使用Python和Keras創(chuàng)建簡單語音識(shí)別引擎
語音識(shí)別是機(jī)器或程序識(shí)別口語中的單詞和短語并將其轉(zhuǎn)換為機(jī)器可讀格式的能力。通常,這些算法的簡單實(shí)現(xiàn)有一個(gè)有限的詞匯表,它可能只識(shí)別單詞/短語。但是,更復(fù)雜的算法(例如Cloud Speech-to-Text和Amazon Transcribe)具有廣泛的詞匯量,并包含方言、噪音和俚語。
在本文中,我將演示:
- 語音轉(zhuǎn)文字的工作原理
- 如何處理要轉(zhuǎn)錄的音頻
- 使用Keras解決問題的深度學(xué)習(xí)模型
- 一種評(píng)估此模型的方法
- 將預(yù)測(cè)模型集成到項(xiàng)目中的腳本
簡介
語音只是由我們的聲帶引起的空氣周圍振動(dòng)而產(chǎn)生的一系列聲波。這些聲波由麥克風(fēng)記錄,然后轉(zhuǎn)換為電信號(hào)。然后使用高級(jí)信號(hào)處理技術(shù)處理信號(hào),分離音節(jié)和單詞。得益于深度學(xué)習(xí)方面令人難以置信的新進(jìn)展,計(jì)算機(jī)也可以從經(jīng)驗(yàn)中學(xué)習(xí)理解語音。
語音識(shí)別通過聲學(xué)和語言建模使用算法來工作。聲學(xué)建模表示語音和音頻信號(hào)的語言單元之間的關(guān)系;語言建模將聲音與單詞序列進(jìn)行匹配,以幫助區(qū)分聽起來相似的單詞。通常,基于循環(huán)層的深度學(xué)習(xí)模型用于識(shí)別語音中的時(shí)間模式,以提高系統(tǒng)內(nèi)的準(zhǔn)確性。也可以使用其他方法,例如隱馬爾可夫模型(第一個(gè)語音識(shí)別算法是使用這種方法)。在本文中,我將僅討論聲學(xué)模型。
信號(hào)處理
有多種方法可以將音頻波轉(zhuǎn)換為算法可以處理的元素,其中一種方法(在本教程中將使用的一種方法)是在等距的點(diǎn)上記錄聲波的高度:
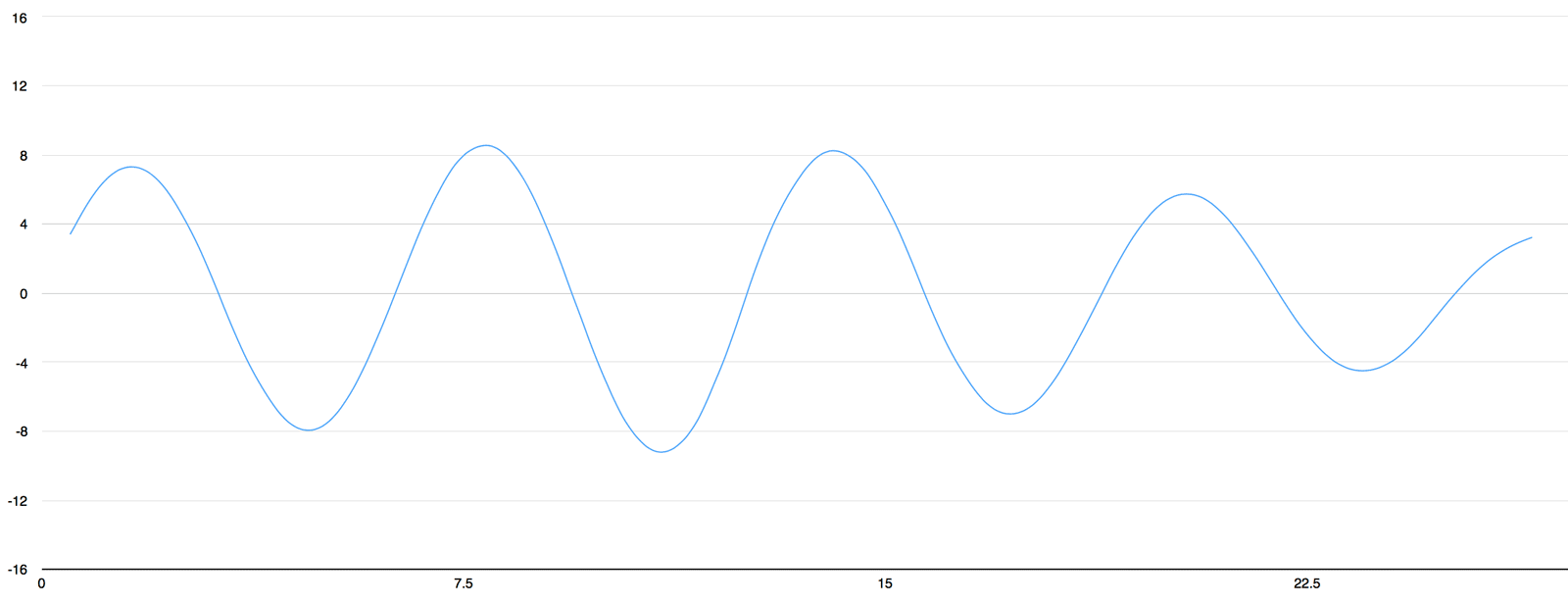
我們每秒讀取數(shù)千次,并記錄一個(gè)代表當(dāng)時(shí)聲波高度的數(shù)字。這是一個(gè)未壓縮的.wav音頻文件。“ CD質(zhì)量”音頻以44.1 kHz(每秒44,100個(gè)讀數(shù))采樣。但是對(duì)于語音識(shí)別而言,16khz(每秒16,000個(gè)樣本)的采樣率足以覆蓋人類語音的頻率范圍。
用這種方法,音頻是通過一個(gè)數(shù)字向量來表示的,其中每個(gè)數(shù)字以1/16000秒的間隔表示聲波的振幅。這個(gè)過程類似于圖像預(yù)處理,如下例所示:
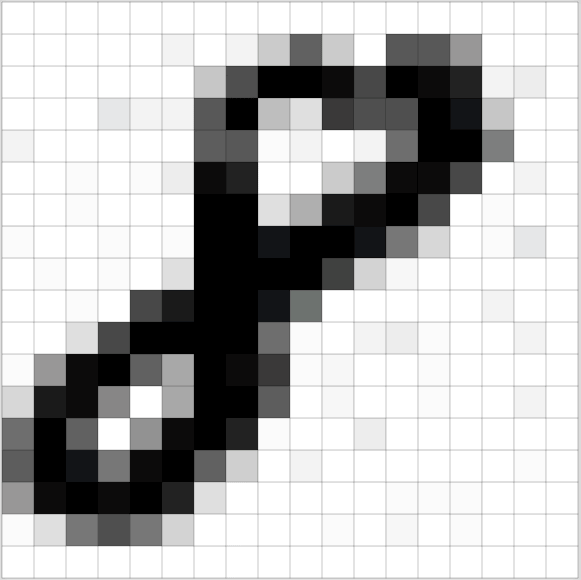
多虧尼奎斯特定理(1933年— 弗拉基米爾·科特爾尼科夫(Vladimir Kotelnikov)),我們知道,只要采樣速度至少是我們要記錄的最高頻率的兩倍,我們就可以使用數(shù)學(xué)方法從間隔采樣中完美重建原始聲波。
Python庫
為了完成這個(gè)任務(wù),我使用Anaconda環(huán)境(Python 3.7)和以下Python庫:
- ipython (v 7.10.2)
- keras (v 2.2.4)
- librosa (v 0.7.2)
- scipy (v 1.1.0)
- sklearn (v 0.20.1)
- sounddevice (v 0.3.14)
- tensorflow (v 1.13.1)
- tensorflow-gpu (v 1.13.1)
