使用深度學(xué)習(xí)的方法進行人臉解鎖
今天,我們將使用深度學(xué)習(xí)來創(chuàng)建面部解鎖算法。 要完成我們的任務(wù)需要三個主要部分。
- 查找人臉的算法
- 一種將人臉嵌入向量空間的方法
- 比較已編碼人臉的函數(shù)
人臉面孔查找和定位
首先,我們需要一種在圖像中查找人臉的方法。 我們可以使用一種稱為MTCNN(多任務(wù)級聯(lián)卷積網(wǎng)絡(luò))的端到端方法。
只是一點技術(shù)背景,所以稱為Cascaded,因為它由多個階段組成,每個階段都有其神經(jīng)網(wǎng)絡(luò)。 下圖顯示了該框架。
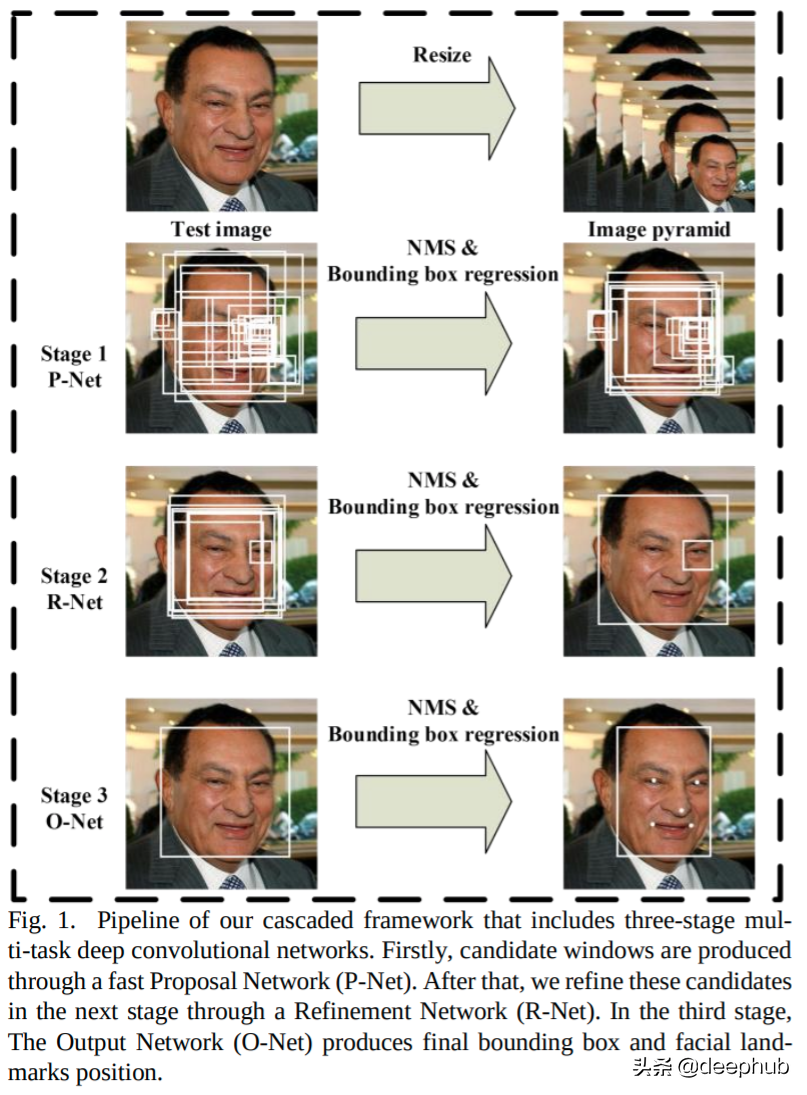
我們依靠facenet-pytorch中的MTCNN實現(xiàn)。
數(shù)據(jù)
我們需要圖像! 我整理了一些照片,萊昂納多·迪卡普里奧和馬特·戴蒙。
遵循PyTorch最佳做法,我使用ImageFolder加載數(shù)據(jù)集。 我創(chuàng)建了MTCNN實例,并使用transform參數(shù)將其傳遞給數(shù)據(jù)集。
我的文件夾結(jié)構(gòu)如下:
- ./faces
- ├── di_caprio
- │ ├── ....jpg
- ├── matt_demon
- │ ├── ....jpg
- └── me
- │ ├── ....jpg
MTCNN自動裁剪輸入并調(diào)整其大小,我使用image_size = 160,因為模型將使用具有該尺寸的圖像進行訓(xùn)練。 我還要添加18像素的邊距,以確保我們包括整個臉部。
- import torch
- import torchvision.transforms as T
- import matplotlib.pyplot as plt
- from torch.utils.data import Dataset, DataLoader
- from torchvision.datasets import ImageFolder
- from facenet_pytorch import MTCNN, InceptionResnetV1
- from pathlib import Path
- from typing import Union, Callable
- data_root = Path('.')
