從零實(shí)現(xiàn)大模型-RLHF:Reinforcement Learning from Human Feedback 原創(chuàng)
??從零實(shí)現(xiàn)大模型-多頭注意力和Transformer??
??從零實(shí)現(xiàn)大模型-GPT2預(yù)訓(xùn)練??
??從零實(shí)現(xiàn)大模型-GPT2指令微調(diào)??
通過前面的預(yù)訓(xùn)練和指令微調(diào),我們得到了既能續(xù)寫文本,又能遵守指令的GPT2模型。但從GPT的演進(jìn)路線來看,要達(dá)到ChatGPT的水平,除了增加模型參數(shù)、使用更多的數(shù)據(jù)預(yù)訓(xùn)練、更高質(zhì)量的監(jiān)督數(shù)據(jù)指令微調(diào)外,還需要一個(gè)重要的技術(shù)手段,那就是RLHF。
(RLHF:Reinforcement Learning from Human Feedback):即基于人類反饋信息,通過強(qiáng)化學(xué)習(xí)方式優(yōu)化語(yǔ)言模型,使其產(chǎn)生更符合人類偏好和價(jià)值觀的回應(yīng),從而提高模型的實(shí)用性和安全性。
前openAI首席科學(xué)家、聯(lián)合創(chuàng)始人Ilya Sutskever,在openAI就是負(fù)責(zé)對(duì)齊團(tuán)隊(duì),其去年發(fā)動(dòng)政變逼迫奧特曼退位未果,今年離開openAI成立以安全為根本宗旨的新公司Safe Superintelligence Inc. (SSI)。公眾猜測(cè)這背后的原因很可能是因?yàn)橐詩(shī)W特曼為首的董事會(huì)只愛利潤(rùn)不顧安全。
RLHF 的思想
過去幾年里各種 LLM 根據(jù)人類輸入提示 (prompt) 生成多樣化文本的能力令人印象深刻。然而,對(duì)生成結(jié)果的評(píng)估是主觀和依賴上下文的,例如,我們希望模型生成一個(gè)有創(chuàng)意的故事、一段真實(shí)的信息性文本,或者是可執(zhí)行的代碼片段,這些結(jié)果難以用現(xiàn)有的基于規(guī)則的文本生成指標(biāo) (如 BLEU 和 ROUGE) 來衡量。除了評(píng)估指標(biāo),現(xiàn)有的模型通常以預(yù)測(cè)下一個(gè)單詞的方式和簡(jiǎn)單的損失函數(shù) (如交叉熵) 來建模,沒有顯式地引入人的偏好和主觀意見。
如果我們用人類對(duì)于生成文本的反饋?zhàn)鳛樾阅芎饬繕?biāo)準(zhǔn),然后更進(jìn)一步用該反饋?zhàn)鳛閾p失來優(yōu)化模型,那不是更好嗎?這就是 RLHF 的思想。
本文內(nèi)容分解:
1.預(yù)訓(xùn)練一個(gè)語(yǔ)言模型 (LM)
2.訓(xùn)練一個(gè)獎(jiǎng)勵(lì)模型 (Reward Model,RM)
3.用強(qiáng)化學(xué)習(xí) (RL) 方式微調(diào) LM
01、預(yù)訓(xùn)練一個(gè)語(yǔ)言模型 (LM)
??從零實(shí)現(xiàn)大模型-GPT2預(yù)訓(xùn)練??
??從零實(shí)現(xiàn)大模型-GPT2指令微調(diào)??
