article, image_agent – Creates Visuals
web_agent = Agent(
name="Web Agent",
role="Search the web for information on Eiffel tower",
model=OpenAIChat(id="o3-mini"),
tools=[DuckDuckGoTools()],
instructions="Give historical information",
show_tool_calls=True,
markdown=True,
)
writer_agent = Agent(
name="Writer Agent",
role="Write comprehensive article on the provided topic",
model=OpenAIChat(id="o3-mini"),
tools=[GoogleSearchTools()],
instructions="Use outlines to write articles",
show_tool_calls=True,
markdown=True,
)
image_agent = Agent(
model=OpenAIChat(id="gpt-4o"),
tools=[DalleTools()],
description=dedent("""\
You are an experienced AI artist with expertise in various artistic styles,
from photorealism to abstract art. You have a deep understanding of composition,
color theory, and visual storytelling.\
"""),
instructions=dedent("""\
As an AI artist, follow these guidelines:
1. Analyze the user's request carefully to understand the desired style and mood
2. Before generating, enhance the prompt with artistic details like lighting, perspective, and atmosphere
3. Use the `create_image` tool with detailed, well-crafted prompts
4. Provide a brief explanation of the artistic choices made
5. If the request is unclear, ask for clarification about style preferences
Always aim to create visually striking and meaningful images that capture the user's vision!\
"""),
markdown=True,
show_tool_calls=True,
)
并入團(tuán)隊(duì)
agent_team = Agent(
team=[web_agent, writer_agent, image_agent],
model=OpenAIChat(id="gpt-4o"),
instructions=["Give historical information", "Use outlines to write articles","Generate Image"],
show_tool_calls=True,
markdown=True,
)
運(yùn)行整體
agent_team.print_response("Write an article on Eiffel towar and generate image", stream=True)
輸出結(jié)果
繼續(xù)輸出
繼續(xù)輸出
I have created a realistic image of the Eiffel Tower. The image captures the
tower's full height and design, ┃
┃ beautifully highlighted by the late afternoon sun. You can view it by
clicking here.?
圖像輸出
上手Agno:構(gòu)建營銷分析師智能體?
此智能體是一套使用Agno的團(tuán)隊(duì)系統(tǒng),將通過DuckDuckGo獲取實(shí)時信息的Web智能體與通過雅虎金融獲取數(shù)據(jù)的金融智能體結(jié)合起來。其由OpenAI模型提供支持,使用表格、markdown及來源支持內(nèi)容提供市場洞察與AI企業(yè)績效,借此保證結(jié)果明確、有深度且公開透明。
from agno.agent import Agent
from agno.models.openai import OpenAIChat
from agno.tools.duckduckgo import DuckDuckGoTools
from agno.tools.yfinance import YFinanceTools
from agno.team import Team
web_agent = Agent(
name="Web Agent",
role="Search the web for information",
model=OpenAIChat(id="o3-mini"),
tools=[DuckDuckGoTools()],
instructinotallow="Always include sources",
show_tool_calls=True,
markdown=True,
)
finance_agent = Agent(
name="Finance Agent",
role="Get financial data",
model=OpenAIChat(id="o3-mini"),
tools=[YFinanceTools(stock_price=True, analyst_recommendatinotallow=True, company_info=True)],
instructinotallow="Use tables to display data",
show_tool_calls=True,
markdown=True,
)
agent_team = Agent(
team=[web_agent, finance_agent],
model=OpenAIChat(id="gpt-4o"),
instructinotallow=["Always include sources", "Use tables to display data"],
show_tool_calls=True,
markdown=True,
)
agent_team.print_response("What's the market outlook and financial performance of top AI companies of the world?", stream=True)
輸出結(jié)果
Agno與LangGraph: 性能對決?
下面我們來看Agno官方文檔中列出的性能細(xì)節(jié):
指標(biāo)? | Agno? | LangGraph? | 差異? |
智能體實(shí)例化用時 | ~2μs | ~20ms | 快~1萬倍 |
每智能體內(nèi)存用量 | ~3.75 KiB | ~137 KiB | 僅為~1/50 |
- 此性能測試采用Python版tracemalloc內(nèi)存分析,硬件平臺為蘋果M4 MacBook Pro筆記本電腦。?
- Agno測量了1000次運(yùn)行的平均實(shí)例化用時及內(nèi)存用量,隔離了智能體代碼以保證結(jié)果準(zhǔn)確。?
這樣的性能優(yōu)勢代表的不只是數(shù)字差異,更是可擴(kuò)展性的新希望。在現(xiàn)實(shí)智能體部署中,用戶往往需要同時啟動成千上萬個智能體,因此每毫秒、每KB都很重要。
雖然LangGraph對于某些流應(yīng)用來說功能強(qiáng)大且結(jié)構(gòu)更加完善,但除非經(jīng)過深度優(yōu)化,否則在這類高負(fù)載場景下往往性能不佳。
所以說……Agno比LangGraph更好??
不一定,具體視應(yīng)用需求而定:
- 如果處理基于流傳輸?shù)闹悄荏w邏輯(例如具有高級控制的分步有向圖),則LangGraph可能會提供更具表現(xiàn)力的結(jié)構(gòu)。?
- 如果需要超快、低內(nèi)存的多模態(tài)智能體執(zhí)行效果,而且環(huán)境的并發(fā)或動態(tài)程度較高,那么Agno將會遙遙領(lǐng)先。?
Agno顯然更傾向于速度和系統(tǒng)層級的效率,而LangGraph則偏重結(jié)構(gòu)化編排與可靠性。不過Agno開發(fā)團(tuán)隊(duì)也非常重視準(zhǔn)確性與可靠性,而且目前正在為此投入時間和精力。
總結(jié)?
從實(shí)踐角度來看,Agno已經(jīng)為生產(chǎn)級工作負(fù)載做好了準(zhǔn)備,尤其是對大規(guī)模構(gòu)建智能體系統(tǒng)的團(tuán)隊(duì)而言。它的實(shí)時性能監(jiān)控、對結(jié)構(gòu)化輸出的支持以及插入內(nèi)存+向量知識的能力,使其成為能夠快速構(gòu)建強(qiáng)大應(yīng)用程序的出色平臺。
但LangGraph的江湖地位也沒有動搖——它的優(yōu)勢在于清晰且面向流程的控制邏輯。不過如果大家遇到了擴(kuò)展障礙,或者需要運(yùn)行成千上萬個智能體又不致擠爆基礎(chǔ)設(shè)施,那么Agno無疑更值得考慮。
原文標(biāo)題:??Agno Framework: A Lightweight Library for Building Multimodal Agents??,作者:Pankaj Singh
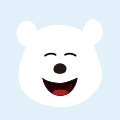