- # create the MTCNN network
- transform = MTCNN(image_size=160, margin=18)
- ds = ImageFolder(root=data_root / 'faces', transform=transform)
- # our dataset is so small that the batch_size can equal to its lenght
- dl = DataLoader(ds, batch_size=len(ds))
- ds[1]
ds結(jié)構(gòu)如下:
- (tensor([[[ 0.9023, 0.9180, 0.9180, ..., 0.8398, 0.8242, 0.8242], [ 0.9023, 0.9414, 0.9492, ..., 0.8555, 0.8320, 0.8164], [ 0.9336, 0.9805, 0.9727, ..., 0.8555, 0.8320, 0.7930], ..., [-0.7070, -0.7383, -0.7305, ..., 0.4102, 0.3320, 0.3711], [-0.7539, -0.7383, -0.7305, ..., 0.3789, 0.3633, 0.4102], [-0.7383, -0.7070, -0.7227, ..., 0.3242, 0.3945, 0.4023]], [[ 0.9492, 0.9492, 0.9492, ..., 0.9336, 0.9258, 0.9258], [ 0.9336, 0.9492, 0.9492, ..., 0.9492, 0.9336, 0.9258], [ 0.9414, 0.9648, 0.9414, ..., 0.9570, 0.9414, 0.9258], ..., [-0.3633, -0.3867, -0.3867, ..., 0.6133, 0.5352, 0.5820], [-0.3945, -0.3867, -0.3945, ..., 0.5820, 0.5742, 0.6211], [-0.3711, -0.3633, -0.4023, ..., 0.5273, 0.6055, 0.6211]], [[ 0.8867, 0.8867, 0.8945, ..., 0.8555, 0.8477, 0.8477], [ 0.8789, 0.8867, 0.8789, ..., 0.8789, 0.8633, 0.8477], [ 0.8867, 0.9023, 0.8633, ..., 0.9023, 0.8789, 0.8555], ..., [-0.0352, -0.0586, -0.0977, ..., 0.7617, 0.7070, 0.7461], [-0.0586, -0.0586, -0.0977, ..., 0.7617, 0.7617, 0.8086], [-0.0352, -0.0352, -0.1211, ..., 0.7227, 0.8086, 0.8086]]]), 0)
數(shù)據(jù)集返回張量。 讓我們可視化所有輸入。 它們已通過MTCNN圖像進行了歸一化,最后一行的最后三張圖像是我自己的自拍照:)
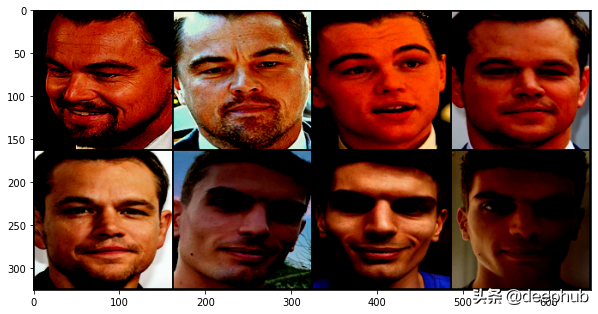
嵌入向量空間
