將端側大模型進行到底-MiniCPM3-4B開源
面壁一直都在做端側大模型,之前有文本系列MiniCPM-2B模型,還有多模態(tài)系列的MiniCPM-V系列模型,今天又開源了MiniCPM3-4B模型,真是端側一路走到低。
這次MiniCPM3-4B也是在效果上有了巨大的提升,超過Phi-3.5-mini-Instruct模型,肩比Llama3.1-8B-Instruct、GLM-4-9B-Chat、Qwen2-7B-Instruct等一眾模型,堪稱小模型之王。
之前的MiniCPM-2B模型報告也是干活滿滿,詳見:https://shengdinghu.notion.site/MiniCPM-c805a17c5c8046398914e47f0542095a
這里說一下哈,MiniCPM-2B是1.0版本模型,MiniCPM-1B是2.0版本模型,現(xiàn)在是3.0版本4B。
模型改進
下面是3個版本的模型結構(1->2->3)的區(qū)別:
- 位置編碼:RoPE->RoPE->RoPE
注意力機制:MHA->GQA->MLA,MLA也是DeepSeek-V2的核心創(chuàng)新
- 詞表大小:123K->73K->73K
- 模型層數(shù):40->52->62
- 隱藏層節(jié)點:2304->1536->2560
- 最大長度:4k->4K->32k
- 系統(tǒng)提示詞:不支持->不支持->支持
- 工具調用和代碼解釋器:不支持->不支持->支持
同時,還發(fā)布了RAG套件MiniCPM-Embedding模型和MiniCPM-Reranker模型,針對 RAG場景還發(fā)布了微調版MiniCPM3-RAG-LoRA模型。
模型效果
MiniCPM3-4B模型在中文英文遵循、數(shù)據(jù)推理、代碼能力、工具調用上表現(xiàn)均很不錯的效果。
其中,工具調用能力尤為突出,在Berkeley Function Calling Leaderboard上優(yōu)于Llama3.1-8B-Instruct、GLM-4-9B-Chat、Qwen2-7B-Instruct等更大模型。
長文檔的大海撈針也是全綠。
模型快速使用
PS:模型下載有困難的同學,詳見我之前寫的一篇文章??《大模型下載使我痛苦》??。
from transformers import AutoModelForCausalLM, AutoTokenizer
import torch
# 模型加載
path = "openbmb/MiniCPM3-4B"
tokenizer = AutoTokenizer.from_pretrained(path, trust_remote_code=True)
model = AutoModelForCausalLM.from_pretrained(path, torch_dtype=torch.bfloat16, device_map="cuda", trust_remote_code=True)
# 輸入構造
messages = [
{"role": "user", "content": "你知道劉聰NLP是誰嗎?"},
]
model_inputs = tokenizer.apply_chat_template(messages, return_tensors="pt").to("cuda")
# 模型生成
model_outputs = model.generate(
model_inputs,
max_new_tokens=1024,
top_p=0.8,
temperature=0.9,
repetition_penalty=1.1
)
# 模型解碼
output_token_ids = [
model_outputs[i][len(model_inputs[i]):] for i in range(len(model_inputs))
]
responses = tokenizer.batch_decode(output_token_ids, skip_special_tokens=True)[0]
print(responses)
本文轉載自 ??NLP工作站??,作者: 劉聰NLP
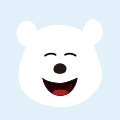