Advanced RAG 09:『提示詞壓縮』技術(shù)綜述 原創(chuàng) 精華
編者按: 如何最大限度地發(fā)揮 LLMs 的強(qiáng)大能力,同時(shí)還能控制其推理成本?這是當(dāng)前業(yè)界研究的一個(gè)熱點(diǎn)課題。
針對(duì)這一問(wèn)題,本期精心選取了一篇關(guān)于"提示詞壓縮"(Prompt Compression)技術(shù)的綜述文章。正如作者所說(shuō),提示詞壓縮技術(shù)的核心目標(biāo)是壓縮向 LLMs 輸入的上下文信息,刪減非關(guān)鍵內(nèi)容,保留語(yǔ)義核心,從而在不影響模型表現(xiàn)的前提下,降低推理成本。
文中全面介紹了多種提示詞壓縮算法的原理和實(shí)現(xiàn)細(xì)節(jié),包括基于信息熵的Selective Context、基于軟提示調(diào)優(yōu)的AutoCompressor、引入數(shù)據(jù)蒸餾方法的LLMLingua-2、綜合利用問(wèn)題語(yǔ)義的LongLLMLingua等。作者還貼心地附上了代碼示例,以便各位讀者可以動(dòng)手實(shí)踐,加深對(duì)算法的理解。
你是否曾因難以處理冗長(zhǎng)的提示詞而寢食難安,被昂貴的推理成本所困擾?現(xiàn)在,就讓我們跟隨本文的腳步,開(kāi)啟一場(chǎng) Prompt Compression 技術(shù)的學(xué)習(xí)之旅吧!也許在了解某個(gè)算法時(shí)靈感閃現(xiàn),你就能找到突破瓶頸的金鑰匙。
作者 | Florian June
編譯 | 岳揚(yáng)
RAG 方法可能會(huì)面臨兩大挑戰(zhàn):
- 大語(yǔ)言模型(LLMs)往往有上下文長(zhǎng)度(context length)的限制。這意味著,隨著輸入文本的長(zhǎng)度增長(zhǎng),處理過(guò)程不僅變得更加耗時(shí),成本也隨之增加。
- 檢索出的上下文未必都能派上用場(chǎng)。有時(shí),僅有一小部分信息對(duì)解答問(wèn)題有幫助。在某些情形下,為了回答某些特定問(wèn)題,可能需要整合來(lái)自多個(gè)文本片段的信息。即便實(shí)施了重排序(re-ranking)技術(shù),這一難題依然未能得到解決。
為了解決上述問(wèn)題,LLM 的提示詞壓縮技術(shù)(Prompt compression)應(yīng)運(yùn)而生。從本質(zhì)上講,其目的是精煉提示詞中的關(guān)鍵信息,使得每個(gè)輸入的詞元(input tokens)都承載更多價(jià)值,從而提升模型效率并還能控制成本。這一理念在圖 1 的右下角進(jìn)行了直觀展示。
圖 1:RAG 架構(gòu)中的提示詞壓縮技術(shù)(見(jiàn)圖右下角)。如紫色虛線(xiàn)標(biāo)記的部分所示,某些壓縮方法能夠直接作用于已檢索的上下文信息。此圖由作者繪制。
如圖 1 中紫色虛線(xiàn)標(biāo)記的部分所示,部分壓縮方法可以直接應(yīng)用于從大語(yǔ)言模型中檢索出的上下文信息。
總的來(lái)說(shuō),提示詞壓縮方法可以分為四大類(lèi):
- 基于信息熵(information entropy)的方法:例如 Selective Context[1]、LLMLingua[2] 和 LongLLMLingua[3]。這些方法利用小型語(yǔ)言模型來(lái)計(jì)算原始提示詞中每個(gè) token 的自信息(self-information )(譯者注:自信息,又稱(chēng)為驚喜度(surprisal)或信息含量(information content),是信息理論中的核心概念之一。它用來(lái)量化某個(gè)事件所傳達(dá)的信息量的大小。)或困惑度(perplexity)。接著刪除那些困惑度較低的 token ,實(shí)現(xiàn)壓縮目的。
- 基于 soft prompt tuning(譯者注:soft prompt tuning 不直接修改模型的權(quán)重,而是引入一組可學(xué)習(xí)的連續(xù)向量(通常稱(chēng)為"soft prompts"),這種方法允許模型在不改變其核心結(jié)構(gòu)的情況下適應(yīng)不同的下游任務(wù),同時(shí)保留了模型在預(yù)訓(xùn)練階段學(xué)到的一般知識(shí)。)的方法:如 AutoCompressor[4] 和 GIST[5]。此類(lèi)方法需要對(duì)大語(yǔ)言模型的參數(shù)進(jìn)行微調(diào),使其適用于特定領(lǐng)域,但不能直接應(yīng)用于黑盒大語(yǔ)言模型(black-box LLM)。
- 先進(jìn)行數(shù)據(jù)蒸餾,再訓(xùn)練模型生成更易解釋的文本摘要:這類(lèi)方法可以跨不同語(yǔ)言模型遷移,并能應(yīng)用于無(wú)需梯度更新的黑盒大語(yǔ)言模型。代表性的方法包括 LLMLingua-2[6] 和 RECOMP[7]。
- 基于詞元合并(token merging)或詞元剪枝(token pruning)的方法:如 ToMe[8] 和 AdapLeR[9]。這些方法通常需要在推理過(guò)程中對(duì)模型進(jìn)行微調(diào)或生成中間結(jié)果。
鑒于第四類(lèi)方法最初是為了像 ViT 或 BERT 這樣的較小模型而提出的,本文將重點(diǎn)介紹前三類(lèi)方法中代表性算法的原理。
01 Selective Context
1.1 作者的領(lǐng)悟見(jiàn)解
圖 2 表明,大語(yǔ)言模型(LLM)即使在缺乏完整上下文或?qū)υ?huà)歷史的情況下,也能對(duì)用戶(hù)的詢(xún)問(wèn)做出回應(yīng)。即便某些相關(guān)細(xì)節(jié)被省略,大語(yǔ)言模型(LLM)依舊能給出用戶(hù)期望的回答。這或許是因?yàn)榇笳Z(yǔ)言模型(LLM)能夠從上下文信息和預(yù)訓(xùn)練階段積累的知識(shí)中推斷出缺失的信息。
圖 2:即便去除了部分非關(guān)鍵信息,大語(yǔ)言模型(LLM)依然能準(zhǔn)確作答。來(lái)源:《Selective Context》[1]
由此看來(lái),我們可以通過(guò)篩選掉非關(guān)鍵信息來(lái)優(yōu)化上下文長(zhǎng)度(context length),而不會(huì)影響其整體性能。這就是 Selective Context 方法的關(guān)鍵所在。
Selective Context 策略采用小型語(yǔ)言模型(SLM),來(lái)計(jì)算給定上下文中各個(gè)詞匯單元(比如句子、短語(yǔ)或詞語(yǔ))的自信息值。然后,基于這些自信息值(self-information)進(jìn)一步評(píng)估各單元的信息含量。通過(guò)僅保留自信息值較高的內(nèi)容,Selective Context 為大語(yǔ)言模型(LLM)提供了更為簡(jiǎn)潔、高效的 context representation (譯者注:經(jīng)過(guò)數(shù)學(xué)化或模型化文本或?qū)υ?huà)后的機(jī)器可處理的上下文信息)。這一做法不會(huì)對(duì)其在各種任務(wù)中的表現(xiàn)造成負(fù)面影響。
1.2 Self-Information 自信息
Selective Context 運(yùn)用自信息(self-information)來(lái)衡量?jī)?nèi)容的價(jià)值。
自信息,又稱(chēng)為驚喜度(surprisal)或信息含量(information content),是信息理論中的核心概念之一。它用來(lái)量化某個(gè)事件所傳達(dá)的信息量的大小。具體來(lái)說(shuō),它是 token 出現(xiàn)概率的負(fù)對(duì)數(shù)形式:
這里,??(??) 代表 token ?? 的自信息量,而 ??(??) 則指代該 token 的出現(xiàn)概率。
在信息論框架(information theory)下,自信息反映了事件發(fā)生時(shí)帶來(lái)的驚喜程度或不確定性程度。那些不常見(jiàn)的事件,由于包含了更多新穎的信息,因而具有較高的自信息值。 相比之下,頻繁發(fā)生的事件,因其提供的新信息較少,自信息值也就相應(yīng)較低。
1.3 Algorithm 算法
為了便于闡述其背后的原理,我們不妨一同探究一下其源代碼。
首要步驟是配置開(kāi)發(fā)環(huán)境,安裝必需的 Python 庫(kù)以及下載 Spacy 模型。
(base) Florian:~ Florian$ conda create -n "selective_context" python=3.10
(base) Florian:~ Florian$ conda activate selective_context
(selective_context) Florian:~ Florian$ pip install selective-context
(selective_context) Florian:~ Florian$ python -m spacy download en_core_web_sm
安裝完成后,版本信息如下:
(selective_context) Florian:~ Florian$ pip list | grep selective
selective-context 0.1.4
測(cè)試代碼如下所示:
from selective_context import SelectiveContext
sc = SelectiveContext(model_type='gpt2', lang='en')
text = "INTRODUCTION Continual Learning ( CL ) , also known as Lifelong Learning , is a promising learning paradigm to design models that have to learn how to perform multiple tasks across different environments over their lifetime [To uniform the language and enhance the readability of the paper we adopt the unique term continual learning ( CL ) .]. Ideal CL models in the real world should be deal with domain shifts , researchers have recently started to sample tasks from two different datasets . For instance , proposed to train and evaluate a model on Imagenet first and then challenge its performance on the Places365 dataset . considers more scenarios , starting with Imagenet or Places365 , and then moving on to the VOC/CUB/Scenes datasets. Few works propose more advanced scenarios built on top of more than two datasets."