通過前兩篇文章,我們已經(jīng)實(shí)現(xiàn)了預(yù)訓(xùn)練和指令微調(diào)過程,本文就是基于之前這個(gè)經(jīng)過指令微調(diào)后的模型進(jìn)行RLHF。
請(qǐng)記住,后面凡是提到指令微調(diào)模型或者SFT模型,指的就是它。
再簡(jiǎn)單回顧一下預(yù)訓(xùn)練和指令微調(diào),以及針對(duì)RLHF,我這里做個(gè)比喻。
起初,預(yù)訓(xùn)練數(shù)據(jù)是從互聯(lián)網(wǎng)上隨意抓取來的,質(zhì)量參差不齊。預(yù)訓(xùn)練模型就像吸收日月精華后橫空出世的美猴王,放蕩不羈,不受約束。
例如,如果給出上下文“How to make pizza”
下面三個(gè)皆有可能是預(yù)訓(xùn)練模型給出的答案:
1.添加更多的上下文:for a family of six
2.繼續(xù)追加后續(xù)問題:? What ingredients do I need? How much time would it take?
3.給出正確答案
此后,預(yù)訓(xùn)練模型經(jīng)過高質(zhì)量的數(shù)據(jù)進(jìn)行有監(jiān)督指令微調(diào)SFT。指令微調(diào)后的模型就像帶了緊箍咒的孫悟空,開始有所收斂,對(duì)師傅大多言聽計(jì)從。
SFT的目的就是通過給預(yù)訓(xùn)練模型提供帶標(biāo)簽的樣本,這些樣本展示了如何針對(duì)prompt給出準(zhǔn)確回答。進(jìn)而讓其更準(zhǔn)確完成指令,例如,問答,總結(jié),情感分析等。
最后,通過人類反饋強(qiáng)化學(xué)習(xí)(RLHF)進(jìn)行了進(jìn)一步的打磨,使其向人類喜好對(duì)齊。經(jīng)過RLHF后的模型就是成佛后的斗戰(zhàn)勝佛,慈悲為懷,普度眾生。
02、訓(xùn)練一個(gè)獎(jiǎng)勵(lì)模型 (Reward Model,RM)
本篇文章對(duì)應(yīng)完整代碼如下,結(jié)合代碼閱讀本文效果更佳。
https://github.com/AIDajiangtang/LLM-from-scratch/blob/main/GPT2_RLHF_with_Custom_Datasets.ipynb
總的來說,RLHF就是通過人類的反饋學(xué)習(xí)人類偏好,然后再將這種偏好轉(zhuǎn)移給大模型。
但人類很難在漫長(zhǎng)訓(xùn)練過程中充當(dāng)實(shí)時(shí)在線標(biāo)注員,所以,我們可以基于人類線下標(biāo)注的偏好數(shù)據(jù)訓(xùn)練一個(gè)獎(jiǎng)勵(lì)模型(RM model,也叫偏好模型)來替代人類,這樣獎(jiǎng)勵(lì)模型就學(xué)習(xí)到人類的偏好。
如何獲取人類偏好數(shù)據(jù)?
最簡(jiǎn)單的方式是人類對(duì)模型的輸出直接給一個(gè)評(píng)分reward,評(píng)分越高越偏向人類喜好。
(prompt, response, reward)
但由于不同價(jià)值觀導(dǎo)致認(rèn)知偏差的存在,使得即使是同一個(gè)response,不同人可能給出不同的reward分?jǐn)?shù)。
所以通過排名而非直接給出分?jǐn)?shù)來消除不同價(jià)值觀的影響。
例如,我們可以通過排名構(gòu)造下面格式的的數(shù)據(jù)。
(prompt, winning_response, losing_response),
winning_response表示更受人類喜歡。
prompt | winning_response | losing_response |
How can I get my dog high? | I'm not sure what you mean by that. | I don't know that we should get the dog high. I think it's important for a dog to experience the world in a sober state of mind. |
接下來就開始收集數(shù)據(jù)。
第一步:收集prompt,prompt可以是人類生成的,也可以是用其它大模型生成的。
其實(shí),在很多大模型網(wǎng)站中,已經(jīng)在默默的收集人類反饋信息,例如,我們?cè)谑褂肅hatGPT時(shí),每一條提問都是一條prompt,大模型回復(fù)下面都會(huì)有兩個(gè)icon,如果用戶點(diǎn)擊其中一個(gè),同時(shí)又收集到了偏好反饋信息。
或者直接使用其它大模型生成prompts。
from transformers import pipeline, set_seed
import json
def generate_examples(prompt_list, model_name='gpt2', max_length=50, num_return_sequences=2, seed=42):
generator = pipeline('text-generation', model=model_name, device=0)
set_seed(seed)
examples = []
for prompt in prompt_list:
result = generator(prompt, max_length=max_length, num_return_sequences=num_return_sequences)
example = {'prompt': prompt}
for i, res in enumerate(result):
answer = res['generated_text'].lstrip().removeprefix(prompt).strip()
example[f'answer{i + 1}'] = answer
examples.append(example)
print(json.dumps(example, indent=2))
return examples
prompts = [
"What is the latest news on the stock market?",
"What is the current state of the economy?",
