ShareGPT4V作者團(tuán)隊(duì)又一力作!百萬高質(zhì)量視頻-字幕數(shù)據(jù)助力社區(qū)提升多模態(tài)大模型視頻理解及生成能力
繼Sora官宣之后,多模態(tài)大模型在視頻生成方面的應(yīng)用簡直就像井噴一樣涌現(xiàn)出來,LUMA、Gen-3 Alpha等視頻生成模型展現(xiàn)了極佳質(zhì)量的藝術(shù)風(fēng)格和視頻場景的細(xì)節(jié)雕刻能力,文生視頻、圖生視頻的新前沿不斷被擴(kuò)展令大家驚喜不已,抱有期待。
最近,來自中國科學(xué)技術(shù)大學(xué)、北京大學(xué)、上海 AI Lab等團(tuán)隊(duì)的研究人員發(fā)布了引人矚目的 ShareGPT4Video系列,旨在提升視頻理解和生成能力。
- 論文鏈接: ???https://arxiv.org/abs/2406.04325v1???
- 項(xiàng)目鏈接:???https://sharegpt4video.github.io/???
- 數(shù)據(jù)集鏈接:???https://huggingface.co/datasets/ShareGPT4Video/ShareGPT4Video???
- 代碼鏈接: ???https://github.com/ShareGPT4Omni/ShareGPT4Video???
- Demo鏈接: ???https://huggingface.co/spaces/Lin-Chen/ShareCaptioner-Video???
在過去半年中,圖像-語言多模態(tài)領(lǐng)域在ShareGPT4V的高質(zhì)量圖像-字幕數(shù)據(jù)集的推出后逐漸意識到詳細(xì)、準(zhǔn)確的圖像-字幕數(shù)據(jù)對于對齊圖像與語言模態(tài)的重要性。ShareGPT4V數(shù)據(jù)集推出至今已在HuggingFace平臺的VQA dataset track上獲得了歷史第二高的點(diǎn)贊數(shù)。
建立在高質(zhì)量的ShareGPT4V數(shù)據(jù)集上,圖像理解和圖像生成社區(qū)也都取得一些突破性的進(jìn)展,例如InternVL-Chat-V1.5與PixArt-Σ等工作。
受ShareGPT4V數(shù)據(jù)集在圖文多模態(tài)領(lǐng)域的成功所鼓舞,原作者團(tuán)隊(duì)把目光再次投向視頻多模態(tài)領(lǐng)域。視頻多模態(tài)領(lǐng)域中閉源商業(yè)模型一直處于斷層領(lǐng)先的地位,一方面,OpenAI和谷歌近期接連的兩場發(fā)布會,把AI視頻推理卷到了新高度。另一方面,OpenAI的Sora文生視頻模型則把文生視頻帶到了一個全新的高度。
研究者們認(rèn)為閉源模型對于視頻理解和視頻生成領(lǐng)域的巨大領(lǐng)先同樣離不開詳細(xì)高質(zhì)量的視頻-字幕數(shù)據(jù)。因此,該研究團(tuán)隊(duì)再次致力于為視頻獲取大量詳細(xì)而精確的字幕,提升大型視頻語言模型的視頻理解能力和文生視頻模型的視頻生成能力。
目前,該研究在HuggingFace的6月7日Daily Papers中位居榜首,并且在代碼公布后迅速獲得500+ Star,得到了國內(nèi)外的一致關(guān)注。
研究者們認(rèn)為用現(xiàn)有的閉源模型生成高質(zhì)量視頻描述的挑戰(zhàn)有三個方面:
- 清晰地理解幀間的時序變化。
- 詳細(xì)準(zhǔn)確地描述幀內(nèi)內(nèi)容。
- 對任意長度視頻的可擴(kuò)展性。
為此,研究者們精心設(shè)計(jì)了一種差分滑窗視頻描述(Differential Sliding-Window Captioning, DiffSW)策略,該策略可以穩(wěn)定且高效地為任意分辨率,寬高比和長度的視頻生成高質(zhì)量描述。
圖 1:差分滑動窗口視頻描述生成
具體而言,研究者們每次送入GPT4V的輸入是當(dāng)前關(guān)鍵幀,上一關(guān)鍵幀以及上一關(guān)鍵幀對應(yīng)的差分描述,旨在讓GPT4V根據(jù)觀察兩幀之間的時間與空間變化總結(jié)出當(dāng)前幀相對于上一幀的重要空間、時序變化,即當(dāng)前幀與上一幀對應(yīng)的差分描述。最終,所有差分描述會連同時間戳一起送入GPT4中從而總結(jié)出最終的關(guān)于整個視頻的高質(zhì)量字幕。
該研究團(tuán)隊(duì)展示了幾個示例:
- Caption 1:
The video segment documented a significant event in Kochi, Kerala, where 2 buildings razed in Kochi. The broadcast began with a split-screen presentation: on one side, thick clouds of dust were seen billowing into the sky, marking the onset of the demolition process, while on the other side, reporter Gopikrishnan provided live coverage, indicated by "BREAKING NEWS" captions and a consistent timestamp of "11:10 AM." The news ticker at the bottom of the screen simultaneously ran other global events, maintaining a flow of information. As the video progresses, the split-screen footage of the razed house turns into a close-up. A notable change in the headline to "KOCHI FLATS RAZED" signaled the demolition's