context, reduced_content = sc(text)
# We can also adjust the reduce ratio
# context_ratio, reduced_content_ratio = sc(text, reduce_ratio = 0.5)
初次執(zhí)行時(shí),系統(tǒng)會(huì)自動(dòng)下載 GPT-2 模型,該模型的文件大小接近 500MB。圖 3 呈現(xiàn)了測(cè)試代碼的具體運(yùn)行結(jié)果。
圖 3:Selective Context 算法測(cè)試代碼運(yùn)行結(jié)果。截圖由作者提供。
隨后,我們將深入研究 sc(text) 函數(shù)。該函數(shù)的內(nèi)部實(shí)現(xiàn)代碼[10]如下:
class SelectiveContext:
...
...
def __call__(self, text: str, reduce_ratio: float = 0.35, reduce_level :str = 'phrase') -> List[str]:
context = self.beautify_context(text)
self.mask_ratio = reduce_ratio
sents = [sent.strip() for sent in re.split(self.sent_tokenize_pattern, context) if sent.strip()]
# You want the reduce happen at sentence level, phrase level, or token level?
assert reduce_level in ['sent', 'phrase', 'token'], f"reduce_level should be one of ['sent', 'phrase', 'token'], got {reduce_level}"
sent_lus, phrase_lus, token_lus = self._lexical_unit(sents)
lexical_level = {
'sent': sent_lus,
'phrase': phrase_lus,
'token': token_lus
}
# context is the reduced context, masked_sents denotes what context has been filtered out
context, masked_sents = self.self_info_mask(lexical_level[reduce_level].text, lexical_level[reduce_level].self_info, reduce_level)
return context, masked_sents
這段代碼的核心操作分為三個(gè)階段:
- 首先,計(jì)算出上下文中每一個(gè) token 的自信息值。
- 接著,依據(jù)詞匯單位(比如短語(yǔ)或句子)整合 token 與其對(duì)應(yīng)的自信息。
- 最后,采取有選擇的方式保留必要的信息上下文,從而達(dá)到優(yōu)化的目的。
第一步:自信息的計(jì)算
給定上下文 ??=??0,??1,…,???? ,其中每個(gè) ???? 均代表一個(gè) token ,我們可以借助因果語(yǔ)言模型(例如 GPT-2、OPT 或 LLaMA)來(lái)求解每個(gè) token ???? 的自信息值:
若你選用的是 GPT-2 模型,以下便是實(shí)現(xiàn)此計(jì)算過(guò)程的相應(yīng)代碼片段[11]:
class SelectiveContext:
...
...
def _get_self_info_via_gpt2(self, text: str) -> Tuple[List[str], List[float]]:
if self.lang == 'en':
text = f"<|endoftext|>{text}"
elif self.lang == 'zh':
text = f"[CLS]{text}"
with torch.no_grad():
encoding = self.tokenizer(text, add_special_tokens=False, return_tensors='pt')
encoding = encoding.to(self.device)
outputs = self.model(**encoding)
logits = outputs.logits
probs = torch.softmax(logits, dim=-1)
self_info = -torch.log(probs)
input_ids = encoding['input_ids']
input_ids_expaned = input_ids[:, 1:].unsqueeze(-1)
第二步:整合為詞匯單元(Lexical Units)
如果僅僅在 tokens 層面上執(zhí)行 selective context filtering(譯者注:識(shí)別和保留那些對(duì)當(dāng)前任務(wù)或用戶(hù)查詢(xún)最為關(guān)鍵的信息,同時(shí)過(guò)濾掉不太相關(guān)或冗余的部分。),可能會(huì)導(dǎo)致最終的上下文失去連貫性。舉個(gè)例子,原本的數(shù)字"2009"在壓縮后可能會(huì)變成"209",這樣的結(jié)果顯然不夠合理。
鑒于此,除了在 tokens 層面進(jìn)行篩選外,同時(shí)在短語(yǔ)和句子層面上實(shí)行過(guò)濾策略也是極其重要的。在這里,我們所說(shuō)的過(guò)濾(filtering)基本單位------詞匯單元,可以是個(gè)別的 token ,也可以是完整的短語(yǔ)或是句子。
那么,怎樣才能計(jì)算出每個(gè)詞匯單元 ??=(????,…,????+??) 的自信息呢?我們可以根據(jù)自信息的可加性原則,將組成 u 的每個(gè) token 的自信息相加:
下面是具體的代碼實(shí)現(xiàn)[12],為了便于調(diào)試,我對(duì)部分變量添加了詳細(xì)的注釋?zhuān)?/p>
class SelectiveContext:
...
...
def _lexical_unit(self, sents):
if self.sent_level_self_info:
sent_self_info = []
all_noun_phrases = []
all_noun_phrases_info = []
all_tokens = []
all_token_self_info = []
for sent in sents:
# print(sent)
tokens, self_info = self.get_self_information(sent)
'''
ipdb> sent
'INTRODUCTION Continual Learning ( CL ) , also known as Lifelong Learning , is a promising learning paradigm to design models that have to learn how to perform multiple tasks across different environments over their lifetime [To uniform the language and enhance the readability of the paper we adopt the unique term continual learning ( CL ) .].'
ipdb> tokens
['IN', 'TR', 'ODUCT', 'ION', ' Contin', 'ual', ' Learning', ' (', ' CL', ' )', ',', ' also', ' known', ' as', ' Lif', 'elong', ' Learning', ',', ' is', ' a', ' promising', ' learning', ' paradigm', ' to', ' design', ' models', ' that', ' have', ' to', ' learn', ' how', ' to', ' perform', ' multiple', ' tasks', ' across', ' different', ' environments', ' over', ' their', ' lifetime', ' [', 'To', ' uniform', ' the', ' language', ' and', ' enhance', ' the', ' read', 'ability', ' of', ' the', ' paper', ' we', ' adopt', ' the', ' unique', ' term', ' continual', ' learning', ' (', ' CL', ' )', '.', '].']
ipdb> self_info
[7.514791011810303, 1.632637619972229, 0.024813441559672356, 0.006853647995740175, 12.09920597076416, 2.1144468784332275, 9.457701683044434, 2.4503376483917236, 10.236454963684082, 0.8689146041870117, 5.269547939300537, 4.641763210296631, 0.22138957679271698, 0.010370315983891487, 10.071824073791504, 0.6905602216720581, 0.01698811538517475, 1.5882389545440674, 0.4495090842247009, 0.45371606945991516, 6.932497978210449, 6.087430477142334, 3.66465425491333, 3.3969509601593018, 7.337691307067871, 5.881226539611816, 1.7340556383132935, 4.599822521209717, 6.482723236083984, 4.045308589935303, 4.762691497802734, 0.21346867084503174, 3.7985599040985107, 4.6389899253845215, 0.33642446994781494, 4.918881416320801, 2.076707601547241, 3.3553669452667236, 5.5081071853637695, 5.625778675079346, 0.7966060638427734, 6.347291946411133, 12.772034645080566, 13.792041778564453, 4.11267614364624, 6.583715915679932, 3.3618998527526855, 8.434362411499023, 1.2423189878463745, 5.8330583572387695, 0.0013973338063806295, 0.3090735077857971, 1.1139129400253296, 4.160390853881836, 3.744772434234619, 7.2841596603393555, 1.4088190793991089, 7.86871337890625, 4.305004596710205, 9.69282341003418, 0.08665203303098679, 1.6127821207046509, 1.6296097040176392, 0.46206924319267273, 3.0398476123809814, 6.892032623291016]
'''
sent_self_info.append(np.mean(self_info))
all_tokens.extend(tokens)
all_token_self_info.extend(self_info)
noun_phrases, noun_phrases_info = self._calculate_lexical_unit(tokens, self_info)
'''
ipdb> noun_phrases
['INTRODUCTION Continual Learning', ' (', ' CL', ' )', ',', ' also', ' known', ' as', ' Lifelong Learning', ',', ' is', ' a promising learning paradigm', ' to', ' design', ' models', ' that', ' have', ' to', ' learn', ' how', ' to', ' perform', ' multiple tasks', ' across', ' different environments', ' over', ' their lifetime', ' [', 'To', ' uniform', ' the language', ' and', ' enhance', ' the readability', ' of', ' the paper', ' we', ' adopt', ' the unique term continual learning', ' (', ' CL', ' )', '.', ']', '.']
