剛剛,DeepSeek開源DeepEP通信庫,千億MoE訓(xùn)推顛覆級創(chuàng)新!FP8狂飆,帶飛GPU 精華
剛剛,DeepSeek放出了開源第二彈——DeepEP!
它擁有高效優(yōu)化的all-to-all通信,并具有以下特點:
- 內(nèi)部節(jié)點和節(jié)點間均支持NVLink和RDMA
- 高吞吐量內(nèi)核用于訓(xùn)練和推理預(yù)填充
- 低延遲推理解碼內(nèi)核
- 本地FP8調(diào)度支持
- 可靈活控制的GPU資源,用于計算-通信重疊
具體來說,DeepEP是一個專為混合專家系統(tǒng)(MoE)和專家并行(EP)設(shè)計的通信庫。
它提供高吞吐量和低延遲的GPU全互聯(lián)內(nèi)核,也被稱為MoE的「調(diào)度」和「組合」操作。該庫還支持低精度運算,包括FP8格式。
DeepEP開源不過一個小時,GitHub星標(biāo)沖破1.5k,還在飚速增長。
項目地址:https://github.com/deepseek-ai/DeepEP
為了配合DeepSeek-V3論文中提出的群組限制門控算法,DeepEP提供了一系列針對不同網(wǎng)絡(luò)域之間帶寬轉(zhuǎn)發(fā)的優(yōu)化內(nèi)核,例如將數(shù)據(jù)從NVLink高速互聯(lián)域轉(zhuǎn)發(fā)到RDMA遠(yuǎn)程直接內(nèi)存訪問域。
這些內(nèi)核具有高吞吐量,適用于模型訓(xùn)練和推理預(yù)填充(預(yù)先計算)任務(wù)。此外,它們還支持對流式多處理器(SM)數(shù)量的精確控制。
針對對延遲敏感的推理解碼任務(wù),DeepEP包含了一組純RDMA實現(xiàn)的低延遲內(nèi)核,以最小化延遲。
該庫還引入了一種基于回調(diào)機制的通信-計算重疊方法,這種方法不會占用任何SM資源。
DeepSeek強調(diào):本庫中的實現(xiàn)可能與DeepSeek-V3論文有些細(xì)微差異。
一位軟件工程師激動地表示,「DeepSeek在MoE模型上所達(dá)到的優(yōu)化水平,令人印象深刻,因為MoE模型因其規(guī)模和復(fù)雜性而廣為人知,難度非常大。而DeepEP能夠如此精確地處理這些問題,使用像NVLink和RDMA這樣的先進(jìn)硬件,并且支持FP8,真是太牛了」。
還有網(wǎng)友稱,這是業(yè)界第一款MoE模型訓(xùn)練和推理通信庫。
DeepEP的這種創(chuàng)新方法,或?qū)⒏淖傾I領(lǐng)域的溝通方式。從此,AI開發(fā)者也許能有效突破大規(guī)模AI模型的界限。
英偉達(dá)未列「特殊指令」,被DeepSeek意外挖掘
為了提高性能,DeepSeek開發(fā)者意外發(fā)現(xiàn),一條在官方文檔中「沒有列出」的特殊指令——ld.global.nc.L1::no_allocate.L2::256B。
這條指令會讓GPU訪問內(nèi)存的方式更高效。
但是,這條指令會導(dǎo)致未定義的行為,因為它使用了.nc修飾符,這會在訪問GPU內(nèi)存時造成一致性問題。
不過,在某些特定的Hopper架構(gòu)硬件上,使用.L1::no_allocate修飾符時,經(jīng)過測試這條指令是安全的,而且性能得到顯著提升。
有網(wǎng)友突然發(fā)現(xiàn)了這個華點——這是非常「硬核」的編碼,完全是那種黑客風(fēng)格的操作,徹底跪了。
隨后,OpenAI華人研究員Clive Chan和網(wǎng)友「main」找到了英偉達(dá)CUDA的官方文檔,發(fā)現(xiàn)在2024年9月時已被收錄。
不過,他又婉轉(zhuǎn)地表示,這個發(fā)現(xiàn)依舊令人驚嘆,任何能夠理解CUDA內(nèi)存模型的人,都值得尊敬。
