RAG實戰(zhàn) | 向量數(shù)據(jù)庫LanceDB指南
1. LanceDB介紹
LanceDB是一個開源的用 Rust 實現(xiàn)的向量數(shù)據(jù)庫(https://github.com/lancedb/lancedb),它的主要特點是:
- 提供單機服務,可以直接嵌入到應用程序中
- 支持多種向量索引算法,包括Flat、HNSW、IVF等。
- 支持全文檢索,包括BM25、TF-IDF等。
- 支持多種向量相似度算法,包括Cosine、L2等。
- 與Arrow生態(tài)系統(tǒng)緊密集成,允許通過 SIMD 和 GPU 加速在共享內(nèi)存中實現(xiàn)真正的零拷貝訪問。
2. LanceDB安裝
pip install lancedb
預覽版本:
pip install --pre --extra-index-url https://pypi.fury.io/lancedb/ lancedb
3. 快速入門
3.1 連接或者打開數(shù)據(jù)庫
創(chuàng)建數(shù)據(jù)庫:
import lancedb
db = lancedb.connect("./test") # 如果數(shù)據(jù)庫不存在,會自動創(chuàng)建
打開數(shù)據(jù)庫:
db = lancedb.open("./test") # 如果數(shù)據(jù)庫不存在,會報錯
3.2 創(chuàng)建表
data = [
{"vector": [1, 2], "text": "hello"},
{"vector": [3, 4], "text": "world"},
]
table = db.create_table("my_table", data=data, mode="overwrite")
df = pd.DataFrame(data)
table = db.create_table("my_table", data=df, mode="overwrite")
3.3 查看當前 db 中的表
print(db.table_names())
3.4 插入數(shù)據(jù)
data = [
{"vector": [1, 2], "text": "hello"},
{"vector": [3, 4], "text": "world"},
]
table.add(data)
3.5 查詢數(shù)據(jù)
通過向量查詢數(shù)據(jù):
query = [1, 2]
results = table.search(query).limit(1).to_pandas()
通過文本查詢數(shù)據(jù):
query = "hello"
results = table.search(query).limit(1).to_pandas()
3.6 創(chuàng)建索引
table.create_index()
LanceDB 不會自動創(chuàng)建索引,對于數(shù)據(jù)量較大的情況下,建議手動創(chuàng)建,否則會走全文檢索(速度會比較慢)。
3.7 刪除數(shù)據(jù)
table.delete(f'text = "hello"')
刪除數(shù)據(jù)當然也支持 SQL 語法,具體參考官方文檔(https://lancedb.github.io/lancedb/sql/#pre-and-post-filtering)。
3.8 刪除表
db.drop_table("my_table")
注意:如果表不存在,會報錯,可以通過傳入?yún)?shù)忽略錯誤 ??ignore_missing=True?
?。
4. 向量搜索
4.1 什么是向量搜索
向量搜索是一種在高維空間中搜索向量的方法,主要是將原始數(shù)據(jù)通過嵌入模型得到向量,然后通過向量相似度算法計算向量之間的距離,從而找到最相似的向量。
4.2 embedding
embedding 是將原始數(shù)據(jù)通過嵌入模型得到向量的過程,嵌入模型可以是預訓練的模型,也可以是自己訓練的模型,是一種將文本、圖像、音頻等數(shù)據(jù)投影到二維空間的方法。
4.3 索引
和關(guān)系型數(shù)據(jù)庫一樣,向量數(shù)據(jù)庫也需要索引來加速查詢,索引是一種數(shù)據(jù)結(jié)構(gòu),用于快速查找數(shù)據(jù),LanceDB 使用基于磁盤的索引:IVF-PQ,是倒排索引的一種變體,使用PQ 來做壓縮嵌入。
PQ 原理主要分為以下步驟:
- 對向量進行分桶,將向量分為多個桶,每個桶包含多個向量,比如 樣本的維度 D=1024,桶的數(shù)量 M=64,每個桶的維度 16;
- 對樣本的每個段分別進行聚類,聚成 k=256(其中 K 可以定義) 個聚類中心,這樣整個樣本被分為 M*K 個聚類中心,每個聚類中心分配一個 ID(范圍 0-K-1);
- 通過上面的聚類和 ID 分配,每個樣本就會變成量化后的向量,例如 [28, 100, 99, 255 ...];
- 對于新加入樣本按照第一步的分桶方式切分,然后再聚類的分段里面找到最近的類中心,然后將聚類中心的 ID 作為量化后的向量;
通過以上的處理,原來 1024 維度向量*float類型(1024 * 4 字節(jié))被壓縮到 64 個字節(jié),大大減少了存儲空間和計算量,當然量化是有損的,所以對于數(shù)據(jù)量不大的情況,可以不使用索引,直接暴力搜索。
4.4 暴力搜索和 ANN 搜索
- 如果要搜索的準確度,執(zhí)行暴力搜索是一種好的選擇,基本上就是對所有的向量進行相似度計算,然后返回最相似的向量,相當于 kNN 搜索。
- kNN 和每個向量都做距離計算,計算量比較大,所以需要使用 ANN 搜索,ANN 搜索是一種基于樹的搜索方法,使用樹結(jié)構(gòu)來存儲向量,然后通過樹的搜索來找到最相似的向量。
4.5 HNSW
HNSW 是一種基于圖的搜索方法,使用圖結(jié)構(gòu)來存儲向量,然后通過圖的搜索來找到最相似的向量,原理類似跳躍表,通過分層的 k-ANN 圖來實現(xiàn)遞歸搜索。
LanceDB 創(chuàng)建 HNSW 索引樣例:
data = [
{"vector": row, "item": f"item {i}"}
for i, row in enumerate(np.random.random((10_000, 1536)).astype('float32'))
]
tbl = db.create_table("my_vectors", data=data)
tbl.create_index(index_type=IVF_HNSW_SQ)
5. LanceDB 使用指南
5.1 通過 Pandas DataFrame 插入數(shù)據(jù)
import pandas as pd
import lancedb
import numpy as np
db = lancedb.connect("./test")
table = db.create_table("my_table", data=[], mode="overwrite")
df = pd.DataFrame({
"vector": [np.random.rand(100) for _ in range(100)],
"text": [f"hello {i}" for i in range(100)],
})
table.add(df)
5.2 通過 Arrow Table 插入數(shù)據(jù)
import pyarrow as pa
import lancedb
import numpy as np
db = lancedb.connect("./test")
table = db.create_table("my_table", data=[], mode="overwrite")
table = db.create_table("my_table", data=pa.Table.from_pandas(df), mode="overwrite")
5.3 通過 Model 插入數(shù)據(jù)
import lancedb
from lancedb.pydantic import LanceModel
class MyModel(LanceModel):
vector: list[float]
text: str
db = lancedb.connect("./test")
table = db.create_table("my_table", schema=MyModel, mode="overwrite")
model = MyModel(vector=[1, 2], text="hello")
table.add(model)
5.4 通過迭代器寫入大規(guī)模數(shù)據(jù)
import lancedb
import pyarrow as pa
def make_batches():
for i in range(1000):
yield pa.Table.from_pandas(pd.DataFrame({
"vector": [np.random.rand(100) for _ in range(100)],
"text": [f"hello {i}"for i in range(100)],
}))
schema = pa.schema(
[
pa.field("vector", pa.list_(pa.float32(), 4)),
pa.field("item", pa.utf8()),
]
)
db = lancedb.connect("./test")
table = db.create_table("my_table", make_batches(), schema=schema, mode="overwrite")
或者通過迭代器寫入數(shù)據(jù):
import lancedb
import pyarrow as pa
def make_batches():
for i in range(1000):
yield pa.Table.from_pandas(pd.DataFrame({
"vector": [np.random.rand(100) for _ in range(100)],
"text": [f"hello {i}" for i in range(100)],
}))
db = lancedb.connect("./test")
table = db.create_table("my_table", data=[], mode="overwrite")
table.add(make_batches())
5.5 刪除指定的數(shù)據(jù)
db = lancedb.connect("./test")
data = [
{"x": 1, "vector": [1, 2]},
{"x": 2, "vector": [3, 4]},
{"x": 3, "vector": [5, 6]},
]
# Synchronous client
table = db.create_table("delete_row", data)
table.to_pandas()
# x vector
# 0 1 [1.0, 2.0]
# 1 2 [3.0, 4.0]
# 2 3 [5.0, 6.0]
table.delete("x = 2")
table.to_pandas()
# x vector
# 0 1 [1.0, 2.0]
# 1 3 [5.0, 6.0]
5.6 更新數(shù)據(jù)
db = lancedb.connect("./test")
data = [
{"x": 1, "vector": [1, 2]},
{"x": 2, "vector": [3, 4]},
{"x": 3, "vector": [5, 6]},
]
# Synchronous client
table = db.create_table("update_row", data)
table.update(where="x = 2", values={"vector": [10, 10]})
5.7 一致性
由于 lancedb 是嵌入到各個應用中,所以數(shù)據(jù)更新并不能保持一致,可以通過設置 ??read_consistency_interval?