- numpy (v 1.17.2)
- from tensorflow.compat.v1 import ConfigProto
- from tensorflow.compat.v1 import Session
- import os
- import librosa
- import IPython.display as ipd
- import matplotlib.pyplot as plt
- import numpy as np
- from scipy.io import wavfile
- import warnings
- config = ConfigProto()
- config.gpu_options.allow_growth = True
- sess = Session(config=config)
- warnings.filterwarnings("ignore")
1.數(shù)據(jù)集
我們?cè)趯?shí)驗(yàn)中使用TensorFlow提供的語音指令數(shù)據(jù)集。它包括由成千上萬不同的人發(fā)出的由30個(gè)短單詞組成的65000個(gè)一秒鐘長的話語。我們將建立一個(gè)語音識(shí)別系統(tǒng),它可以理解簡單的語音命令。您可以從此處下載數(shù)據(jù)集(https://www.kaggle.com/c/tensorflow-speech-recognition-challenge)。
2.預(yù)處理音頻波
在使用的數(shù)據(jù)集中,一些記錄的持續(xù)時(shí)間少于1秒,并且采樣率太高。因此,讓我們閱讀聲波并使用下面的預(yù)處理步驟來解決這個(gè)問題。這是我們要執(zhí)行的兩個(gè)步驟:
- 重采樣
- 刪除少于1秒的短命令
讓我們?cè)谙旅娴腜ython代碼片段中定義這些預(yù)處理步驟:
- train_audio_path = './train/audio/'
- all_wave = []
- all_label = []
- for label in labels:
- print(label)
- waves = [f for f in os.listdir(train_audio_path + '/'+ label) if f.endswith('.wav')]
- for wav in waves:
- samples, sample_rate = librosa.load(train_audio_path + '/' + label + '/' + wav, sr = 16000)
- samples = librosa.resample(samples, sample_rate, 8000)
- if(len(samples)== 8000) :
- all_wave.append(samples)
- all_label.append(label)
由上可知,信號(hào)的采樣率為16000 hz。我們把它重采樣到8000赫茲,因?yàn)榇蠖鄶?shù)語音相關(guān)的頻率都在8000赫茲。
第二步是處理我們的標(biāo)簽,這里我們將輸出標(biāo)簽轉(zhuǎn)換為整數(shù)編碼,將整數(shù)編碼標(biāo)簽轉(zhuǎn)換為one-hot 向量,因?yàn)檫@是一個(gè)多目標(biāo)問題:
- from sklearn.preprocessing import LabelEncoder
- from keras.utils import np_utils
- label_enconder = LabelEncoder()
- y = label_enconder.fit_transform(all_label)
