從“無(wú)法找到答案”到“一問(wèn)一個(gè)準(zhǔn)”! Contextual Embedding讓chunk自帶上下文,精準(zhǔn)召回,效果立竿見(jiàn)影! 原創(chuàng)
背景
最近,公司的一個(gè)項(xiàng)目經(jīng)理找我聊了個(gè)頭疼的問(wèn)題:他們給外部交付的項(xiàng)目POC效果不太理想,他發(fā)現(xiàn)從向量庫(kù)中檢索不到想要的信息。起初,我建議他換個(gè)更好的embedding模型,別再用??text-embedding-ada-002?
??了。結(jié)果他反饋說(shuō),試了??text-embedding-3-large?
??和??bge-m3?
?,效果也沒(méi)啥顯著提升。
我仔細(xì)看了他們的數(shù)據(jù),發(fā)現(xiàn)他們上傳了大量用戶的文檔,并對(duì)文檔進(jìn)行了切分,分成一個(gè)個(gè)??chunk?
??,然后召回這些??chunk?
??送給LLM生成回答。問(wèn)題就在于他們切分chunk的方式用的是RecursiveCharacterTextSplitter,單獨(dú)看一個(gè)切分后的??chunk?
??,根本不知道它在講什么。比如,有個(gè)??chunk?
??提到了??opening hours?
??,但因?yàn)檫f歸切分的原因,缺少了主體信息。結(jié)果,即使召回了這個(gè)??chunk?
?,LLM也會(huì)回復(fù)“從提供的上下文中無(wú)法找到答案”。
我給了他一個(gè)建議:可以試試??contextual-embedding?
??。引入這個(gè)方案不需要太多開(kāi)發(fā)成本,而且配合??prompt cache?
?,還能有效減少LLM調(diào)用的開(kāi)銷。
什么是contextual embedding
在傳統(tǒng)的RAG中,文檔通常被分成更小的塊以進(jìn)行有效的檢索。雖然這種方法對(duì)于許多應(yīng)用程序都很有效,但當(dāng)單個(gè)塊缺乏足夠的上下文時(shí),它可能會(huì)導(dǎo)致問(wèn)題。Contextual Embedding 通過(guò)使用LLM給每段chunk補(bǔ)充上下文信息,用戶更精準(zhǔn)召回和更高質(zhì)量的回答。
舉個(gè)簡(jiǎn)單的例子,比如有段chunk的內(nèi)容如下:
The company's revenue grew by 3% over the previous quarter.
當(dāng)我們提問(wèn):"What was the revenue growth for ACME Corp in Q2 2023?",雖然這段chunk是真實(shí)答案,但是卻檢索不到。這是因?yàn)樵糲hunk,是兩個(gè)"The"對(duì)象,導(dǎo)致不管使用embedding還是BM25都抓不出來(lái)它。但是如果我們通過(guò)某種手段,給轉(zhuǎn)換成下面這種contextualized_chunk,把上下文信息給注入到chunk里:
This chunk is from an SEC filing on ACME corp's performance in Q2 2023; the previous quarter's revenue was $314 million. The company's revenue grew by 3% over the previous quarter.
那么還是剛才的問(wèn)題,就一問(wèn)一個(gè)準(zhǔn)了。
原理
我們通過(guò)一個(gè)特定的提示生成每個(gè)分塊的簡(jiǎn)潔上下文,生成的上下文通常為 50-100 個(gè) token,然后 索引之前將其添加到分塊中。對(duì)應(yīng)的prompt示例:
system prompt
Here is the whole document:
<document>
{{WHOLE_DOCUMENT}}
</document>
user prompt
Here is the chunk we want to situate within the whole document:
<chunk>
{{CHUNK_CONTENT}}
</chunk>
Please give a short succinct context to situate this chunk within the overall document for the purposes of improving search retrieval of the chunk. Answer only with the succinct context and nothing else.
實(shí)戰(zhàn)案例
這里我們還是以sentosa的一個(gè)網(wǎng)頁(yè)為例:https://www.sentosa.com.sg/en/places-to-stay/amara-sanctuary-sentosa/。
從這個(gè)網(wǎng)頁(yè)中,我們切分出了一段??chunk?
?,內(nèi)容如下:
description: Bed: King
Room Size: 37 sqm
Maximum Occupants: 2 Adults or 2 Adults and 1 child age 11 and below
Room Essentials
Flat-screen TV with cable channel access
Individually controlled air-conditioning
Spacious bathroom with separate bathtub and shower facilities
Luxury bathroom amenities
Bathrobes and hair dryer
Electronic safe
Tea and coffee making facilities
Iron and ironing board
Baby cot is available on request (subject to availability)
name: Couple Suite
name: Courtyard Suite
name: Junior Suite
name: Verandah Suite
Opening Hours:
Check in: from 3pm
Check out: until 12pm
單獨(dú)看這段??chunk?
??,我們只能看出它在描述一些房間信息,但具體是哪些房間的信息,卻并不清楚。于是,我們使用??gpt-4o-mini?