DeepSeek稱,如果在其他平臺上使用時遇到問題,可以通過在setup.py中設(shè)置DISABLE_AGGRESSIVE_PTX_INSTRS=1來禁用這條指令,或者報告問題。
為了在集群上獲得更好的性能,建議運行所有的測試,并使用自動調(diào)優(yōu)后的最佳配置。默認(rèn)配置已經(jīng)針對 DeepSeek 的內(nèi)部集群進(jìn)行了優(yōu)化。
性能表現(xiàn)
支持NVLink和RDMA轉(zhuǎn)發(fā)的普通內(nèi)核
研究人員使用H800(配備NVLink技術(shù),最大帶寬可達(dá)160 GB/s)進(jìn)行標(biāo)準(zhǔn)內(nèi)核測試,每張顯卡均連接CX7 InfiniBand RDMA網(wǎng)絡(luò)卡(400 Gb/s,最大帶寬可達(dá)50 GB/s)。
測試采用DeepSeek-V3/R1預(yù)訓(xùn)練配置:每批處理4096個token,隱藏層維度為7168,采用top-k組選擇(k=4)和top-k專家選擇(k=8),并使用FP8格式進(jìn)行調(diào)度運算,BF16格式進(jìn)行組合運算。
純RDMA低延遲內(nèi)核測試
他們使用H800測試低延遲內(nèi)核,每張顯卡均連接CX7 InfiniBand RDMA(遠(yuǎn)程直接內(nèi)存訪問)網(wǎng)絡(luò)卡(400 Gb/s,最大帶寬可達(dá)50 GB/s)。
測試采用典型的DeepSeek-V3/R1生產(chǎn)配置:每批處理128個token,隱藏層維度為7168,采用top-k專家選擇(k=8),并使用FP8格式進(jìn)行調(diào)度運算,BF16格式進(jìn)行組合運算。
快速入門
環(huán)境要求
- 英偉達(dá)Hopper GPU(未來可能支持更多架構(gòu)或設(shè)備)
- Python 3.8及以上版本
- CUDA 12.3及以上版本
- PyTorch 2.1及以上版本
- NVLink高速互聯(lián)技術(shù)(用于單機多卡通信)
- RDMA網(wǎng)絡(luò)(用于多機分布式通信)
下載并安裝NVSHMEM依賴
DeepEP依賴于DeepSeek定制修改的NVSHMEM版本。詳細(xì)步驟可參考NVSHMEM安裝指南:
??https://github.com/deepseek-ai/DeepEP/blob/main/third-party/README.md??
開發(fā)
下面代碼片段用于構(gòu)建并測試一個集成NVSHMEM的Python包:
# Build and make symbolic links for SO files
NVSHMEM_DIR=/path/to/installed/nvshmem python setup.py build
# You may modify the specific SO names according to your own platform
ln -s build/lib.linux-x86_64-cpython-38/deep_ep_cpp.cpython-38-x86_64-linux-gnu.so
# Run test cases
# NOTES: you may modify the `init_dist` function in `tests/utils.py`
# according to your own cluster settings, and launch into multiple nodes
python tests/test_intranode.py
python tests/test_internode.py
python tests/test_low_latency.py
安裝
NVSHMEM_DIR=/path/to/installed/nvshmem python setup.py install
然后,在你的Python項目中導(dǎo)入deep_ep,就可以使用啦!