? 參數(shù)來保證數(shù)據(jù)更新的一致性。
??read_consistency_interval?
? 是一個時間間隔,單位是秒。
- 不設置,數(shù)據(jù)庫不檢查其他進程對表所做的更新。這提供了最佳查詢性能,但意味著客戶端可能無法看到最新的數(shù)據(jù),此設置適用于在表引用的生命周期內(nèi)數(shù)據(jù)不會發(fā)生變化的應用程序。
- 如果設置為 0,數(shù)據(jù)庫在每次讀取時檢查更新。這提供了最強的一致性保證,確保所有客戶端都看到最新提交的數(shù)據(jù),但是,它的開銷最大。當一致性比高 QPS 更重要時,此設置是合適的。
- 自定義間隔時間,數(shù)據(jù)庫以自定義間隔(例如每 5 秒)檢查更新。這提供了最終一致性,允許寫入和讀取操作之間有一些滯后,從性能方面來看,這是強一致性和無一致性檢查之間的中間地帶,此設置適用于即時一致性并不重要但客戶端最終應該看到更新數(shù)據(jù)的應用程序。
from datetime import timedelta
uri = "data/sample-lancedb"
# 保障數(shù)據(jù)最終一致性
db = lancedb.connect(uri, read_consistency_interval=timedelta(secnotallow=5))
tbl = db.open_table("test_table")
5.8 構(gòu)建 ANN 索引
import lancedb
import numpy as np
db = lancedb.connect("./test")
data = [
{"vector": row, "item": f"item {i}"}
for i, row in enumerate(np.random.random((10_000, 1536)).astype('float32'))
]
tbl = db.create_table("my_vectors", data=data)
tbl.create_index(distance_type='l2', num_partitinotallow=2, num_sub_vectors=4)
- distance_type:距離度量算法,可以參考(cosine, l2)等;
- num_partitions: 分區(qū)數(shù)量;
- num_sub_vectors:子向量數(shù)量,PQ 的子向量數(shù)量;
- num_bits:用于編碼的子向量的位數(shù),支持 4 和 8;
如果需要通過 CUDA 加速,可以增加參數(shù):
tbl.create_index(distance_type='l2', num_partitinotallow=2, num_sub_vectors=4, accelerator='cuda')
5.9 搜索數(shù)據(jù)
1)kNN 搜索:不建立索引,就會掃描全表,計算每個向量的距離,然后返回最相似的 k 個向量,也可以指定距離度量算法。
query = np.random.random(1536).astype('float32')
results = tbl.search(query).limit(10).distance_type("cosine").to_pandas()
2)ANN 搜索:通過索引搜索,支持 nprobes 和 refine_factor 參數(shù)。
- nprobes 數(shù)字越大,搜索越準確,但是速度越慢;
- refine_factor 對召回的進行重排優(yōu)化;
query = np.random.random(1536).astype('float32')
tbl.search(query).limit(2).nprobes(20).refine_factor(
10
).to_pandas()
3)基于距離范圍搜索:主要用于不通過topk 查詢,而是通過距離范圍查詢。
query = np.random.random(1536).astype('float32')
tbl.search(query).distance_range(0.1, 0.5).to_pandas()
4)全文搜索:如果需要對字符串進行索引,并通過關(guān)鍵字搜索進行查詢,可以通過創(chuàng)建 FTS 索引。
from lancedb.index import FTS
tbl = db.create_table("my_vectors", data=[
{"vector": np.rand.random(10), "item": f"this item {i}"}
{"vector": np.rand.random(10), "item": f"this item {i + 100}"}
])
tbl.create_fts_index("text", use_tantivy=False)
tbl.search("this item 10").limit(10).select(["item"]).to_pandas()
5)過濾搜索:通過 SQL 語法進行過濾搜索。
tbl.search("this item 10").limit(10).where("item='this'", prefilter=True).to_pandas()
5.10 SQL 語法