??為這段??chunk?
?生成了上下文,結(jié)果如下:
This chunk provides detailed information about the room types and amenities available at Amara Sanctuary Sentosa, including the Deluxe Room specifications, other suite options, opening hours, accessibility features, and pet-friendly services, enhancing the overall description of the resort's accommodations.
接下來(lái),我們將原始的??chunk?
??和生成的上下文結(jié)合起來(lái)(使用\n\n 連接chunk),形成一個(gè)新的??chunk?
?:
description: Bed: King
Room Size: 37 sqm
Maximum Occupants: 2 Adults or 2 Adults and 1 child age 11 and below
Room Essentials
Flat-screen TV with cable channel access
Individually controlled air-conditioning
Spacious bathroom with separate bathtub and shower facilities
Luxury bathroom amenities
Bathrobes and hair dryer
Electronic safe
Tea and coffee making facilities
Iron and ironing board
Baby cot is available on request (subject to availability)
name: Couple Suite
name: Courtyard Suite
name: Junior Suite
name: Verandah Suite
Opening Hours:
Check in: from 3pm
Check out: until 12pm
This chunk provides detailed information about the room types and amenities available at Amara Sanctuary Sentosa, including the Deluxe Room specifications, other suite options, opening hours, accessibility features, and pet-friendly services, enhancing the overall description of the resort's accommodations.
這樣一來(lái),當(dāng)我們?cè)僭儐?wèn)關(guān)于“Amara Sanctuary Sentosa的Deluxe Room”相關(guān)問(wèn)題時(shí),LLM就能準(zhǔn)確回答上來(lái)了。這種方法不僅提升了信息的連貫性,還大大減少了LLM的誤判率。
prompt cache
對(duì)于OpenAI模型,當(dāng)你的提示(prompt)長(zhǎng)度超過(guò)1,024個(gè)token時(shí),API調(diào)用將自動(dòng)受益于Prompt Caching功能。(deepseek也支持prompt cache)如果你重復(fù)使用具有相同前綴的提示,系統(tǒng)會(huì)自動(dòng)應(yīng)用Prompt Caching折扣,而你無(wú)需對(duì)API集成做任何修改。緩存通常在5-10分鐘的不活動(dòng)后被清除,并且無(wú)論如何都會(huì)在最后一次使用后的一小時(shí)內(nèi)被移除。
當(dāng)我們對(duì)某個(gè)文檔進(jìn)行切分,生成多個(gè)??chunk?
??時(shí),通常需要為每個(gè)??chunk?
?生成上下文信息。如果每次調(diào)用都傳入全部文檔信息,會(huì)導(dǎo)致重復(fù)計(jì)算,增加LLM的調(diào)用成本。這時(shí),我們可以將全部文檔信息放在system prompt中,利用Prompt Cache來(lái)節(jié)省費(fèi)用。
以下是我調(diào)用LLM的Response中的??usage?
?字段,展示了Prompt Cache的實(shí)際效果:
CompletionUsage(
completion_tokens=24,
prompt_tokens=1584,
total_tokens=1608,
completion_tokens_details=CompletionTokensDetails(
accepted_prediction_tokens=0,
audio_tokens=0,
reasoning_tokens=0,
rejected_prediction_tokens=0
),
prompt_tokens_details=PromptTokensDetails(
audio_tokens=0,
cached_tokens=1536 # 這里顯示有1,536個(gè)token被緩存
)
)
從上面的數(shù)據(jù)可以看出:
- prompt_tokens: 1,584個(gè)token被用于提示。
- cached_tokens: 1,536個(gè)token被緩存,這意味著這部分token的計(jì)算成本被節(jié)省了下來(lái)。
- completion_tokens: 24個(gè)token用于生成回答。
通過(guò)將文檔信息放在system prompt中,我們成功利用Prompt Cache減少了重復(fù)計(jì)算,顯著降低了LLM的調(diào)用成本。
總結(jié)
傳統(tǒng)的文檔切分方法(如RecursiveCharacterTextSplitter)可能會(huì)導(dǎo)致chunk缺乏足夠的上下文信息,從而影響檢索效果。通過(guò)引入Contextual Embedding,我們能夠?yàn)槊總€(gè)chunk補(bǔ)充上下文信息,顯著提升檢索的精準(zhǔn)度和回答的質(zhì)量。
總的來(lái)說(shuō),Contextual Embedding和Prompt Cache的結(jié)合,為RAG系統(tǒng)提供了一種低成本、高效率的優(yōu)化方案。尤其是在項(xiàng)目時(shí)間緊張、資源有限的情況下,這種方案能夠快速提升系統(tǒng)的表現(xiàn)。
本文轉(zhuǎn)載自公眾號(hào)AI 博物院 作者:longyunfeigu
原文鏈接:??https://mp.weixin.qq.com/s/I8muNOkLenngFn9I9U2ZQg??
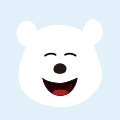