網(wǎng)絡(luò)配置
DeepEP已在InfiniBand網(wǎng)絡(luò)上完成全面測試。理論上,它也兼容融合以太網(wǎng)RDMA(RoCE)。
流量隔離
InfiniBand通過虛擬通道(VL)支持流量隔離。
為防止不同類型流量之間的干擾,團隊建議按以下方式將計算任務(wù)分配到不同的虛擬通道:
- 使用常規(guī)內(nèi)核的計算任務(wù)
- 使用低延遲內(nèi)核的計算任務(wù)
- 其他計算任務(wù)
對于DeepEP,可以通過設(shè)置NVSHMEM_IB_SL環(huán)境變量,來控制虛擬通道分配。
自適應(yīng)路由
自適應(yīng)路由是InfiniBand交換機提供的高級路由功能,可以在多個路徑間均勻分配流量。
目前,低延遲內(nèi)核支持自適應(yīng)路由,而常規(guī)內(nèi)核暫不支持(即將添加支持)。在常規(guī)節(jié)點間內(nèi)核上啟用自適應(yīng)路由,可能導(dǎo)致死鎖(deadlock)或數(shù)據(jù)損壞問題。
對于低延遲內(nèi)核,啟用自適應(yīng)路由可以完全消除由路由沖突引起的網(wǎng)絡(luò)擁塞,但也會引入額外延遲。
團隊建議采用以下配置以獲得最佳性能:
- 在網(wǎng)絡(luò)負(fù)載較重的環(huán)境中啟用自適應(yīng)路由
- 在網(wǎng)絡(luò)負(fù)載較輕的環(huán)境中使用靜態(tài)路由
擁塞控制(Congestion Control)
由于在生產(chǎn)環(huán)境中未觀察到明顯擁塞,因此禁用了擁塞控制功能。
接口和示例
模型訓(xùn)練或推理預(yù)填充示例
常規(guī)內(nèi)核可用于模型訓(xùn)練或推理預(yù)填充階段(預(yù)計算階段,不包含反向傳播部分),如下面的示例代碼所示。
這段代碼實現(xiàn)了一個基于PyTorch的分布式混合專家(MoE)模型的分發(fā)與組合功能,支持前向和反向傳播的通信與計算重疊優(yōu)化。
import torch
import torch.distributed as dist
from typing import List, Tuple, Optional, Union
from deep_ep import Buffer, EventOverlap
# Communication buffer (will allocate at runtime)
_buffer: Optional[Buffer] = None
# Set the number of SMs to use
# NOTES: this is a static variable
Buffer.set_num_sms(24)
# You may call this function at the framework initialization
def get_buffer(group: dist.ProcessGroup, hidden_bytes: int) -> Buffer:
global _buffer
# NOTES: you may also replace `get_*_config` with your auto-tuned results via all the tests
num_nvl_bytes, num_rdma_bytes = 0, 0
for config in (Buffer.get_dispatch_config(group.size()), Buffer.get_combine_config(group.size())):
num_nvl_bytes = max(config.get_nvl_buffer_size_hint(hidden_bytes, group.size()), num_nvl_bytes)
num_rdma_bytes = max(config.get_rdma_buffer_size_hint(hidden_bytes, group.size()), num_rdma_bytes)
# Allocate a buffer if not existed or not enough buffer size
# NOTES: the adaptive routing configuration of the network **must be off**
if _buffer is None or _buffer.group != group or _buffer.num_nvl_bytes < num_nvl_bytes or _buffer.num_rdma_bytes < num_rdma_bytes:
_buffer = Buffer(group, num_nvl_bytes, num_rdma_bytes)
return _buffer
def get_hidden_bytes(x: torch.Tensor) -> int:
t = x[0] if isinstance(x, tuple) else x
return t.size(1) * max(t.element_size(), 2)
def dispatch_forward(x: Union[torch.Tensor, Tuple[torch.Tensor, torch.Tensor]],
topk_idx: torch.Tensor, topk_weights: torch.Tensor,
num_experts: int, previous_event: Optional[EventOverlap] = None) -> \
Tuple[Union[torch.Tensor, Tuple[torch.Tensor, torch.Tensor]], torch.Tensor, torch.Tensor, List, Tuple, EventOverlap]:
# NOTES: an optional `previous_event` means a CUDA event captured that you want to make it as a dependency
# of the dispatch kernel, it may be useful with communication-computation overlap. For more information, please
# refer to the docs of `Buffer.dispatch`
global _buffer
# Calculate layout before actual dispatch
num_tokens_per_rank, num_tokens_per_rdma_rank, num_tokens_per_expert, is_token_in_rank, previous_event = \