我們的數(shù)據(jù)已準備就緒。 為了比較人臉并找出兩個人臉是否相似,我們需要在向量空間中對它們進行編碼,如果兩個人臉相似,則與它們相關(guān)聯(lián)的兩個向量也都相似(接近)。
我們可以使用在一個著名的人臉數(shù)據(jù)集(例如vgg_face2)上訓(xùn)練的模型,并使用分類頭之前的最后一層的輸出(潛在空間)作為編碼器。
在這些數(shù)據(jù)集之一上訓(xùn)練的模型必須學(xué)習(xí)有關(guān)輸入的重要特征。 最后一層(在完全連接的層之前)對高級功能進行編碼。 因此,我們可以使用它將輸入嵌入向量空間中,希望相似圖像彼此靠近。
詳細地,我們將使用在vggface2數(shù)據(jù)集上訓(xùn)練的初始Resnet。 嵌入空間的尺寸為512。
- resnet = InceptionResnetV1(pretrained='vggface2').eval()
- with torch.no_grad():
- for (imgs, labels) in dl:
- embs = resnet(imgs)
- break
- embs.shape
- torch.Size([8, 512])
完美,我們有8張圖片,我們獲得了8個矢量
相似度計算
為了比較向量,我們可以使用cosine_similarity來查看它們彼此之間的距離。 余弦相似度將輸出[-1,1]之間的值。 在樸素的情況下,兩個比較的向量相同,它們的相似度為1。因此,最接近1的相似度。
現(xiàn)在,我們可以在數(shù)據(jù)集中找到每對之間的所有距離。
- import seaborn as sns
- import numpy as np
- similarity_matrix = torch.zeros(embs.shape[0], embs.shape[0])
- for i in range(embs.shape[0]):
- for j in range(embs.shape[0]):
- similarity_matrix[i,j] = torch.cosine_similarity(embs[i].view(1, -1), embs[j].view(1, -1))
- fig = plt.figure(figsize=(15, 15))
- sns.heatmap(similarity_matrix.numpy(), annot = True,)
- numicons = 8
- for i in range(numicons):
- axicon = fig.add_axes([0.12+0.082*i,0.01,0.05,0.05])
- axicon.imshow(un_normalize(ds[i][0]).permute(1,2,0).numpy())
- axicon.set_xticks([])
- axicon.set_yticks([])
- axicon = fig.add_axes([0, 0.15 + 0.092 * i,.05,0.05])
- axicon.imshow(un_normalize(ds[len(ds) - 1 - i][0]).permute(1,2,0).numpy())
- axicon.set_xticks([])
- axicon.set_yticks([])
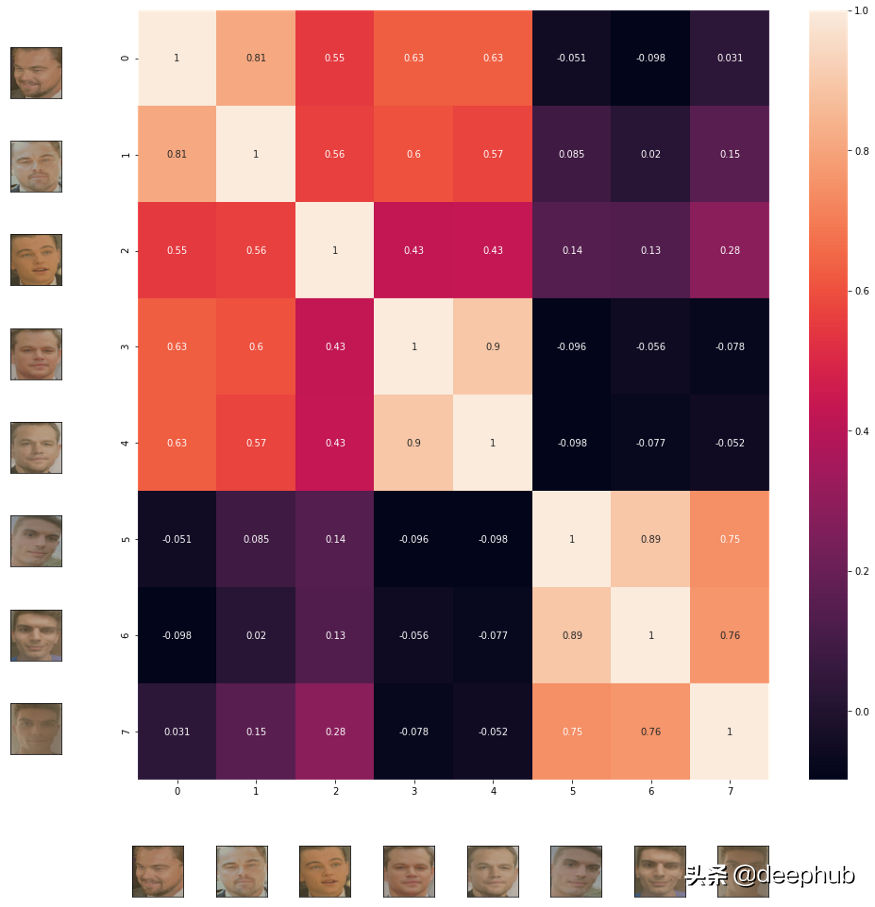
顯然,我與Matt或Leo不太相似,但是它們有一些共同點!
