讓AI讀懂PPT圖表!RAG系統(tǒng)從60分到95分的進(jìn)化之路,LlamaParse+多模態(tài)實戰(zhàn)全解析 原創(chuàng)
背景信息
近期前線人員反饋,在使用問答系統(tǒng)處理PPT文件時回答效果不佳。經(jīng)查發(fā)現(xiàn),用戶上傳的PPT內(nèi)容多為圖文混排形式,包含大量圖像和統(tǒng)計圖表。用戶提問主要聚焦于圖表數(shù)據(jù)及其相互關(guān)系。
我這里隨意在網(wǎng)上找了一個PPT做嘗試,這個PPT有一張圖如下所示:
提問:"2017年大數(shù)據(jù)開發(fā)人數(shù)比2016年增加了還是減少了,具體增加或減少了多少人?"
系統(tǒng)返回:
根據(jù)提供的資源數(shù)據(jù),2017年大數(shù)據(jù)開發(fā)職位的招聘人數(shù)大幅增加。具體來說,2016年大數(shù)據(jù)開發(fā)的招聘人數(shù)為5,667人,而2017年則增加到41,831人。因此,2017年大數(shù)據(jù)開發(fā)職位比2016年增加了36,164人 (41,831 - 5,667 = 36,164)。
從圖片我們可以看出明顯存在回答錯誤的問題。
問題分析
目前使用的LangChain UnstructuredPowerPointLoader在解析PPT時存在以下不足:
- 對圖文混排內(nèi)容處理能力弱
- 圖表數(shù)據(jù)提取不準(zhǔn)確
- 語義信息丟失嚴(yán)重
PPT文檔特性
- 非結(jié)構(gòu)化布局:沒有固定格式,圖文表混排
- 視覺化表達(dá):大量使用圖表而非純文本傳遞信息
- 天然分塊:每頁幻燈片構(gòu)成獨立的知識單元
傳統(tǒng)文本提取+RAG的處理方式會丟失視覺元素中的語義信息,這正是當(dāng)前系統(tǒng)效果不佳的主因。
解決方案
隨著現(xiàn)在多模態(tài)大模型的效果越來越強(qiáng),我們就可以使用LVM來解決這類問題。既然僅僅參考從圖片識別出的文本回答不是很準(zhǔn)確,那么我們可不可以考慮使用文本+原始圖片的方式送給LVM來回答呢?
首先借助LVM對PPT進(jìn)行解析,可以解析出每頁幻燈片對應(yīng)的文本和圖片,我們把文本進(jìn)行embedding作為召回,在檢索的時候把檢索到的文本和關(guān)聯(lián)的圖片一起送給大模型用于生成。
LlamaParse
為了方便演示,這里對PPT進(jìn)行解析成文本和圖片我使用的工具是LlamaParse。免費用戶每天有1k頁的額度,夠我們?nèi)粘y試使用。
首先注冊賬號并登錄https://cloud.llamaindex.ai,然后打開LVM功能
接著申請項目對應(yīng)的API key 就可以用來測試了,我們可以通過對應(yīng)的api 來獲取每頁幻燈片的文本內(nèi)容并下載每頁圖片到本地。
LlamaParse是LlamaCloud的一部分,是一個GenAI原生文檔解析器,可以為任何下游LLM用例(RAG、代理)解析復(fù)雜的文檔數(shù)據(jù)。
os.environ["LLAMA_CLOUD_API_KEY"] = "xxx"
parser = LlamaParse(
result_type="json",
use_vendor_multimodal_model=True,
vendor_multimodal_model_name="gemini-2.0-flash-001",
language="ch_sim"
)
md_result = parser.get_json_result(file_path)
doc_id = md_result[0]["job_id"]
pages = md_result[0]["pages"]
# 下載圖片到本地
parser.get_images(md_result, download_path="data_images")
如何索引
- 原文檔的每一頁P(yáng)PT轉(zhuǎn)為圖片,并借助多模態(tài)模型解析成每一頁的Markdown文本
- 將每一頁的Markdown文本塊作為一個Chunk,并根據(jù)頁碼與頁面圖片關(guān)聯(lián)起來(存儲base64編碼/云路徑/本地路徑)。這樣,在檢索時可以根據(jù)文本塊找到對應(yīng)的圖片
- 嵌入這些文本Chunks,并將它們存儲在向量庫中
dataset = []
docs = []
base64_map = {}
for page in pages:
md = page["md"]
page_number = page["page"]
# 查找并上傳對應(yīng)頁碼的圖片
local_image_path = find_image_by_page(
"data_images", doc_id, page_number)
with open(local_image_path, "rb") as image_file:
image_base64 = base64.b64encode(image_file.read()).decode('utf-8')
# 添加到dataset
dataset.append({
'content': md,
'image_base64': image_base64,
'page_number': page_number
})
docs.append(
Document(
page_cnotallow=md,
metadata={
"page_number": page_number,
})
)
base64_map[page_number] = image_base64
vectorstore = Milvus.from_documents(
documents=docs,
embedding=embeddings,
connection_args={"host": "127.0.0.1", "port": "19530"},
drop_old=True, # Drop the old Milvus collection if it exists
collection_name="collection_ppt",
)
檢索和生成