culmination. A brief interlude offered a visual contradiction by showcasing the flats presumably before their demolition, providing a stark before and after comparison. As the video progressed, the left building's collapse initiated a dramatic alteration in the skyline, marked by significant dust plumes. Subsequently, another building was shown partially collapsing amid debris, fully obscured by dust in seconds, with surrounding greenery remaining untouched. This transitioned into a graphic interlude featuring the "India Today" logo, briefly pausing the live footage. Resuming to the aftermath, split imagery displayed the rubble and ongoing smoke. Then, the imagery continued to juxtapose the scenes of destruction against intact high-rise buildings nearby. The narrative was augmented by the revelation that the Supreme Court directed the demolition within a broader national news context. Throughout, the report maintained a real-time approach, threading continuity and urgency across the unfolding event's documentation.
- Caption 2:
The video begins with an individual seated on a gray couch in a cozy domestic setting, about to unbox a product from a red CCM-branded box placed on a white table in front of them. Initially, the person is seen drinking from a blue can, indicating a casual atmosphere. Soon after, the individual shifts attention from the can to the red box, signifying the start of the unboxing process. The red box, initially closed, gradually becomes the focal point as the person prepares to open it, conveying a build-up of anticipation. As the video progresses, the box is flipped over and then opened, revealing its content still hidden under white tissue paper adorned with prints, adding to the suspense. The individual’s engagement with the box evolves, from initially preparing to open it, to actively delving into its contents. A momentary pause in activity is captured before the anticipation culminates with the individual lifting an object from the box. This object, identifiable by a yellow label, is then examined closely by the person, indicating a thorough inspection or perusal of the product or its packaging. Throughout the video, the surrounding environment remains consistent and undisturbed, with household items like a potted plant and a wall clock maintaining the setting's homely ambiance. The camera’s perspective remains fixed, focusing on the unfolding unboxing event without any movement, thus allowing the viewer to observe the narrative closely. Another partially open brown box is visible beside the main red box, though its role or contents are not elaborated upon. The video encapsulates the anticipation, action, and reveal inherent to unboxing experiences in a home setting.