- classes = list(label_enconder.classes_)
- y = np_utils.to_categorical(y, num_classes=len(labels))
預(yù)處理步驟的最后一步是將2D數(shù)組reshape為3D,因?yàn)閏onv1d的輸入必須是3D數(shù)組:
- all_wave = np.array(all_wave).reshape(-1,8000,1)
3.創(chuàng)建訓(xùn)練和驗(yàn)證集
為了執(zhí)行我們的深度學(xué)習(xí)模型,我們將需要生成兩個(gè)集合(訓(xùn)練和驗(yàn)證)。對(duì)于此實(shí)驗(yàn),我使用80%的數(shù)據(jù)訓(xùn)練模型,并在其余20%的數(shù)據(jù)上進(jìn)行驗(yàn)證:
- from sklearn.model_selection import train_test_split
- x_train, x_valid, y_train, y_valid = train_test_split(np.array(all_wave),np.array(y),stratify=y,test_size = 0.2,random_state=777,shuffle=True)
4.機(jī)器學(xué)習(xí)模型架構(gòu)
我使用Conv1d和GRU層來建模用于語音識(shí)別的網(wǎng)絡(luò)。Conv1d是一個(gè)僅在一維上進(jìn)行卷積的卷積神經(jīng)網(wǎng)絡(luò),而GRU的目標(biāo)是解決標(biāo)準(zhǔn)循環(huán)神經(jīng)網(wǎng)絡(luò)的梯度消失問題。GRU也可以看作是LSTM的一個(gè)變體,因?yàn)閮烧叩脑O(shè)計(jì)相似,在某些情況下,可以產(chǎn)生同樣優(yōu)秀的結(jié)果。
該模型基于deepspeech h2和Wav2letter++ algoritms這兩種著名的語音識(shí)別方法。下面的代碼演示了使用Keras提出的模型:
- from keras.layers import Bidirectional, BatchNormalization, CuDNNGRU, TimeDistributed
- from keras.layers import Dense, Dropout, Flatten, Conv1D, Input, MaxPooling1D
- from keras.models import Model
- from keras.callbacks import EarlyStopping, ModelCheckpoint
- from keras import backend as K
- K.clear_session()
- inputs = Input(shape=(8000,1))
- x = BatchNormalization(axis=-1, momentum=0.99, epsilon=1e-3, center=True, scale=True)(inputs)
- #First Conv1D layer
- x = Conv1D(8,13, padding='valid', activation='relu', strides=1)(x)
- x = MaxPooling1D(3)(x)
- x = Dropout(0.3)(x)
- #Second Conv1D layer
- x = Conv1D(16, 11, padding='valid', activation='relu', strides=1)(x)