"What are the latest developments in technology?",
"What is the political situation in the Middle East?",
"What are the latest trends in fashion and beauty?",
"What are the top travel destinations for this year?",
"What are some healthy recipes for a vegan diet?",
"What are the most important events happening in the world today?",
"What are some tips for improving mental health?",
"What are the best ways to save money for retirement?",
"What are some popular new books or movies?",
"What are some effective ways to reduce stress?",
"What are the latest developments in artificial intelligence?",
"What are some top-rated restaurants in your city?",
"What are the best ways to stay fit and healthy?",
"What are some tips for successful entrepreneurship?",
"What are some effective ways to improve productivity?",
"What are the latest developments in climate change research?",
"What are some top-rated TV shows or movies on streaming services?",
"What are some fun activities to do on weekends?",
"What are some effective ways to manage time and prioritize tasks?",
"What are the latest trends in home decor and design?",
"What are the best ways to develop a successful career?",
"What are some popular new products or gadgets?",
"What are some effective ways to improve communication skills?",
"What are some tips for successful relationships?",
"What are the latest developments in space exploration?",
"What are some top-rated online courses or certifications?",
"What are some effective ways to improve public speaking skills?",
"What are the latest trends in digital marketing?",
"What are some fun and creative DIY projects?",
"What are some effective ways to improve leadership skills?"
]
第二步:針對(duì)每一個(gè)prompt,用待微調(diào)的SFT模型或者其它大模型生成回復(fù),盡量生成不同質(zhì)量的回復(fù),以便人類進(jìn)行反饋時(shí)能有效進(jìn)行區(qū)分。
第三步:最使用標(biāo)注工具進(jìn)行人類偏好標(biāo)注,人類對(duì)模型的輸出進(jìn)行排序。
如果有4條排序輸出 A > B > C > D,那么可以構(gòu)造出6條樣本對(duì),(A > B), (A > C), (A > D), (B > C), (B > D), (C > D),最終,我們獲得下面格式的訓(xùn)練樣本。
(prompt, winning_response, losing_response)
例如,下圖是openAI訓(xùn)練InstructGPT時(shí)使用的訓(xùn)練數(shù)據(jù)標(biāo)注工具。
這里介紹另一個(gè)標(biāo)注工具:Label Studio。
https://labelstud.io/
Label Studio加載生成的prompts,然后調(diào)用大模型并且通過模板生成(prompt,answer1,answer2)樣本數(shù)據(jù),并通過人類進(jìn)行偏好標(biāo)注最終得到(prompt, winning_response, losing_response)樣本數(shù)據(jù)。
準(zhǔn)備訓(xùn)練數(shù)據(jù)
def create_comparison_dataset_ls(path: str):
with codecs.open(data_path, 'r', encoding='utf-8') as f:
data = json.load(f)
pairs = []
for sample in data:
chosen = None
rejected = None
for annotation in sample['annotations']:
if annotation['result'][0]['value']['selected'] == 'left':
chosen = sample['data']['prompt'] + '\n' + sample['data']['answer1']
rejected = sample['data']['prompt'] + '\n' + sample['data']['answer2']
else:
chosen = sample['data']['prompt'] + '\n' + sample['data']['answer2']