LanceDB 支持 SQL 語法如下:
>, <, >=, <=
AND, OR, NOT
IS NULL, IS NOT NULL
IS TRUE, IS FALSE
IN
LIKE, NOT LIKE
CAST
regexp_match(column, pattern)
樣例如下:
table.search("this item 10").where(
"(item IN ('item 0', 'item 2')) AND (id > 10)"
).to_arrow()
6. LanceDB 結(jié)合 embedding
6.1 注冊 embedding 模型
LanceDB 支持結(jié)合 embedding 模型進行搜索。
import lancedb
from lancedb.pydantic import LanceModel, Vector
from lancedb.embeddings import get_registry
db = lancedb.connect("./test")
func = get_registry().get("openai").create(name="text-embedding-ada-002")
class Words(LanceModel):
text: str = func.SourceField()
vector: Vector(func.ndims()) = func.VectorField()
table = db.create_table("words", schema=Words, mode="overwrite")
table.add(
[
{"text": "hello world"},
{"text": "goodbye world"}
]
)
query = "greetings"
actual = table.search(query).limit(1).to_pydantic(Words)[0]
print(actual.text)
通過 get_registry() 注冊不同的模型參數(shù),其中支持的代碼如下:
- ?
?get_registry().get("sentence-transformers").create(name="BAAI/bge-small-en-v1.5", device="cpu")?
? - ?
?get_registry().get("huggingface").create(name='facebook/bart-base')?
? - ?
?get_registry().get("ollama").create(name="nomic-embed-text")?
? - ?
?get_registry().get("openai").create(name="text-embedding-ada-002")?
? - ?
?et_registry().get("instructor").create(source_instructinotallow="represent the docuement for retreival", query_instructinotallow="represent the document for retreiving the most similar documents")?
? - ?
?get_registry().get("gemini-text").create()?
? - ?
?get_registry().get("open-clip").create()?
? - ?
?get_registry().get("imagebind").create()?
?...
6.2 完整的使用樣例
1)注冊 embedding 函數(shù)
from lancedb.embeddings import get_registry
registry = get_registry()
clip = registry.get("open-clip").create()
2)定義數(shù)據(jù)模型
from lancedb.pydantic import LanceModel, Vector
from typing import List
class Document(LanceModel):
id: str
vector: Vector(clip.ndims()) = clip.VectorField()
image_uri: str = clip.SourceField()
3)創(chuàng)建表并添加數(shù)據(jù)
db = lancedb.connect("~/lancedb")
table = db.create_table("pets", schema=Pets)
table.add([{"image_uri": u} for u in uris])
4)查詢數(shù)據(jù)
results = (
table.search("dog")
.limit(10)
.to_pandas()
)
參考
(1)??https://lancedb.github.io/lancedb/??
(2)https://excalidraw-phi-woad.vercel.app/
本文轉(zhuǎn)載自??周末程序猿??,作者:周末程序猿
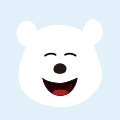