通過這一方法,研究者們推出了大型“視頻-文本描述”數(shù)據(jù)集--ShareGPT4Video數(shù)據(jù)集,其中包括4萬條(共291小時)由GPT-4V標(biāo)注的視頻數(shù)據(jù)。這些數(shù)據(jù)涵蓋了廣泛的類別,生成的描述包含豐富的世界知識,對象屬性,攝像機(jī)運(yùn)動,以及詳細(xì)和精確的事件時間描述。
圖 2 :(a)數(shù)據(jù)集涵蓋廣泛的內(nèi)容,包括野生動物、烹飪、體育、風(fēng)景、第一人稱人類活動、自動駕駛場景等。(c) 字幕的字?jǐn)?shù)主要在 200 到 400 之間,提供了豐富的時間信息,可以很好地完成視頻理解和生成任務(wù)。
在ShareGPT4Video數(shù)據(jù)集的基礎(chǔ)上,為了進(jìn)一步擴(kuò)大數(shù)據(jù)集規(guī)模以及便于開源社區(qū)在自有數(shù)據(jù)上的使用,研究者們進(jìn)一步設(shè)計(jì)開發(fā)了ShareCaptioner-Video,一個能夠有效地為任意視頻生成高質(zhì)量描述的多功能多模態(tài)大模型。
圖 3:ShareCaptioner-Video 是一款四合一的特殊視頻描述模型,具有以下功能:滑動窗口生成視頻描述、快速生成視頻描述、視頻片段對應(yīng)描述整合,提示詞生成詳細(xì)描述
具體而言,滑窗視頻描述功能可以擔(dān)任GPT4V收集標(biāo)注數(shù)據(jù)中的全部角色,并且通過滑窗的方式來產(chǎn)生差分描述并匯總出最終的字幕??焖僖曨l描述功能則是把所有關(guān)鍵幀沿豎直方向拼成一張長圖一次性產(chǎn)生最終的字幕,在略微犧牲性能的情況下大幅提升標(biāo)注速度。視頻片段總結(jié)功能則可以在對完整視頻進(jìn)行一次滑窗描述后,對其中任意的視頻片段直接總結(jié)出字幕而不需要再次進(jìn)行滑窗描述過程。
在得到了優(yōu)異的視頻描述模型后,研究者們用它進(jìn)一步標(biāo)注了480萬條,總時長3000小時的豐富的視頻數(shù)據(jù)。這些視頻具有較高的美學(xué)評分以及較少的轉(zhuǎn)場效果,旨在為視頻生成任務(wù)服務(wù)。
表1:由 ShareCaptioner-Video 標(biāo)注的480萬條視頻數(shù)據(jù)的構(gòu)成
實(shí)驗(yàn)
在視頻理解方面,研究者們首先通過簡單的等量替換實(shí)驗(yàn),驗(yàn)證了ShareGPT4Video數(shù)據(jù)集在幾種當(dāng)前LVLM架構(gòu)上的有效性。研究者們把VideoChatGPT數(shù)據(jù)集中100K視頻訓(xùn)練數(shù)據(jù)中的與詳細(xì)caption相關(guān)的28K數(shù)據(jù)等量替換成ShareGPT4Video數(shù)據(jù)集中的子集。從下表可以看到,通過簡單的數(shù)據(jù)替換,僅僅是字幕數(shù)據(jù)質(zhì)量上的提升便可以一致地為不同架構(gòu)、不同規(guī)模的視頻理解多模態(tài)大模型帶來顯著的性能增益。
表 2:ShareGPT4Video數(shù)據(jù)集在各模型架構(gòu)上均能產(chǎn)生性能增益
之后,研究者們自主收集了153K的視頻VQA數(shù)據(jù),并結(jié)合ShareGPT4Video數(shù)據(jù)集中與視頻理解相關(guān)的28K高質(zhì)量字幕數(shù)據(jù),提出了新的LVLM ShareGPT4Video-8B。僅需8卡以及5個小時的訓(xùn)練開銷,即可在多項(xiàng)Benchmark上取得優(yōu)異的結(jié)果。
表 3 :TempCompass上性能對比
表 4 :VideoBench上性能對比
表 5:MVBench上性能對比
即使是在最近新出現(xiàn)的幾個視頻理解基準(zhǔn)上,ShareGPT4Video-8B也可以在7B參數(shù)規(guī)模上一致地展現(xiàn)出具有競爭力的性能。
表 6 :LongVideoBench上性能對比
表 7 :Video-MME基準(zhǔn)性能對比
表 8:MMBench-Video基準(zhǔn)性能對比
在視頻生成方面,研究者們基于Open-Sora-Plan項(xiàng)目簡單直接地驗(yàn)證了詳細(xì)的字幕數(shù)據(jù)對于文生視頻模型的幫助。下圖中,第一行的結(jié)果是使用了短字幕數(shù)據(jù)訓(xùn)練出的文生視頻模型得到的,第二行的結(jié)果是使用了ShareCaptioner-Video標(biāo)注的高質(zhì)量字幕數(shù)據(jù)訓(xùn)練出的文生視頻模型得到的??梢钥吹?,使用詳細(xì)的字幕數(shù)據(jù)可以讓文生視頻模型具備優(yōu)異的鏡頭移動控制以及語義內(nèi)容控制能力。
本文轉(zhuǎn)自 機(jī)器之心 ,作者:機(jī)器之心
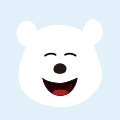