ipdb> noun_phrases_info
[4.692921464797109, 2.4503376483917236, 10.236454963684082, 0.8689146041870117, 5.269547939300537, 4.641763210296631, 0.22138957679271698, 0.010370315983891487, 3.5931241369495788, 1.5882389545440674, 0.4495090842247009, 4.284574694931507, 3.3969509601593018, 7.337691307067871, 5.881226539611816, 1.7340556383132935, 4.599822521209717, 6.482723236083984, 4.045308589935303, 4.762691497802734, 0.21346867084503174, 3.7985599040985107, 2.487707197666168, 4.918881416320801, 2.7160372734069824, 5.5081071853637695, 3.2111923694610596, 6.347291946411133, 12.772034645080566, 13.792041778564453, 5.348196029663086, 3.3618998527526855, 8.434362411499023, 2.3589248929638416, 0.3090735077857971, 2.6371518969535828, 3.744772434234619, 7.2841596603393555, 4.672402499616146, 1.6127821207046509, 1.6296097040176392, 0.46206924319267273, 3.0398476123809814, 3.446016311645508, 3.446016311645508]
'''
# We need to add a space before the first noun phrase for every sentence except the first one
if all_noun_phrases:
noun_phrases[0] = f" {noun_phrases[0]}"
all_noun_phrases.extend(noun_phrases)
all_noun_phrases_info.extend(noun_phrases_info)
return [
LexicalUnits('sent', text=sents, self_info=sent_self_info),
LexicalUnits('phrase', text=all_noun_phrases, self_info=all_noun_phrases_info),
LexicalUnits('token', text=all_tokens, self_info=all_token_self_info)
]
第三步:精選保留信息含量高的上下文
在計(jì)算了每個(gè)詞匯單元的自信息之后,我們面臨的問(wèn)題是如何判斷其信息含量。論文介紹了一種創(chuàng)新方法,利用基于百分位數(shù)的篩選策略,動(dòng)態(tài)挑選出信息最豐富的內(nèi)容。這種方法相較于設(shè)定固定閾值或僅僅保留前 k 個(gè)最高信息量的詞匯單元更為靈活有效。
我們的操作流程是先按自信息值(self-information values)從高到低排序所有詞匯單元 ,接著計(jì)算所有詞匯單元自信息值的 p-th percentile (譯者注:“p-th percentile” 在統(tǒng)計(jì)學(xué)中指的是數(shù)據(jù)分布的一個(gè)特定點(diǎn),在這一點(diǎn)之下包含了總數(shù)據(jù)中 p% 的數(shù)值。舉個(gè)例子,假設(shè)你有一個(gè)班級(jí)的數(shù)學(xué)成績(jī)分布,如果某個(gè)學(xué)生的成績(jī)位于第90百分位(90th percentile),這意味著班上90%的學(xué)生的成績(jī)低于或等于他的成績(jī),而他僅比剩下的10%的學(xué)生成績(jī)低。)。最后,我們精挑細(xì)選出那些自信息值不低于該百分位數(shù)的詞匯單元,確保所保留的都是信息含量最高的部分。
相關(guān)代碼[13]如下:
class SelectiveContext:
...
...
def self_info_mask(self, sents: List[str], self_info: List[float], mask_level):
# mask_level: mask sentences, phrases, or tokens
sents_after_mask = []
masked_sents = []
self.ppl_threshold = np.nanpercentile(self_info, self.mask_ratio * 100)
# if title is not None:
# with open(os.path.join(self.path, title+'_prob_token.tsv'), 'w', encoding='utf-8') as f:
# for token, info in zip(tokens, self_info):
# f.write(f"{token}\t{info}\n")
# with open(os.path.join(self.path, title+'_prob_sent.tsv'), 'w', encoding='utf-8') as f:
# for sent, info in zip(sents, sent_self_info):
# f.write(f"{sent}\n{info}\n\n")
for sent, info in zip(sents, self_info):
if info < self.ppl_threshold:
masked_sents.append(sent)
sents_after_mask.append(self.mask_a_sent(sent, mask_level))
else:
sents_after_mask.append(sent)
masked_context = " ".join(sents_after_mask) if mask_level == 'sent' else "".join(sents_after_mask)
return masked_context, masked_sents
02 LLMLingua
2.1 Overview 概覽
LLMLingua[2] 這種方法認(rèn)為,Selective Context[1] 方法常常忽略了壓縮內(nèi)容間的內(nèi)在聯(lián)系及 LLM 與用于提示詞壓縮的小型語(yǔ)言模型間的協(xié)同作用。LLMLingua 正好解決了這些問(wèn)題。
具體而言,參照?qǐng)D 4,LLMLingua 利用 budget controller 為原始提示詞的各個(gè)組成部分(如指導(dǎo)性提示詞、演示樣例和問(wèn)題)動(dòng)態(tài)分配不同的壓縮率。同時(shí),它采取粗粒度的 demonstration-level (譯者注:在完整的演示案例上進(jìn)行壓縮或處理,而不是單獨(dú)處理每個(gè)小的組成部分(比如單詞或短語(yǔ))。)壓縮策略,確保即使在高度壓縮的情況下,語(yǔ)義依然完整無(wú)損。此外,LLMLingua[2] 還引入了一種基于 tokens 的迭代算法,進(jìn)一步優(yōu)化細(xì)粒度的提示詞壓縮過(guò)程。
圖 4:LLMLingua 方法的架構(gòu)概覽。來(lái)源:LLMLingua[2]
與 Selective Context 相比,LLMLingua 能更有效地保留提示詞中的關(guān)鍵信息,同時(shí)還能夠考慮到 tokens 之間的條件依賴(lài)關(guān)系,其壓縮倍數(shù)可達(dá) 20 倍。
2.2 Budget controller
Budget controller 是 LLMLingua 的關(guān)鍵組件,用于為原始提示詞的不同部分動(dòng)態(tài)分配不同的壓縮率。
考慮到提示詞各部分對(duì)壓縮行為的敏感程度各不相同 ------ 例如,問(wèn)題需要保持較高的信息密度,而演示樣例部分則可適度壓縮。budget controller 的職責(zé)就在于此:對(duì)指導(dǎo)性提示詞和問(wèn)題采用較低的壓縮比率,確保核心信息的完整留存;而對(duì)于演示樣例部分,則可實(shí)施更高比率的壓縮,剔除不必要的冗余信息。
budget controller 的具體算法,詳述于圖 5 中。
圖 5:budget controller 的具體算法。Source: LLMLingua[2]
其核心變量定義如下:
- M?: 小型語(yǔ)言模型,比如 GPT-2 或 LLaMA。
- x = (x^ins , x^dems , x^que): 原始提示詞,整合了指導(dǎo)性提示詞、演示樣例與問(wèn)題三大部分。
- L, L_ins, L_dems, 和 L_que分別代表 x, x^ins , x^dems, 和 x^que 中的 token 總數(shù)。
- ??_dems: 在總體壓縮率 τ 的約束下,依據(jù)指導(dǎo)性提示詞和問(wèn)題預(yù)設(shè)的壓縮率 τ_ins 和 τ_que 來(lái)決定的演示樣例壓縮率。
- D: 集合 D 將收納所有經(jīng)過(guò)壓縮處理后的演示樣例。
主要操作步驟如下:
- 確定演示樣例的壓縮比例。
- 利用小型語(yǔ)言模型(如 GPT-2 或 LLaMA)計(jì)算原始演示樣例集合中每個(gè)演示樣例的困惑度(perplexity)。
- 按照困惑度從高到低排序全部演示樣例。
- 迭代挑選演示樣例并將其添加到集合 D。
- 完成演示樣例的壓縮后,將未使用的 budget (譯者注:在總體壓縮率的限制下,算法會(huì)優(yōu)先確保演示樣例被充分壓縮,然后利用剩下的壓縮能力去進(jìn)一步壓縮指導(dǎo)性提示詞和問(wèn)題,以實(shí)現(xiàn)最佳的信息保留和資源利用平衡。)轉(zhuǎn)用于指導(dǎo)性提示詞和問(wèn)題的處理。
- 輸出經(jīng)過(guò)粗粒度壓縮后的集合 D。
借助 demonstration-level (譯者注:在完整的演示案例上進(jìn)行壓縮或處理,而不是單獨(dú)處理每個(gè)小的組成部分(比如單詞或短語(yǔ))。)的壓縮流程,budget controller 可以確保在削減數(shù)據(jù)量的同時(shí),核心信息得以保全,能夠有效實(shí)現(xiàn)原始提示詞的瘦身。這一策略特別適合處理包含多重演示樣例的復(fù)雜提示詞。
涉及的程序代碼,可在 control_context_budget 函數(shù)[14]中找到實(shí)現(xiàn)細(xì)節(jié)。
2.3 Iterative Token-level Prompt Compression (ITPC)
利用困惑度(perplexity)作為壓縮標(biāo)準(zhǔn),有其內(nèi)在的局限性:the independence assumption(譯者注:假設(shè)文本序列中的每個(gè)詞匯(token)或字符的出現(xiàn)是彼此獨(dú)立的,其出現(xiàn)的概率只依賴(lài)于它前面的一個(gè)或幾個(gè)詞匯,而與序列中更遠(yuǎn)的其他詞匯無(wú)關(guān)。)。該假設(shè)認(rèn)為每個(gè) token 在提示詞中孤立存在,其出現(xiàn)的概率僅取決于緊鄰的前一個(gè)token,而不受其他任何 tokens 的影響。
然而,這一假設(shè)忽略了自然語(yǔ)言中詞元(token)間錯(cuò)綜復(fù)雜的相互依存關(guān)系,而這種關(guān)系對(duì)于理解上下文和保持語(yǔ)義的完整性至關(guān)重要。
忽略這些相互依存的關(guān)系,極有可能在壓縮過(guò)程中造成重要信息的流失。 例如,在進(jìn)行高比例壓縮時(shí),倘若某個(gè) token 承載著上下文中的核心推理環(huán)節(jié)或邏輯聯(lián)系紐帶,那么僅僅依據(jù)其困惑度判定其去留,可能會(huì)導(dǎo)致推理鏈的斷裂。
為克服這一挑戰(zhàn),LLMLingua 引入了 Iterative Token-level Prompt Compression(ITPC) 算法。不同于僅憑獨(dú)立概率評(píng)判 token 價(jià)值的傳統(tǒng)方法,ITPC 算法在壓縮提示詞時(shí),會(huì)更精細(xì)地評(píng)估每個(gè) token 的實(shí)際貢獻(xiàn)。通過(guò)反復(fù)審視提示詞的每一部分,同時(shí)考量當(dāng)前上下文中每個(gè) token 的條件概率,這一算法能更有效地維系 token 間的內(nèi)在聯(lián)系,確保壓縮后提示詞的語(yǔ)義完整性和邏輯連貫性。
圖 6 展示了 ITPC 算法的詳細(xì)步驟:
圖 6:ITPC 算法的詳細(xì)步驟。圖片由原文作者提供
借助這一流程,ITPC 算法可以有效縮短提示詞信息的長(zhǎng)度,同時(shí)還能確保其語(yǔ)義內(nèi)容的完整性,進(jìn)而有效地降低了 LLM 的推理成本。