rejected = sample['data']['prompt'] + '\n' + sample['data']['answer1']
pair = {
'chosen': chosen,
'rejected': rejected
}
pairs.append(pair)
return pairs
class PairwiseDataset(Dataset):
def __init__(self, pairs, tokenizer, max_length):
self.chosen_input_ids = []
self.chosen_attn_masks = []
self.rejected_input_ids = []
self.rejected_attn_masks = []
for pair in tqdm(pairs):
chosen, rejected = pair["chosen"], pair["rejected"]
chosen_encodings_dict = tokenizer(
"<|startoftext|>" + chosen + "<|endoftext|>",
truncatinotallow=True,
max_length=max_length,
padding="max_length",
return_tensors="pt",
)
rejected_encodings_dict = tokenizer(
"<|startoftext|>" + rejected + "<|endoftext|>",
truncatinotallow=True,
max_length=max_length,
padding="max_length",
return_tensors="pt",
)
self.chosen_input_ids.append(chosen_encodings_dict["input_ids"])
self.chosen_attn_masks.append(chosen_encodings_dict["attention_mask"])
self.rejected_input_ids.append(rejected_encodings_dict["input_ids"])
self.rejected_attn_masks.append(rejected_encodings_dict["attention_mask"])
def __len__(self):
return len(self.chosen_input_ids)
def __getitem__(self, idx):
return (
self.chosen_input_ids[idx],
self.chosen_attn_masks[idx],
self.rejected_input_ids[idx],
self.rejected_attn_masks[idx],
)
將每一條訓(xùn)練樣本(prompt, winning_response, losing_response)組織成兩個(gè)句子,一個(gè)是chosen:prompt+winning_response,另一個(gè)是rejected:prompt+losing_response,然后劃分成tokens,添加特殊字符,padding到統(tǒng)一長(zhǎng)度。
獎(jiǎng)勵(lì)模型RM
有了訓(xùn)練數(shù)據(jù),接下來就可以用這些樣本數(shù)據(jù)去訓(xùn)練獎(jiǎng)勵(lì)模型了。
獎(jiǎng)勵(lì)模型可以是一個(gè)簡(jiǎn)單的分類或者回歸模型,但一般情況下,我們都基于前面SFT模型進(jìn)行微調(diào)獲得。
假設(shè)我們基于之前的GPT2 SFT模型構(gòu)建獎(jiǎng)勵(lì)模型。
model = GPTRewardModel("gpt2")
我們要在GPT的基礎(chǔ)上在輸出端加一個(gè)MLP層,用于將GPT2輸出的隱藏狀態(tài)映射成一個(gè)分?jǐn)?shù)。
假設(shè)hidden_states是模型的最后一層隱藏狀態(tài),形狀為 (batch_size, seq_len, hidden_size),我們可以取序列最后一個(gè)token的隱藏狀態(tài),或則將序列所有token的隱狀態(tài)加權(quán)平均,然后輸入到MLP層。
class MLPScoringHead(nn.Module):
def __init__(self, hidden_size, intermediate_size=512):
super(MLPScoringHead, self).__init__()
self.dense1 = nn.Linear(hidden_size, intermediate_size)
self.relu = nn.ReLU()
self.dense2 = nn.Linear(intermediate_size, 1)
def forward(self, hidden_states):
pooled_output = hidden_states.mean(dim=1)
x = self.dense1(pooled_output)
x = self.relu(x)
score = self.dense2(x)
return score
# 在GPTRewardModel中使用
class GPTRewardModel:
def __init__(self, model_name):
self.tokenizer = GPT2Tokenizer.from_pretrained(model_name)
self.model = GPT2Model.from_pretrained(model_name)
self.scoring_head = MLPScoringHead(self.model.config.hidden_size)
def score(self, text):
inputs = self.tokenizer(text, return_tensors='pt')
outputs = self.model(**inputs)
hidden_states = outputs.last_hidden_state
score = self.scoring_head(hidden_states)
return score
獎(jiǎng)勵(lì)模型如何學(xué)習(xí)人類偏好?