- x = MaxPooling1D(3)(x)
- x = Dropout(0.3)(x)
- #Third Conv1D layer
- x = Conv1D(32, 9, padding='valid', activation='relu', strides=1)(x)
- x = MaxPooling1D(3)(x)
- x = Dropout(0.3)(x)
- x = BatchNormalization(axis=-1, momentum=0.99, epsilon=1e-3, center=True, scale=True)(x)
- x = Bidirectional(CuDNNGRU(128, return_sequences=True), merge_mode='sum')(x)
- x = Bidirectional(CuDNNGRU(128, return_sequences=True), merge_mode='sum')(x)
- x = Bidirectional(CuDNNGRU(128, return_sequences=False), merge_mode='sum')(x)
- x = BatchNormalization(axis=-1, momentum=0.99, epsilon=1e-3, center=True, scale=True)(x)
- #Flatten layer
- # x = Flatten()(x)
- #Dense Layer 1
- x = Dense(256, activation='relu')(x)
- outputs = Dense(len(labels), activation="softmax")(x)
- model = Model(inputs, outputs)
- model.summary()
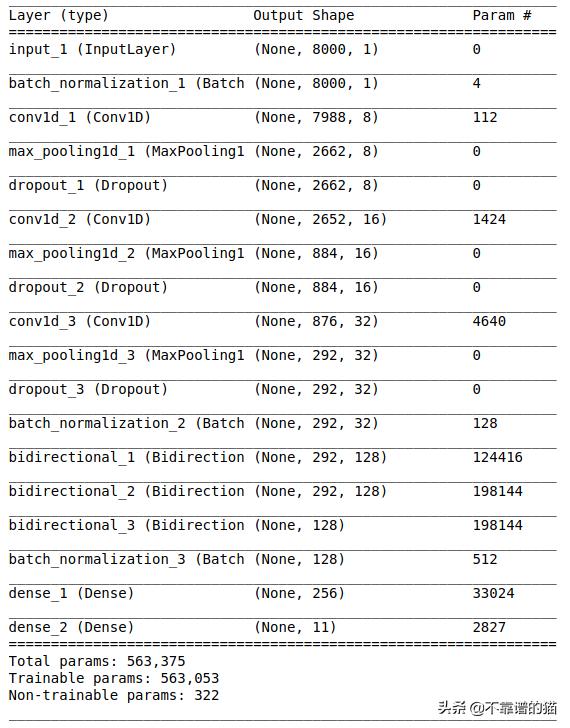
注意:如果僅使用CPU來訓(xùn)練此模型,請(qǐng)用GRU替換CuDNNGRU層。
下一步是將損失函數(shù)定義為分類交叉熵,因?yàn)樗且粋€(gè)多類分類問題:
- model.compile(loss='categorical_crossentropy',optimizer='nadam',metrics=['accuracy'])
Early stopping和模型檢查點(diǎn)是回調(diào),以在適當(dāng)?shù)臅r(shí)間停止訓(xùn)練神經(jīng)網(wǎng)絡(luò)并在每個(gè)epoch后保存最佳模型:
- early_stop = EarlyStopping(monitor='val_loss', mode='min', verbose=1, patience=10, min_delta=0.0001)
- checkpoint = ModelCheckpoint('speech2text_model.hdf5', monitor='val_acc', verbose=1, save_best_only=True, mode='max')
讓我們?cè)?2的batch size上訓(xùn)練機(jī)器學(xué)習(xí)模型,并評(píng)估保留集上的性能:
- hist = model.fit(
- x=x_train,
- y=y_train,
- epochs=100,
- callbacks=[early_stop, checkpoint],
- batch_size=32,
- validation_data=(x_valid,y_valid)
- )
該命令的輸出為:
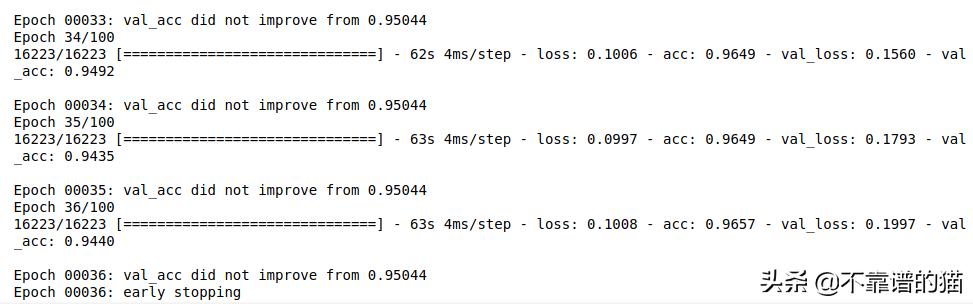