相關(guān)的實(shí)現(xiàn)代碼可以在函數(shù) ??iterative_compress_prompt?
?[15] 中找到。
2.4 Instruction Tuning 指令調(diào)優(yōu)
如圖 4 所示,在 LLMLingua 框架內(nèi),指令調(diào)優(yōu)(instruction tuning)扮演著至關(guān)重要的角色。該步驟的核心目的是縮小用于提示詞壓縮的小型語(yǔ)言模型與大語(yǔ)言模型(LLMs)之間在分布特性上的差異。
圖 7 展示了 Instruction Tuning 算法的詳細(xì)步驟:
圖 7:Instruction Tuning 的詳細(xì)步驟。圖片由原文作者提供
2.5 Code Demonstration 代碼演示
我們現(xiàn)在開(kāi)始展示代碼。首要步驟是配置好環(huán)境。
(base) Florian:~ Florian$ conda create -n "llmlingua" python=3.11
(base) Florian:~ Florian$ conda activate llmlingua
(llmlingua) Florian:~ Florian$ pip install llmlingua
以下是已安裝的版本信息:
llmlingua 0.2.1
下面是用于測(cè)試的代碼段:
from llmlingua import PromptCompressor
GSM8K_PROMPT = "Question: Angelo and Melanie want to plan how many hours over the next week they should study together for their test next week. They have 2 chapters of their textbook to study and 4 worksheets to memorize. They figure out that they should dedicate 3 hours to each chapter of their textbook and 1.5 hours for each worksheet. If they plan to study no more than 4 hours each day, how many days should they plan to study total over the next week if they take a 10-minute break every hour, include 3 10-minute snack breaks each day, and 30 minutes for lunch each day?\nLet's think step by step\nAngelo and Melanie think they should dedicate 3 hours to each of the 2 chapters, 3 hours x 2 chapters = 6 hours total.\nFor the worksheets they plan to dedicate 1.5 hours for each worksheet, 1.5 hours x 4 worksheets = 6 hours total.\nAngelo and Melanie need to start with planning 12 hours to study, at 4 hours a day, 12 / 4 = 3 days.\nHowever, they need to include time for breaks and lunch. Every hour they want to include a 10-minute break, so 12 total hours x 10 minutes = 120 extra minutes for breaks.\nThey also want to include 3 10-minute snack breaks, 3 x 10 minutes = 30 minutes.\nAnd they want to include 30 minutes for lunch each day, so 120 minutes for breaks + 30 minutes for snack breaks + 30 minutes for lunch = 180 minutes, or 180 / 60 minutes per hour = 3 extra hours.\nSo Angelo and Melanie want to plan 12 hours to study + 3 hours of breaks = 15 hours total.\nThey want to study no more than 4 hours each day, 15 hours / 4 hours each day = 3.75\nThey will need to plan to study 4 days to allow for all the time they need.\nThe answer is 4\n\nQuestion: You can buy 4 apples or 1 watermelon for the same price. You bought 36 fruits evenly split between oranges, apples and watermelons, and the price of 1 orange is $0.50. How much does 1 apple cost if your total bill was $66?\nLet's think step by step\nIf 36 fruits were evenly split between 3 types of fruits, then I bought 36/3 = 12 units of each fruit\nIf 1 orange costs $0.50 then 12 oranges will cost $0.50 * 12 = $6\nIf my total bill was $66 and I spent $6 on oranges then I spent $66 - $6 = $60 on the other 2 fruit types.\nAssuming the price of watermelon is W, and knowing that you can buy 4 apples for the same price and that the price of one apple is A, then 1W=4A\nIf we know we bought 12 watermelons and 12 apples for $60, then we know that $60 = 12W + 12A\nKnowing that 1W=4A, then we can convert the above to $60 = 12(4A) + 12A\n$60 = 48A + 12A\n$60 = 60A\nThen we know the price of one apple (A) is $60/60= $1\nThe answer is 1\n\nQuestion: Susy goes to a large school with 800 students, while Sarah goes to a smaller school with only 300 students. At the start of the school year, Susy had 100 social media followers. She gained 40 new followers in the first week of the school year, half that in the second week, and half of that in the third week. Sarah only had 50 social media followers at the start of the year, but she gained 90 new followers the first week, a third of that in the second week, and a third of that in the third week. After three weeks, how many social media followers did the girl with the most total followers have?\nLet's think step by step\nAfter one week, Susy has 100+40 = 140 followers.\nIn the second week, Susy gains 40/2 = 20 new followers.\nIn the third week, Susy gains 20/2 = 10 new followers.\nIn total, Susy finishes the three weeks with 140+20+10 = 170 total followers.\nAfter one week, Sarah has 50+90 = 140 followers.\nAfter the second week, Sarah gains 90/3 = 30 followers.\nAfter the third week, Sarah gains 30/3 = 10 followers.\nSo, Sarah finishes the three weeks with 140+30+10 = 180 total followers.\nThus, Sarah is the girl with the most total followers with a total of 180.\nThe answer is 180"
llm_lingua = PromptCompressor()
## Or use the phi-2 model,
# llm_lingua = PromptCompressor("microsoft/phi-2")
## Or use the quantation model, like TheBloke/Llama-2-7b-Chat-GPTQ, only need <8GB GPU memory.
## Before that, you need to pip install optimum auto-gptq
# llm_lingua = PromptCompressor("TheBloke/Llama-2-7b-Chat-GPTQ", model_config={"revision": "main"})
compressed_prompt = llm_lingua.compress_prompt(GSM8K_PROMPT.split("\n\n")[0], instruction="", question="", target_token=200)
print('-' * 100)
print("original:")
print(GSM8K_PROMPT.split("\n\n")[0])
print('-' * 100)
print("compressed_prompt:")
print(compressed_prompt)
首次運(yùn)行時(shí)會(huì)自動(dòng)下載默認(rèn)模型。當(dāng)然,我們也有另一個(gè)選項(xiàng),即使用量化模型(quantized model)。相關(guān)的運(yùn)行結(jié)果展示在圖 8 中:
圖 8 :LLMLingua 測(cè)試代碼的運(yùn)行結(jié)果。此截圖由原文作者提供
03 LongLLMLingua
LLMLingua 的問(wèn)題在于,在壓縮處理過(guò)程中忽略了用戶(hù)提出的問(wèn)題,這可能導(dǎo)致一些無(wú)關(guān)緊要的信息被無(wú)謂地保留下來(lái)。
而 LongLLMLingua[3] 的設(shè)計(jì)初衷正是為了解決這一缺陷,它創(chuàng)新性地在壓縮流程中融入了對(duì)用戶(hù)問(wèn)題的考量和處理。
圖 9:LongLLMLingua 框架,灰色斜體內(nèi)容與 LLMLingua 相同。圖片來(lái)源:LongLLMLingua[3]
如圖 9 所示,LongLLMLingua 框架引入了四項(xiàng)新功能,以提升大語(yǔ)言模型識(shí)別關(guān)鍵信息的能力:
- 針對(duì)用戶(hù)問(wèn)題的粗粒度和細(xì)粒度兩級(jí)壓縮技術(shù)(Question-aware coarse-grained and fine-grained compression);
- 動(dòng)態(tài)調(diào)整的文檔排序機(jī)制(Document reordering mechanism);
- 可變的壓縮比例設(shè)定(Dynamic compression ratio);
- 子序列恢復(fù)算法(Subsequence recovery algorithm)。
3.1 針對(duì)用戶(hù)問(wèn)題的粗粒度壓縮技術(shù)
LongLLMLingua 推薦采用這樣一種方法,利用在不同文檔上下文 ??x^doc_k?