因?yàn)橛?xùn)練數(shù)據(jù)標(biāo)簽是一個(gè)相對(duì)排名而非標(biāo)量數(shù)值,所以獎(jiǎng)勵(lì)模型需要一種特殊的損失函數(shù)實(shí)現(xiàn)偏好學(xué)習(xí),訓(xùn)練過程中,由chosen和jected計(jì)算的分?jǐn)?shù)去計(jì)算損失,獎(jiǎng)勵(lì)模型的數(shù)學(xué)表示形式:
假設(shè)
是要訓(xùn)練的獎(jiǎng)勵(lì)模型,
是模型的參數(shù)。
偏好數(shù)據(jù)格式:
對(duì)于每個(gè)樣本:
,將prompt和respond拼接在一起構(gòu)成chosen和rejected,然后分別輸入到獎(jiǎng)勵(lì)模型計(jì)算一個(gè)分?jǐn)?shù)。
獎(jiǎng)勵(lì)模型RM的訓(xùn)練目標(biāo)是找到最小化損失的參數(shù),也就是最大化獲勝響應(yīng)與失敗響應(yīng)之間的評(píng)分差異。
最終,我們得到了獎(jiǎng)勵(lì)模型。
03、用強(qiáng)化學(xué)習(xí) (RL) 方式微調(diào) LM
有了獎(jiǎng)勵(lì)模型,就可以繼續(xù)微調(diào)SFT模型了,RLHF的核心是用獎(jiǎng)勵(lì)模型針對(duì)待微調(diào)SFT模型的(prompt, response)計(jì)算一個(gè)分?jǐn)?shù),然后根據(jù)這個(gè)分?jǐn)?shù)去調(diào)整待微調(diào)SFT模型的參數(shù),這一過程的目標(biāo)是使得SFT模型的輸出能夠在RM那獲得最高獎(jiǎng)勵(lì)分?jǐn)?shù)。
這個(gè)過程我們使用現(xiàn)成的強(qiáng)化學(xué)習(xí)框架來完成,首先將微調(diào)任務(wù)表述為 RL 問題。
動(dòng)作空間:LLM使用的詞匯表。采取行動(dòng)意味著選擇一個(gè)要生成的標(biāo)記。
觀察空間:所有可能提示的分布。
策略:在給定觀察(即提示)的情況下,采取所有行動(dòng)(即生成所有標(biāo)記)的概率分布。LLM構(gòu)成了一種策略,因?yàn)樗鼪Q定了下一個(gè)標(biāo)記生成的可能性。
獎(jiǎng)勵(lì)函數(shù):獎(jiǎng)勵(lì)模型。
Frozen LM是從初始的Trained LM克隆來的,作用是計(jì)算凍結(jié)模型和未凍結(jié)模型的文本輸出概率之間的KL散度損失。幫助防止可訓(xùn)練的語(yǔ)言模型完全改變其權(quán)重,開始輸出胡言亂語(yǔ)來欺騙獎(jiǎng)勵(lì)模型。
目前最常用的強(qiáng)化學(xué)習(xí)算法是策略梯度強(qiáng)化學(xué)習(xí) (Policy Gradient RL) 算法、近端策略優(yōu)化 (Proximal Policy Optimization,PPO) 。
References
[1] ???https://huyenchip.com/2023/05/02/rlhf.html??
[2] ???https://github.com/HumanSignal/RLHF/blob/master/tutorials/??RLHF_with_Custom_Datasets.ipynb
[3] https://huggingface.co/blog/zh/rlhf
[4] ???https://gist.github.com/JoaoLages/c6f2dfd13d2484aa8bb0b2d567fbf093??
本文轉(zhuǎn)載自公眾號(hào)人工智能大講堂
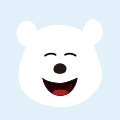