_buffer.get_dispatch_layout(topk_idx, num_experts,
previous_event=previous_event, async_finish=True,
allocate_on_comm_stream=previous_event is not None)
# Do MoE dispatch
# NOTES: the CPU will wait for GPU's signal to arrive, so this is not compatible with CUDA graph
# For more advanced usages, please refer to the docs of the `dispatch` function
recv_x, recv_topk_idx, recv_topk_weights, num_recv_tokens_per_expert_list, handle, event = \
_buffer.dispatch(x, topk_idx=topk_idx, topk_weights=topk_weights,
num_tokens_per_rank=num_tokens_per_rank, num_tokens_per_rdma_rank=num_tokens_per_rdma_rank,
is_token_in_rank=is_token_in_rank, num_tokens_per_expert=num_tokens_per_expert,
previous_event=previous_event, async_finish=True,
allocate_on_comm_stream=True)
# For event management, please refer to the docs of the `EventOverlap` class
return recv_x, recv_topk_idx, recv_topk_weights, num_recv_tokens_per_expert_list, handle, event
def dispatch_backward(grad_recv_x: torch.Tensor, grad_recv_topk_weights: torch.Tensor, handle: Tuple) -> \
Tuple[torch.Tensor, torch.Tensor, EventOverlap]:
global _buffer
# The backward process of MoE dispatch is actually a combine
# For more advanced usages, please refer to the docs of the `combine` function
combined_grad_x, combined_grad_recv_topk_weights, event = \
_buffer.combine(grad_recv_x, handle, topk_weights=grad_recv_topk_weights, async_finish=True)
# For event management, please refer to the docs of the `EventOverlap` class
return combined_grad_x, combined_grad_recv_topk_weights, event
def combine_forward(x: torch.Tensor, handle: Tuple, previous_event: Optional[EventOverlap] = None) -> \
Tuple[torch.Tensor, EventOverlap]:
global _buffer
# Do MoE combine
# For more advanced usages, please refer to the docs of the `combine` function
combined_x, _, event = _buffer.combine(x, handle, async_finish=True, previous_event=previous_event,
allocate_on_comm_stream=previous_event is not None)
# For event management, please refer to the docs of the `EventOverlap` class
return combined_x, event
def combine_backward(grad_combined_x: Union[torch.Tensor, Tuple[torch.Tensor, torch.Tensor]],
handle: Tuple, previous_event: Optional[EventOverlap] = None) -> \
Tuple[Union[torch.Tensor, Tuple[torch.Tensor, torch.Tensor]], EventOverlap]:
global _buffer
# The backward process of MoE combine is actually a dispatch
# For more advanced usages, please refer to the docs of the `combine` function
grad_x, _, _, _, _, event = _buffer.dispatch(grad_combined_x, handle=handle, async_finish=True,
previous_event=previous_event,
allocate_on_comm_stream=previous_event is not None)
# For event management, please refer to the docs of the `EventOverlap` class
return grad_x, event
此外,在調(diào)度函數(shù)(dispatch function)內(nèi)部,可能無法預(yù)知當(dāng)前進(jìn)程(rank)需要接收的具體token數(shù)量。
如下圖所示,這種情況下系統(tǒng)會采用CPU同步等待機制,等待GPU返回接收完成的計數(shù)信號。
推理解碼(Inference Decoding)應(yīng)用示例
在模型推理的解碼階段,可以使用低延遲內(nèi)核(專為實時推理優(yōu)化)來提升性能。
具體使用方法請參考以下示例代碼:
這段代碼實現(xiàn)了一個低延遲模式的分布式混合專家(MoE)模型的分發(fā)與組合功能,支持PyTorch和CUDA圖優(yōu)化,適用于高效推理。
import torch
import torch.distributed as dist
from typing import Tuple, Optional
from deep_ep import Buffer
# Communication buffer (will allocate at runtime)
# NOTES: there is no SM control API for the low-latency kernels
_buffer: Optional[Buffer] = None
# You may call this function at the framework initialization
def get_buffer(group: dist.ProcessGroup, num_max_dispatch_tokens_per_rank: int, hidden: int, num_experts: int) -> Buffer:
# NOTES: the low-latency mode will consume much more space than the normal mode
# So we recommend that `num_max_dispatch_tokens_per_rank` (the actual batch size in the decoding engine) should be less than 256
global _buffer
num_rdma_bytes = Buffer.get_low_latency_rdma_size_hint(num_max_dispatch_tokens_per_rank, hidden, group.size(), num_experts)
# Allocate a buffer if not existed or not enough buffer size
if _buffer is None or _buffer.group != group or not _buffer.low_latency_mode or _buffer.num_rdma_bytes < num_rdma_bytes:
# NOTES: for best performance, the QP number **must** be equal to the number of the local experts
assert num_experts % group.size() == 0
_buffer = Buffer(group, 0, num_rdma_bytes, low_latency_mode=True, num_qps_per_rank=num_experts // group.size())
return _buffer
def low_latency_dispatch(hidden_states: torch.Tensor, topk_idx: torch.Tensor, num_max_dispatch_tokens_per_rank: int, num_experts: int):
global _buffer
# Do MoE dispatch, compatible with CUDA graph (but you may restore some buffer status once you replay)
recv_hidden_states, recv_expert_count, handle, event, hook = \
_buffer.low_latency_dispatch(hidden_states, topk_idx, num_max_dispatch_tokens_per_rank, num_experts,
async_finish=False, return_recv_hook=True)
# NOTES: the actual tensor will not be received only if you call `hook()`,
# it is useful for double-batch overlapping, but **without any SM occupation**
# If you don't want to overlap, please set `return_recv_hook=False`
# Later, you can use our GEMM library to do the computation with this specific format
return recv_hidden_states, recv_expert_count, handle, event, hook
def low_latency_combine(hidden_states: torch.Tensor,
topk_idx: torch.Tensor, topk_weights: torch.Tensor, handle: Tuple):
global _buffer
# Do MoE combine, compatible with CUDA graph (but you may restore some buffer status once you replay)
combined_hidden_states, event_overlap, hook = \
_buffer.low_latency_combine(hidden_states, topk_idx, topk_weights, handle,
async_finish=False, return_recv_hook=True)
# NOTES: the same behavior as described in the dispatch kernel
return combined_hidden_states, event_overlap, hook
關(guān)于兩個micro-batch的重疊處理機制,請參考下圖。
團隊實現(xiàn)的接收鉤子(receiving hook)接口,允許RDMA網(wǎng)絡(luò)通信在后臺進(jìn)行,這種設(shè)計不會占用GPU SM的計算資源。
需要注意的是,重疊部分的時間可以靈活調(diào)整,因為注意力計算(attention)、調(diào)度(dispatch)、混合專家(MoE)和組合(combine)這四個處理階段的執(zhí)行時間可能并不相同。
因此,可以根據(jù)具體的計算任務(wù)特點來調(diào)整各個階段的配置參數(shù),以獲得最優(yōu)性能。
本文轉(zhuǎn)自新智元 ,作者:新智元
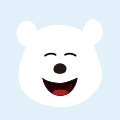