?? 背景下問(wèn)題 ??x^que?
?? 的困惑度,來(lái)衡量?jī)烧唛g的關(guān)聯(lián)強(qiáng)度。我們可以在問(wèn)題 ??x^que?
?? 后面附加一句限定語(yǔ) ??x^restrict = "我們可以在提供的文檔里找到這個(gè)問(wèn)題的答案"?
??。這樣做的目的是強(qiáng)化 ??x^que?
?? 與 ??x^doc_k?
? 之間的聯(lián)系,同時(shí),這句話(huà)作為一個(gè)正則化項(xiàng)(regularization item),能有效降低模型產(chǎn)生不切實(shí)際的預(yù)測(cè)結(jié)果的可能性。這可以表示為:
為什么不直接計(jì)算在問(wèn)題 x^que 約束下的整體文檔困惑度呢?原因在于,文檔內(nèi)往往充斥著許多與問(wèn)題不相關(guān)的冗余信息。即便是在 x^que 的引導(dǎo)下,對(duì)整篇文檔計(jì)算出的困惑度值也可能不夠明顯,從而導(dǎo)致它無(wú)法成為評(píng)估文檔層面壓縮效果的理想指標(biāo)。
可以在函數(shù) get_distance_longllmlingua[16] 中找到實(shí)現(xiàn)這一技術(shù)的相關(guān)代碼。
3.2 針對(duì)用戶(hù)問(wèn)題的細(xì)粒度壓縮技術(shù)
LongLLMLingua 引入了對(duì)比困惑度(contrastive perplexity)的概念。
首先,我們計(jì)算一個(gè) token 的困惑度,不考慮問(wèn)題的情況下,表示為 ??perplexity(x_i | x<i)?
?? 。然后,我們?cè)俅螠y(cè)量困惑度,這次包括了問(wèn)題,表示為 ??perplexity(x_i | x^que, x<i)?
??。這衡量的是在給定問(wèn)題 ??x^que?
?? 的情況下,詞元 ??x_i?
? 對(duì)之前所有詞元(token)的驚訝程度。
我們的目標(biāo)是確定每個(gè) token 的驚訝程度隨問(wèn)題變化的程度。如果當(dāng)問(wèn)題被包括進(jìn)來(lái)后,某個(gè)詞變得不那么令人驚訝,那么這個(gè)詞很可能與問(wèn)題高度相關(guān)。
3.3 動(dòng)態(tài)調(diào)整的文檔排序機(jī)制
如圖 10 所示,在推理階段,大語(yǔ)言模型(LLMs)傾向于利用提示詞信息的起始和尾部?jī)?nèi)容,而往往忽視了其中間部分的信息,這便是所謂的??"Lost in the Middle"?
?問(wèn)題。
圖 10:大語(yǔ)言模型(LLM)對(duì)相關(guān)資訊的把握能力受到其在提示詞信息中位置的影響。為了解決中間信息丟失的問(wèn)題,我們引入了一項(xiàng)文檔重排序機(jī)制。圖片來(lái)源:LongLLMLingua[3]
圖 10 進(jìn)一步表明,當(dāng)關(guān)鍵信息被置于開(kāi)頭時(shí),LLMs 的表現(xiàn)最為出色?;诖?, LongLLMLingua 會(huì)根據(jù)粗粒度壓縮的結(jié)果來(lái)組織段落,從前往后依據(jù)評(píng)分高低進(jìn)行排序。
3.4 可變的壓縮比例設(shè)定
鑒于不同文檔中關(guān)鍵信息的密集程度存在差異,我們應(yīng)當(dāng)對(duì)那些與問(wèn)題更加相關(guān)的文檔分配更多資源(即采取更低的壓縮比率)。
LongLLMLingua 運(yùn)用在粗粒度壓縮過(guò)程中得出的重要性得分,來(lái)指引細(xì)粒度壓縮階段的資源分配策略。
具體操作如下:首先,通過(guò) LLMLingua 的 budget controller 為保留的文檔設(shè)定初始資源量。隨后,在細(xì)粒度壓縮階段,為每個(gè)文檔動(dòng)態(tài)分配資源。這一分配策略基于文檔在粗粒度壓縮階段確定的重要性得分排名,以排名順序作為資源分配依據(jù)。
LongLLMLingua 實(shí)施了一種線(xiàn)性調(diào)度方法(linear scheduler),實(shí)現(xiàn)資源的自適應(yīng)分配(adaptive allocation)。對(duì)于每個(gè)詞元(token) ??xi?
?,其資源量計(jì)算公式如下:
其中,??Nd?
??表示所有文檔的數(shù)量,???????
?是一個(gè)控制動(dòng)態(tài)分配總資源量的超參數(shù)。
對(duì)應(yīng)的源代碼可以在 get_dynamic_compression_ratio[17] 函數(shù)中找到。
3.5 子序列恢復(fù)算法
如圖 11 所示,在細(xì)粒度的逐 token 壓縮環(huán)節(jié)中,一些關(guān)鍵實(shí)體的 token 有被丟棄的風(fēng)險(xiǎn)。例如,??"2009"?
??在原始提示詞中可能被壓縮至??"209"?
??,??"Wilhelm Conrad Rontgen"?
??也可能被簡(jiǎn)化壓縮為??"Wilhelmgen"?
?。
圖 11:展示了一個(gè)子序列恢復(fù)算法案例,其中紅色文本代表原始內(nèi)容,而藍(lán)色文字則是經(jīng)過(guò)壓縮后的結(jié)果。來(lái)源:LongLLMLingua[3]
LongLLMLingua 設(shè)計(jì)了一套子序列恢復(fù)算法,能夠從大語(yǔ)言模型(LLMs)的回應(yīng)中復(fù)原原始信息,如圖 12 所示。
圖 12:子序列恢復(fù)算法流程圖。圖片來(lái)源:LongLLMLingua
其核心流程包括以下幾個(gè)步驟:
- 遍歷大語(yǔ)言模型(LLM)響應(yīng)內(nèi)容中的每一個(gè)詞元(token)?
?yl?
??,從中選取在壓縮提示詞 ??x??
?? 中出現(xiàn)的最長(zhǎng)子序列 ??y?key,l?
?; - 在原始提示詞 ?
?x?
?? 內(nèi),尋找與 ??y?key,l?
?? 匹配的最大公共最短子序列(maximum common shortest subsequence)??xi,j?
?; - 將大語(yǔ)言模型(LLMs)響應(yīng)內(nèi)容中的相應(yīng)詞元 ?
?y?key,l?
?? 替換為原始的 ??xi,j?