我們可以更加深入,在嵌入向量中運行PCA并將圖像投影到二維平面中
- from matplotlib.offsetbox import OffsetImage, AnnotationBbox
- def pca(x: torch.Tensor, k: int = 2) -> torch.Tensor:
- """
- From http://agnesmustar.com/2017/11/01/principal-component-analysis-pca-implemented-pytorch/
- """
- # preprocess the data
- X_mean = torch.mean(x, 0)
- x = x - X_mean.expand_as(x)
- # svd
- U, S, V = torch.svd(torch.t(x))
- return torch.mm(x, U[:, :k])
- points = pca(embs, k=2)
- plt.rcParams["figure.figsize"] = (12,12)
- fig, ax = plt.figure(), plt.subplot(111)
- plt.scatter(points[:,0], points[:,1])
- for i, p in enumerate(points):
- x, y = p[0], p[1]
- img = un_normalize(ds[i][0])
- img_np = img.permute(1, 2, 0).numpy().squeeze()
- ab = AnnotationBbox(OffsetImage(img_np, zoom=0.6), (x, y), frameon=False)
- ax.add_artist(ab)
- plt.plot()
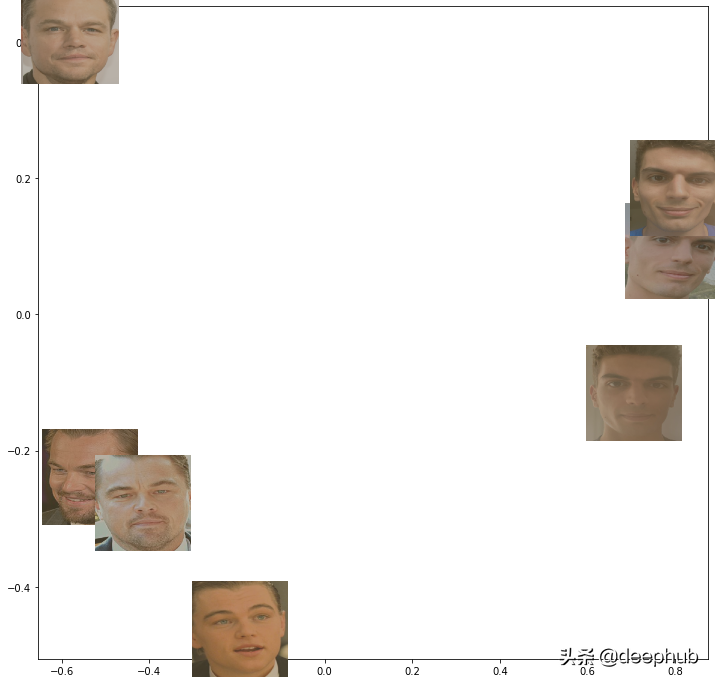
我們將512維壓縮為2,所以我們丟失了很多數(shù)據(jù)。
好的,我們有一種方法來找到臉,看看它們是否彼此相似,現(xiàn)在我們可以創(chuàng)建我們的臉解鎖算法。
我的想法是取n張允許的人的圖像,在嵌入空間中找到中心,選擇一個閾值,看d看中心和新圖像之間的余弦相似度是小于還是大于它。
- from dataclasses import dataclass, field
- from typing import List, Callable
- from PIL import Image
- @dataclass
- class FaceUnlock:
- images: List[Image.Image] = field(default_factory = list)
- th: float = 0.8