- 從向量庫檢索關(guān)聯(lián)的塊,也就是前面對應(yīng)到PPT頁面的生成文本
- 根據(jù)這些塊中的元數(shù)據(jù),找到對應(yīng)的頁面截圖base64
- 將文本塊組裝成Prompt,與找到的圖片的base64一起輸入多模態(tài)模型,等待響應(yīng)
dat = vectorstore.similarity_search(query=question, k=5)
image_base64_list = []
chunk = []
for doc in dat:
page_number = doc.metadata["page_number"]
print(page_number)
image_base64 = base64_map.get(page_number)
if image_base64:
image_base64_list.append(image_base64)
chunk.append(doc.page_content)
openai_api_key = os.environ["OPENAI_API_KEY"] # 替換為你的 OpenAI API Key
headers = {
"Content-Type": "application/json",
"Authorization": f"Bearer {openai_api_key}"
}
# 構(gòu)建 messages 內(nèi)容
messages_content = [
{"type": "text", "text": """The following is the Markdown text and image information parsed in the slide. Markdown text has attempted to convert the relevant charts into tables. Give priority to using picture information to answer questions. Use Markdown text information only when the image cannot be understood.
Here is the context:
---------
{context}
---------
Question: {question}
""".format(cnotallow="\n".join(chunk), questinotallow=question)}
]
if image_base64_list:
# 添加所有檢索到的圖片
for img_base64 in image_base64_list:
messages_content.append({
"type": "image_url",
"image_url": {
"url": f"data:image/jpeg;base64,{img_base64}"
}
})
payload = {
"model": "gpt-4.1",
"messages": [
{
"role": "user",
"content": messages_content
}
],
"temperature": 0.1,
"max_tokens": 1000# 根據(jù)需要調(diào)整
}
# 發(fā)送請求到 OpenAI API
try:
response = requests.post(
"https://api.openai-proxy.com/v1/chat/completions",
headers=headers,
jsnotallow=payload
)
response.raise_for_status() # 檢查請求是否成功
# 解析并打印結(jié)果
result = response.json()
print("OpenAI 分析結(jié)果:")
print(result["choices"][0]["message"]["content"])
except requests.exceptions.RequestException as e:
print(f"請求 OpenAI API 失敗: {e}")
if hasattr(e, 'response') and e.response:
print(f"錯誤詳情: {e.response.text}")
當(dāng)我們執(zhí)行query:
??2017年大數(shù)據(jù)開發(fā)人數(shù)比2016年增加了還是減少了, 具體增加或者減少了多少人??
?
返回的結(jié)果就是正確的了:
總結(jié)和優(yōu)化
在測試驗證過程中,我們發(fā)現(xiàn)當(dāng)前方案仍存在一些可優(yōu)化的空間,主要涉及準(zhǔn)確性、性能和擴(kuò)展性三個方面:
- 解析準(zhǔn)確性問題
- 多維度數(shù)據(jù)圖表(如組合柱狀圖+折線圖)
- 顏色相近的信息元素
- 非標(biāo)準(zhǔn)圖表類型
- 視覺模型對復(fù)雜圖表的解析仍存在約5-10%的偏差率
- 特別在以下場景容易出錯:
- 性能與成本考量
- 多模態(tài)模型響應(yīng)時間較純文本LLM增加40-60%
- Token消耗量約為普通文本處理的2-3倍
- 高并發(fā)場景下API調(diào)用成本顯著增加
- 檢索效率挑戰(zhàn)
- 檢索準(zhǔn)確率下降15-20%
- 模糊查詢的召回率問題尤為突出
- 相似頁面間容易產(chǎn)生干擾
- 當(dāng)文檔庫擴(kuò)展到100+個PPT時:
基于這些問題,我們可以做如下嘗試:
- 存儲架構(gòu):
a.使用CDN托管解析后的圖片
b.采用對象存儲+臨時URL訪問機(jī)制
c.實現(xiàn)緩存策略(TTL 24h)
- 模型選型:
a.評估不同多模態(tài)模型效果
b.元數(shù)據(jù)增強(qiáng):借助元數(shù)據(jù)粗略過濾一些無關(guān)數(shù)據(jù)
本文轉(zhuǎn)載自公眾號AI 博物院 作者:longyunfeigu
原文鏈接:??https://mp.weixin.qq.com/s/AYdBlzBmkxQBntVX8BN29w??
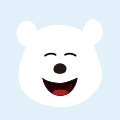