?。
這一算法的具體代碼可以在 recover 函數(shù)[18]中找到。
3.6 代碼演示
環(huán)境配置的方法與 LLMLingua 相同。下面是測(cè)試代碼:
from llmlingua import PromptCompressor
GSM8K_PROMPT = "Question: Angelo and Melanie want to plan how many hours over the next week they should study together for their test next week. They have 2 chapters of their textbook to study and 4 worksheets to memorize. They figure out that they should dedicate 3 hours to each chapter of their textbook and 1.5 hours for each worksheet. If they plan to study no more than 4 hours each day, how many days should they plan to study total over the next week if they take a 10-minute break every hour, include 3 10-minute snack breaks each day, and 30 minutes for lunch each day?\nLet's think step by step\nAngelo and Melanie think they should dedicate 3 hours to each of the 2 chapters, 3 hours x 2 chapters = 6 hours total.\nFor the worksheets they plan to dedicate 1.5 hours for each worksheet, 1.5 hours x 4 worksheets = 6 hours total.\nAngelo and Melanie need to start with planning 12 hours to study, at 4 hours a day, 12 / 4 = 3 days.\nHowever, they need to include time for breaks and lunch. Every hour they want to include a 10-minute break, so 12 total hours x 10 minutes = 120 extra minutes for breaks.\nThey also want to include 3 10-minute snack breaks, 3 x 10 minutes = 30 minutes.\nAnd they want to include 30 minutes for lunch each day, so 120 minutes for breaks + 30 minutes for snack breaks + 30 minutes for lunch = 180 minutes, or 180 / 60 minutes per hour = 3 extra hours.\nSo Angelo and Melanie want to plan 12 hours to study + 3 hours of breaks = 15 hours total.\nThey want to study no more than 4 hours each day, 15 hours / 4 hours each day = 3.75\nThey will need to plan to study 4 days to allow for all the time they need.\nThe answer is 4\n\nQuestion: You can buy 4 apples or 1 watermelon for the same price. You bought 36 fruits evenly split between oranges, apples and watermelons, and the price of 1 orange is $0.50. How much does 1 apple cost if your total bill was $66?\nLet's think step by step\nIf 36 fruits were evenly split between 3 types of fruits, then I bought 36/3 = 12 units of each fruit\nIf 1 orange costs $0.50 then 12 oranges will cost $0.50 * 12 = $6\nIf my total bill was $66 and I spent $6 on oranges then I spent $66 - $6 = $60 on the other 2 fruit types.\nAssuming the price of watermelon is W, and knowing that you can buy 4 apples for the same price and that the price of one apple is A, then 1W=4A\nIf we know we bought 12 watermelons and 12 apples for $60, then we know that $60 = 12W + 12A\nKnowing that 1W=4A, then we can convert the above to $60 = 12(4A) + 12A\n$60 = 48A + 12A\n$60 = 60A\nThen we know the price of one apple (A) is $60/60= $1\nThe answer is 1\n\nQuestion: Susy goes to a large school with 800 students, while Sarah goes to a smaller school with only 300 students. At the start of the school year, Susy had 100 social media followers. She gained 40 new followers in the first week of the school year, half that in the second week, and half of that in the third week. Sarah only had 50 social media followers at the start of the year, but she gained 90 new followers the first week, a third of that in the second week, and a third of that in the third week. After three weeks, how many social media followers did the girl with the most total followers have?\nLet's think step by step\nAfter one week, Susy has 100+40 = 140 followers.\nIn the second week, Susy gains 40/2 = 20 new followers.\nIn the third week, Susy gains 20/2 = 10 new followers.\nIn total, Susy finishes the three weeks with 140+20+10 = 170 total followers.\nAfter one week, Sarah has 50+90 = 140 followers.\nAfter the second week, Sarah gains 90/3 = 30 followers.\nAfter the third week, Sarah gains 30/3 = 10 followers.\nSo, Sarah finishes the three weeks with 140+30+10 = 180 total followers.\nThus, Sarah is the girl with the most total followers with a total of 180.\nThe answer is 180"
QUESTION = "Question: Josh decides to try flipping a house. He buys a house for $80,000 and then puts in $50,000 in repairs. This increased the value of the house by 150%. How much profit did he make?"
llm_lingua = PromptCompressor()
compressed_prompt = llm_lingua.compress_prompt(
GSM8K_PROMPT.split("\n\n")[0],
question = QUESTION,
# ratio=0.55
# Set the special parameter for LongLLMLingua
condition_in_question = "after_condition",
reorder_context = "sort",
dynamic_context_compression_ratio = 0.3, # or 0.4
condition_compare = True,
context_budget = "+100",
rank_method = "longllmlingua",
)
print('-' * 100)
print("original:")
print(GSM8K_PROMPT.split("\n\n")[0])
print('-' * 100)
print("compressed_prompt:")
print(compressed_prompt)
運(yùn)行結(jié)果如圖 13 所示:
圖 13:LongLLMLingua 測(cè)試代碼的運(yùn)行結(jié)果。截圖由作者提供。
04 AutoCompressor
不同于先前提及的方法,AutoCompressor[4] 采取了一種基于軟提示詞的創(chuàng)新途徑。
它巧妙地通過(guò)增加詞匯量和利用??"summary tokens"?
??和??"summary vectors"?
?來(lái)提煉大量上下文信息,進(jìn)而精調(diào)現(xiàn)有的模型結(jié)構(gòu)。
圖 14:AutoCompressor 通過(guò)遞歸生成 summary vectors 來(lái)處理長(zhǎng)文檔,這些 summary vectors 作為軟提示詞(soft prompts)被傳遞給后續(xù)的所有文檔片段。圖片來(lái)源:AutoCompressor[4]
圖 14 描繪了 AutoCompressor 的工作原理,其運(yùn)行步驟如下:
- 詞匯擴(kuò)展(Expand Vocabulary):在這一步驟中,我們將 “summary tokens” 加入到模型現(xiàn)有的詞匯庫(kù)中。這些 tokens 的作用是幫助模型將龐大的信息量壓縮成更緊湊的向量表征。
- 文檔分割(Split Document):待處理的文檔被切割成若干小段,每一小段后都會(huì)附加有 summary tokens 。這些 tokens 不僅攜帶了本段的信息,還包含了前面所有段落的摘要信息,實(shí)現(xiàn)了摘要信息的連續(xù)積累(summary accumulation)。
- 微調(diào)訓(xùn)練(Fine-tuning Training):采用無(wú)監(jiān)督訓(xùn)練的方式,借助 “next word prediction” 任務(wù)對(duì)模型進(jìn)行微調(diào)。該任務(wù)的核心在于,根據(jù)當(dāng)前片段前的 tokens 序列以及之前片段的摘要向量(summary vectors),預(yù)測(cè)下一個(gè)單詞。
- 反向傳播(Backpropagation):AutoCompressor 在每個(gè)文檔片段上運(yùn)用 backpropagation through time(BPTT)(譯者注:對(duì)于每一個(gè)時(shí)間步,BPTT 都會(huì)計(jì)算損失函數(shù)關(guān)于當(dāng)前時(shí)間步和所有之前時(shí)間步參數(shù)的梯度,然后將這些梯度反向傳播回網(wǎng)絡(luò),以更新參數(shù)。) 和 gradient checkpointing(譯者注:在標(biāo)準(zhǔn)的反向傳播過(guò)程中,為了計(jì)算梯度,需要保存前向傳播過(guò)程中的所有中間結(jié)果。但隨著網(wǎng)絡(luò)深度的增加,這會(huì)消耗大量的內(nèi)存。Gradient checkpointing 通過(guò)犧牲一些計(jì)算效率來(lái)減少內(nèi)存需求。) 技術(shù),能夠有效縮減計(jì)算圖(computational graph)的規(guī)模。反向傳播針對(duì)整個(gè)文檔進(jìn)行,使得模型能夠全面理解并學(xué)習(xí)到整個(gè)上下文之間存在的關(guān)聯(lián)。
4.1 代碼演示
AutoCompressor[19] 開(kāi)放了其源代碼,感興趣的讀者可以試著讀一讀。
import torch
from transformers import AutoTokenizer
from auto_compressor import LlamaAutoCompressorModel, AutoCompressorModel
# Load AutoCompressor trained by compressing 6k tokens in 4 compression steps
tokenizer = AutoTokenizer.from_pretrained("princeton-nlp/AutoCompressor-Llama-2-7b-6k")
# Need bfloat16 + cuda to run Llama model with flash attention
model = LlamaAutoCompressorModel.from_pretrained("princeton-nlp/AutoCompressor-Llama-2-7b-6k", torch_dtype=torch.bfloat16).eval().cuda()
prompt = 'The first name of the current US president is "'
prompt_tokens = tokenizer(prompt, add_special_tokens=False, return_tensors="pt").input_ids.cuda()
context = """Joe Biden, born in Scranton, Pennsylvania, on November 20, 1942, had a modest upbringing in a middle-class family. He attended the University of Delaware, where he double-majored in history and political science, graduating in 1965. Afterward, he earned his law degree from Syracuse University College of Law in 1968.\nBiden's early political career began in 1970 when he was elected to the New Castle County Council in Delaware. In 1972, tragedy struck when his wife Neilia and 1-year-old daughter Naomi were killed in a car accident, and his two sons, Beau and Hunter, were injured. Despite this devastating loss, Biden chose to honor his commitment and was sworn in as a senator by his sons' hospital bedsides.\nHe went on to serve as the United States Senator from Delaware for six terms, from 1973 to 2009. During his time in the Senate, Biden was involved in various committees and was particularly known for his expertise in foreign affairs, serving as the chairman of the Senate Foreign Relations Committee on multiple occasions.\nIn 2008, Joe Biden was selected as the running mate for Barack Obama, who went on to win the presidential election. As Vice President, Biden played an integral role in the Obama administration, helping to shape policies and handling issues such as economic recovery, foreign relations, and the implementation of the Affordable Care Act (ACA), commonly known as Obamacare.\nAfter completing two terms as Vice President, Joe Biden decided to run for the presidency in 2020. He secured the Democratic nomination and faced the incumbent President Donald Trump in the general election. Biden campaigned on a platform of unity, promising to heal the divisions in the country and tackle pressing issues, including the COVID-19 pandemic, climate change, racial justice, and economic inequality.\nIn the November 2020 election, Biden emerged victorious, and on January 20, 2021, he was inaugurated as the 46th President of the United States. At the age of 78, Biden became the oldest person to assume the presidency in American history.\nAs President, Joe Biden has worked to implement his agenda, focusing on various initiatives, such as infrastructure investment, climate action, immigration reform, and expanding access to healthcare. He has emphasized the importance of diplomacy in international relations and has sought to rebuild alliances with global partners.\nThroughout his long career in public service, Joe Biden has been recognized for his commitment to bipartisanship, empathy, and his dedication to working-class issues. He continues to navigate the challenges facing the nation, striving to bring the country together and create positive change for all Americans."""