- transform: Callable = MTCNN(image_size=160, margin=18)
- embedder: torch.nn.Module = InceptionResnetV1(pretrained='vggface2').eval()
- center: torch.Tensor = None
- def __post_init__(self):
- faces = torch.stack(list(map(self.transform, self.images)))
- embds = self.embedder(faces)
- self.center = embds.sum(0) / embds.shape[0]
- def __call__(self, x: Image.Image) -> bool:
- face = self.transform(x)
- emb = self.embedder(face.unsqueeze(0))
- similarity = torch.cosine_similarity(emb.view(1, -1), self.center.view(1, -1))
- is_me = similarity > self.th
- return is_me, similarity
- # load pictures of myself
- me = data_root / 'faces' / 'me'
- images = list(map(Image.open, me.glob('*')))
- # initialize face unlock with my images
- face_unlock = FaceUnlock(images)
- from ipywidgets import interact, interactive, fixed, interact_manual
- def unlock_with_filepath(path):
- img = Image.open(path)
- is_me, similarity = face_unlock(img)
- print(f"{'' if is_me else ''} similarity={similarity.item():.3f}")
- fig = plt.figure()
- plt.imshow(img)
- plt.plot()
- test_root = data_root / 'faces_test'
- interact(unlock_with_filepath, path=list(test_root.glob('*')))
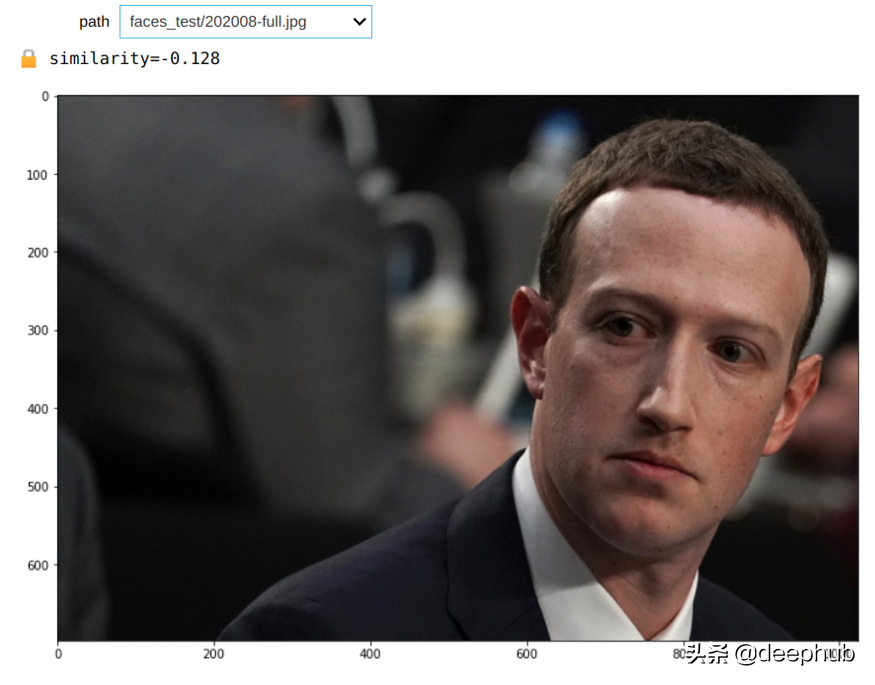
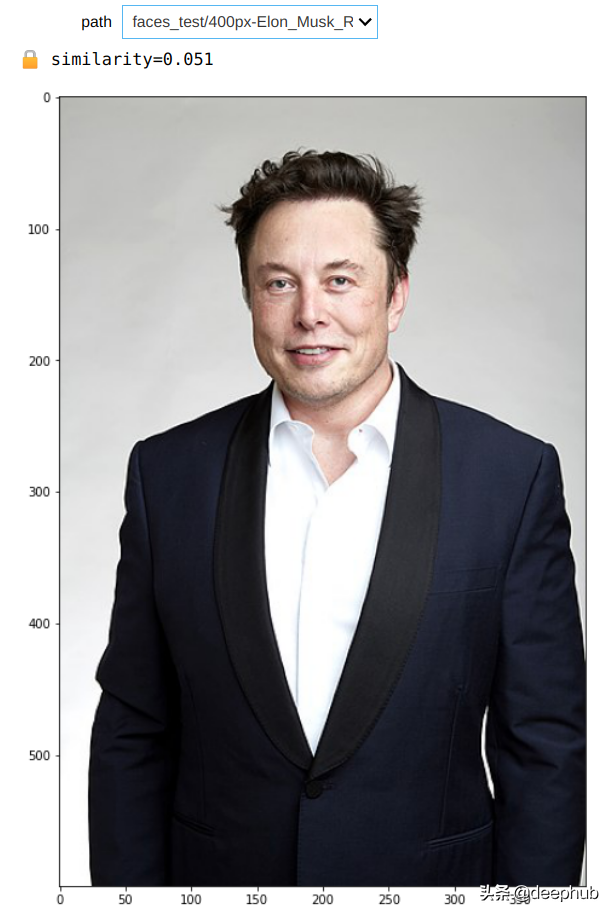
相似度得分比以前的圖像高,所以我猜是真的!