5.可視化
我將依靠可視化來了解機(jī)器學(xué)習(xí)模型在一段時(shí)間內(nèi)的性能:
- from matplotlib import pyplot
- pyplot.plot(hist.history['loss'], label='train')
- pyplot.plot(hist.history['val_loss'], label='test')
- pyplot.legend()
- pyplot.show()
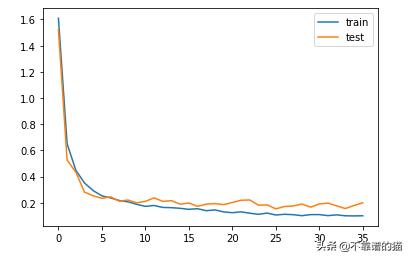
6.預(yù)測(cè)
在這一步中,我們將加載最佳的權(quán)重,并定義識(shí)別音頻和將其轉(zhuǎn)換為文本的函數(shù):
- from keras.models import load_model
- model = load_model('speech2text_model.hdf5')
- def s2t_predict(audio, shape_num=8000):
- prob=model.predict(audio.reshape(1,shape_num,1))
- index=np.argmax(prob[0])
- return classes[index]
對(duì)驗(yàn)證數(shù)據(jù)進(jìn)行預(yù)測(cè):
- import random
- index=random.randint(0,len(x_valid)-1)
- samples=x_valid[index].ravel()
- print("Audio:",classes[np.argmax(y_valid[index])])
- ipd.Audio(samples, rate=8000)
這是一個(gè)提示用戶錄制語音命令的腳本??梢凿浿谱约旱恼Z音命令,并在機(jī)器學(xué)習(xí)模型上測(cè)試:
- import sounddevice as sd
- import soundfile as sf
- samplerate = 16000
- duration = 1 # seconds
- filename = 'yes.wav'
- print("start")
- mydata = sd.rec(int(samplerate * duration), samplerate=samplerate,
- channels=1, blocking=True)
- print("end")
- sd.wait()
- sf.write(filename, mydata, samplerate)
最后,我們創(chuàng)建一個(gè)腳本來讀取保存的語音命令并將其轉(zhuǎn)換為文本:
- #reading the voice commands
- test, test_rate = librosa.load('./test/left.wav', sr = 16000)
- test_sample = librosa.resample(test, test_rate, 4351)
- print(test_sample.shape)
- ipd.Audio(test_sample,rate=8000)
- #converting voice commands to text
- s2t_predict(test_sample)
最后
語音識(shí)別技術(shù)已經(jīng)成為我們?nèi)粘I畹囊徊糠?,但目前仍局限于相?duì)簡單的命令。隨著技術(shù)的進(jìn)步,研究人員將能夠創(chuàng)造出更多能夠理解會(huì)話語音的智能系統(tǒng)。