context_tokens = tokenizer(context, add_special_tokens=False, return_tensors="pt").input_ids.cuda()
summary_vectors = model(context_tokens, output_softprompt=True).softprompt
print(f"Compressing {context_tokens.size(1)} tokens to {summary_vectors.size(1)} summary vectors")
# >>> Compressing 660 tokens to 50 summary vectors
generation_with_summary_vecs = model.generate(prompt_tokens, do_sample=False, softprompt=summary_vectors, max_new_tokens=12)[0]
print("Generation w/ summary vectors:\n" + tokenizer.decode(generation_with_summary_vecs))
# >>> The first name of the current US president is "Joe" and the last name is "Biden".
next_tokens_without_context = model.generate(prompt_tokens, do_sample=False, max_new_tokens=11)[0]
print("Generation w/o context:\n" + tokenizer.decode(next_tokens_without_context))
# >>> The first name of the current US president is "Donald" and the last name is "Trump".
05 LLMLingua-2
LLMLingua-2[6] 發(fā)現(xiàn),通過(guò)基于因果語(yǔ)言模型(如LLaMa-7B)的信息熵刪除 tokens 或詞匯單位(lexical units)來(lái)進(jìn)行提示詞壓縮存在兩大挑戰(zhàn):
(1) 用來(lái)計(jì)算信息熵的小型語(yǔ)言模型與提示詞壓縮的實(shí)際目標(biāo)不一致。
(2) 這一方法僅依賴(lài)于單向的上下文信息,而這或許無(wú)法覆蓋提示詞壓縮所需的所有必要信息。
這些問(wèn)題的核心在于,基于信息熵(information entropy)進(jìn)行提示詞壓縮可能并非是最優(yōu)的選擇。
LLMLingua-2 的整體架構(gòu)如圖 15 所示:
圖 15:LLMLingua-2的架構(gòu)總覽。來(lái)源:LLMLingua-2[6]
針對(duì)第一個(gè)問(wèn)題,LLMLingua-2 引入了數(shù)據(jù)蒸餾流程。該流程從大語(yǔ)言模型中提取知識(shí),在不丟失關(guān)鍵信息的情況下壓縮提示詞。同時(shí),它還構(gòu)建了一個(gè) extractive text compression dataset (譯者注:從原始文本中挑選出最重要的句子、短語(yǔ)或詞匯,直接組成一個(gè)較短的版本,以保留原文的主要信息和意義。一般來(lái)說(shuō)不涉及生成新的句子來(lái)概括原文)。在這樣的數(shù)據(jù)集上進(jìn)行訓(xùn)練,有助于小型語(yǔ)言模型更精準(zhǔn)地對(duì)齊提示詞壓縮的需求。
面對(duì)第二個(gè)問(wèn)題,LLMLingua-2 采取了一種創(chuàng)新策略 ------ 將提示詞壓縮轉(zhuǎn)化為詞元(token)分類(lèi)任務(wù)。這一策略確保了壓縮后的提示詞能忠實(shí)地反映原始提示詞的意圖。它選用 transformer 的編碼器作為底層架構(gòu),能夠充分利用完整的雙向上下文信息(bidirectional context),捕捉到進(jìn)行提示詞壓縮所需的全部必要細(xì)節(jié)。
5.1 如何構(gòu)建有效的提示詞壓縮數(shù)據(jù)集?
數(shù)據(jù)蒸餾
數(shù)據(jù)蒸餾從大語(yǔ)言模型(比如 GPT-4)中抽取知識(shí),以便在不丟失基本信息的情況下實(shí)現(xiàn)有效壓縮提示詞。
在 LLMLingua-2 這一項(xiàng)目中,指導(dǎo)性提示詞的設(shè)計(jì)經(jīng)過(guò)了精心設(shè)計(jì),如圖 16 所示。這些指導(dǎo)性提示詞(instructions)指導(dǎo) GPT-4 在不向生成文本中引入新詞匯的前提下,剔除原始文本中的冗余詞匯,從而實(shí)現(xiàn)文本的壓縮。
與此同時(shí),這些指導(dǎo)性提示詞(instructions)并未強(qiáng)行規(guī)定壓縮的比例。相反,GPT-4 被鼓勵(lì)盡可能地縮減原始文本的體積,但前提是必須確保原始信息的完整性。
圖 16:LLMLingua-2 中用于數(shù)據(jù)蒸餾的指導(dǎo)性提示詞。
如圖 17 所示,在處理非常長(zhǎng)的文本時(shí),GPT-4 傾向于采取高比例的壓縮策略??赡苁且?yàn)槠涮幚黹L(zhǎng)文本的能力有限。這種激進(jìn)的壓縮策略往往伴隨著大量信息的流失,可能?chē)?yán)重影響接下來(lái)的任務(wù)執(zhí)行效果。
圖 17:在 MeetingBank 數(shù)據(jù)集上,根據(jù)原始文本長(zhǎng)度,GPT-4 的壓縮比情況。在本研究中,我們使用了 GPT-4–32k ,并將輸出 tokens 的數(shù)量上限設(shè)為 4096。來(lái)源:LLMLingua-2[6]。
為了解決這個(gè)問(wèn)題,LLMLingua-2 引入了一種分塊壓縮(chunk compression) 技術(shù),即先將長(zhǎng)文本拆解為若干個(gè)不超過(guò) 512 tokens 的小文本塊,再分別對(duì)每一小文本塊進(jìn)行壓縮處理,由 GPT-4 來(lái)完成這一過(guò)程。
數(shù)據(jù)標(biāo)注
現(xiàn)在,我們已經(jīng)利用數(shù)據(jù)蒸餾手段,收集到了原始文本與其壓縮內(nèi)容的組合。數(shù)據(jù)標(biāo)注的目的是為原始文本里的每個(gè) token 標(biāo)上一個(gè)二元標(biāo)簽,以此判斷壓縮后該字符是否應(yīng)該被保留。
考慮到 GPT-4 不一定能夠完美遵循指導(dǎo)性提示詞,LLMLingua-2 采取了滑動(dòng)窗口策略(sliding window) ,以此來(lái)限定搜索范圍。同時(shí),還引入了模糊匹配技術(shù)(fuzzy matching) ,有效處理了 GPT-4 在提示詞壓縮過(guò)程中對(duì)原始詞匯可能做出的細(xì)微改動(dòng)。
質(zhì)量控制
在 LLMLingua-2 項(xiàng)目中,質(zhì)量控制環(huán)節(jié)采用了兩個(gè)關(guān)鍵指標(biāo)來(lái)評(píng)估通過(guò) GPT-4 蒸餾生成的壓縮文本,以及自動(dòng)標(biāo)注標(biāo)簽的優(yōu)劣:Variation Rate(VR) 和Alignment Gap(AG) 。
Variation Rate(VR)衡量的是,壓縮后的文本與原始文本相比,有多少比例的詞匯發(fā)生了改變。而Alignment Gap(AG),則是用來(lái)衡量自動(dòng)標(biāo)注的標(biāo)簽的精準(zhǔn)程度。
通過(guò)這些評(píng)估指標(biāo),LLMLingua-2 便能有效地篩除不合格的樣本,從而保障整個(gè)數(shù)據(jù)集質(zhì)量。
5.2 Compressor 壓縮器
將其視為二元分類(lèi)問(wèn)題
從本質(zhì)上講,可將提示詞壓縮問(wèn)題重塑為二元分類(lèi)問(wèn)題。其基本概念是將每一個(gè)詞匯單元視為一個(gè)獨(dú)立的實(shí)體,并為其分配一個(gè)標(biāo)簽:??"保留"?
?? 或 ??"丟棄"?