讓我們嘗試自己的新自拍
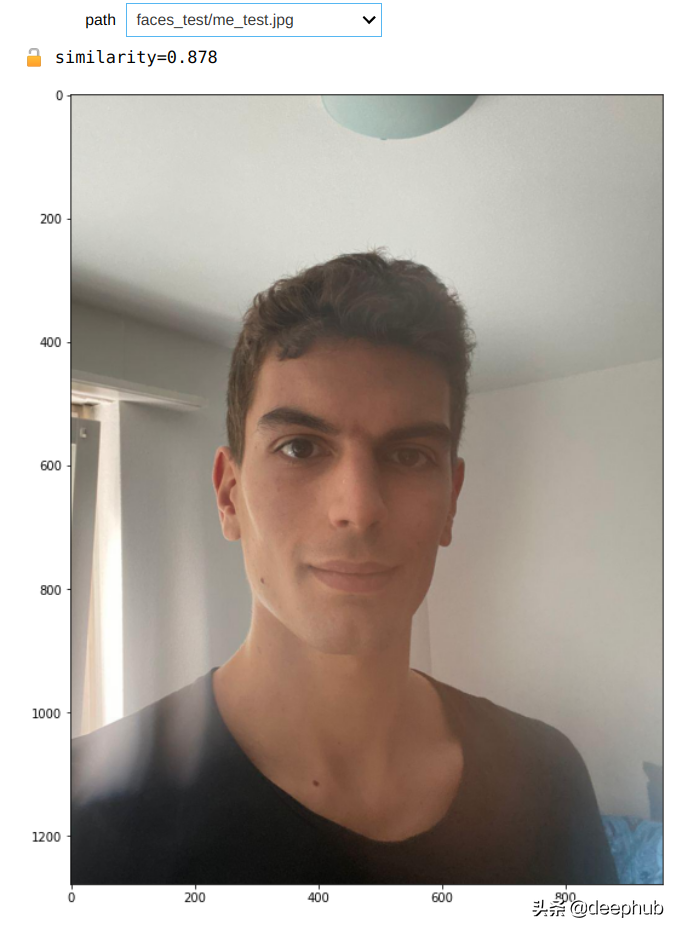
總結(jié)
我們已經(jīng)看到了一種僅使用2D數(shù)據(jù)(圖像)創(chuàng)建人臉解鎖算法的有吸引力的方法。 它依靠神經(jīng)網(wǎng)絡(luò)對相似面孔彼此靠近的高維向量空間中的裁剪面孔進行編碼。 但是,我不知道該模型是如何訓(xùn)練的,并且可能很容易弄糊涂(即使在我的實驗中該算法效果很好)。
如果在沒有數(shù)據(jù)擴充的情況下訓(xùn)練模型怎么辦? 然后,可能只是翻轉(zhuǎn)同一個人可能會破壞潛在的表示。
更加健壯的訓(xùn)練例程將是無監(jiān)督的(類似于BYOL),它嚴重依賴于數(shù)據(jù)增強。