?。這一策略不僅確保了壓縮后提示詞內(nèi)容的完整性,同時(shí)還簡(jiǎn)化了模型結(jié)構(gòu)。
模型架構(gòu)設(shè)計(jì)
采用了基于 Transformer 編碼器的特征編碼器(feature encoder),并在其上巧妙地疊加了一個(gè)線(xiàn)性分類(lèi)層(linear classification layer)。
這樣的架構(gòu)設(shè)計(jì)使得模型能夠深刻理解每個(gè)詞匯單元的雙向上下文信息,為高效完成壓縮任務(wù)奠定了堅(jiān)實(shí)的基礎(chǔ)。
提示詞壓縮策略
壓縮原始提示詞 ??x?
?? 的策略分為三個(gè)步驟。目標(biāo)壓縮比率設(shè)定為 ??1/τ?
??,這里 ??τ?
?? 即為壓縮后提示詞的詞匯量與原始提示詞 ??x?
? 的詞匯量之商。
- 首先,我們計(jì)算出壓縮后提示詞 ?
?x??
?? 需要保留的 token 數(shù)量:??N? = τN?
?。 - 隨后,運(yùn)用 token 分類(lèi)模型來(lái)預(yù)估每個(gè)詞匯 ?
?xi?
?? 被標(biāo)定為"保留"的概率 ??pi?
?。 - 最后,我們從原始提示詞 ?
?x?
?? 中篩選出前 ??N??
?? 個(gè) ??pi?
?? 值最高的詞匯,嚴(yán)格保持其原有排列順序,進(jìn)而組成壓縮后的提示詞 ??x??
?。
5.3 代碼演示
從上文可以看出,LLMLingua-2 的主要工作是構(gòu)建壓縮器(compressor)。那么,當(dāng)我們成功獲取了這個(gè)壓縮器之后,下一步該如何操作呢?
請(qǐng)參照下方的代碼示例(環(huán)境配置方式與 LLMLingua 一致)。compress_prompt_llmlingua2[20] 函數(shù)內(nèi)集中體現(xiàn)了主要的處理邏輯。
from llmlingua import PromptCompressor
PROMPT = "John: So, um, I've been thinking about the project, you know, and I believe we need to, uh, make some changes. I mean, we want the project to succeed, right? So, like, I think we should consider maybe revising the timeline.\n\nSarah: I totally agree, John. I mean, we have to be realistic, you know. The timeline is, like, too tight. You know what I mean? We should definitely extend it."
llm_lingua = PromptCompressor(
model_name = "microsoft/llmlingua-2-xlm-roberta-large-meetingbank",
use_llmlingua2 = True,
)
compressed_prompt = llm_lingua.compress_prompt(PROMPT, rate=0.33, force_tokens = ['\n', '?'])
## Or use LLMLingua-2-small model
# llm_lingua = PromptCompressor(
# model_name="microsoft/llmlingua-2-bert-base-multilingual-cased-meetingbank",
# use_llmlingua2=True,
# )
print('-' * 100)
print("original:")
print(PROMPT)
print('-' * 100)
print("compressed_prompt:")
print(compressed_prompt)
運(yùn)行結(jié)果如圖 18 所示:
圖 18:LLMLingua-2 測(cè)試代碼的運(yùn)行結(jié)果。截圖由原文作者提供
06 RECOMP
RECOMP[7] 創(chuàng)新性地引入了兩類(lèi)經(jīng)過(guò)訓(xùn)練的壓縮器:抽取型(extractive)和概括型(abstractive)。抽取型壓縮器擅長(zhǎng)從已檢索的文檔中精挑細(xì)選出有價(jià)值的部分 ;而概括型壓縮器則通過(guò)融合多篇文檔的精華,自動(dòng)生成摘要。
圖 19 生動(dòng)描繪了壓縮器在 RECOMP 架構(gòu)中的位置。
圖 19:RECOMP 架構(gòu)。圖片來(lái)源:RECOMP
6.1 抽取型壓縮器
給定輸入文檔集中的 n 個(gè)句子 ??[s1, s2, ..., sn]?
?? ,使用一個(gè)雙編碼器模型(dual encoder model)進(jìn)行訓(xùn)練。該模型能將每個(gè)句子 ??si?
?? 和輸入序列 ??x?
?? 轉(zhuǎn)換為固定長(zhǎng)度的向量表征。這些嵌入向量的內(nèi)積反映了將句子 ??si?
?? 添加到輸入序列 ??x?
? 中,對(duì)于大語(yǔ)言模型(LLM)生成目標(biāo)輸出序列(target output sequence)的幫助程度。
壓縮器最終生成的摘要 ??s?
?? 由排名前 ??N?
? 的句子組成,按照它們與輸入序列的內(nèi)積進(jìn)行排序。
6.2 概括型壓縮器
概括型壓縮器采用的是編碼器-解碼器架構(gòu)(encoder-decoder)。它處理輸入序列 ??x?
?? 與檢索出的文檔集合并將其連接起來(lái),進(jìn)而產(chǎn)生摘要 ??s?
?。
該方法具體步驟如下:首先利用大語(yǔ)言模型(如GPT-3)來(lái)生成訓(xùn)練數(shù)據(jù)集;然后對(duì)數(shù)據(jù)集進(jìn)行篩選;最后,使用經(jīng)過(guò)篩選后的數(shù)據(jù)集來(lái)訓(xùn)練編碼器-解碼器模型(encoder-decoder model)。
6.3 代碼演示
鑒于 RECOMP 當(dāng)前尚處在開(kāi)發(fā)初期,我們?cè)诖藭翰贿M(jìn)行演示。對(duì)此感興趣的讀者不妨親自動(dòng)手體驗(yàn)一番。
07 結(jié)論 Conclusion
本文探討了提示詞壓縮技術(shù),覆蓋了該技術(shù)的方法分類(lèi)、算法原理以及代碼實(shí)踐演示。
在本文所討論的各種方法中,LongLLMLingua 或許是一個(gè)更為出色的選擇 。我們已在項(xiàng)目實(shí)踐中應(yīng)用了這一方法。原文作者承諾一旦他們發(fā)現(xiàn)了 LongLLMLingua 存在的不足,或是發(fā)現(xiàn)了更為優(yōu)秀的替代方案,他們將對(duì)原文(??https://ai.gopubby.com/advanced-rag-09-prompt-compression-95a589f7b554?? )進(jìn)行更新(譯者注:如果有小伙伴關(guān)注到了內(nèi)容更新,請(qǐng)?jiān)谙路搅粞?,我們?huì)盡量及時(shí)進(jìn)行內(nèi)容補(bǔ)充,感謝??。)。此外,LLMLingua-2 也值得一試,它在運(yùn)行速度和內(nèi)存消耗方面都表現(xiàn)優(yōu)異。
Thanks for reading!
Florian June
An artificial intelligence researcher, mainly write articles about Large Language Models, data structures and algorithms, and NLP.
END
參考資料
[1]??https://arxiv.org/pdf/2304.12102.pdf??
[2]??https://arxiv.org/pdf/2310.05736.pdf??
[3]??https://arxiv.org/pdf/2310.06839.pdf??
[4]??https://arxiv.org/pdf/2305.14788.pdf??
[5]??https://arxiv.org/pdf/2304.08467.pdf??
[6]??https://arxiv.org/pdf/2403.12968.pdf??
[7]??https://arxiv.org/pdf/2310.04408.pdf??
[8]??https://arxiv.org/pdf/2210.09461.pdf??
[9]??https://aclanthology.org/2022.acl-long.1.pdf??
[10]??https://github.com/liyucheng09/Selective_Context/blob/v0.1.0rc1/src/selective_context/??init.py#L273
[11]??https://github.com/liyucheng09/Selective_Context/blob/v0.1.0rc1/src/selective_context/??init.py#L100
[12]??https://github.com/liyucheng09/Selective_Context/blob/v0.1.0rc1/src/selective_context/??init.py#L146
[13]??https://github.com/liyucheng09/Selective_Context/blob/v0.1.0rc1/src/selective_context/??init.py#L236
[14]??https://github.com/microsoft/LLMLingua/blob/v0.2.1/llmlingua/prompt_compressor.py#L1108??
[15]??https://github.com/microsoft/LLMLingua/blob/v0.2.1/llmlingua/prompt_compressor.py#L1458??
[16]??https://github.com/microsoft/LLMLingua/blob/v0.2.1/llmlingua/prompt_compressor.py#L1967??
[17]??https://github.com/microsoft/LLMLingua/blob/v0.2.1/llmlingua/prompt_compressor.py#L958??
[18]??https://github.com/microsoft/LLMLingua/blob/v0.2.1/llmlingua/prompt_compressor.py#L1686??
[19]??https://github.com/princeton-nlp/AutoCompressors??
[20]??https://github.com/microsoft/LLMLingua/blob/v0.2.1/llmlingua/prompt_compressor.py#L661??
本文經(jīng)原作者授權(quán),由 Baihai IDP 編譯。如需轉(zhuǎn)載譯文,請(qǐng)聯(lián)系獲取授權(quán)。
原文鏈接:
??https://ai.gopubby.com/advanced-rag-09-prompt-compression-95a589f7b554??
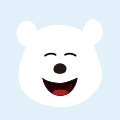