使用Python進(jìn)行線性規(guī)劃示例
運(yùn)籌學(xué)
運(yùn)籌學(xué)是一種科學(xué)的決策方法,它通常是在需要分配稀缺資源的條件下,尋求系統(tǒng)的優(yōu)秀設(shè)計(jì)??茖W(xué)的決策方法需要使用一個(gè)或多個(gè)數(shù)學(xué)模型(優(yōu)化模型)來(lái)做出最優(yōu)決策。
優(yōu)化模型試圖在滿足給定約束的決策變量的所有值的集合中,找到優(yōu)化(最大化或最小化)目標(biāo)函數(shù)的決策變量的值。 它的三個(gè)主要組成部分是:
- 目標(biāo)函數(shù):要優(yōu)化的函數(shù)(最大化或最小化)。
- 決策變量:影響系統(tǒng)性能的可控變量。
- 約束:決策變量的一組約束(即線性不等式或等式)。非負(fù)性約束限制了決策變量取正值。
優(yōu)化模型的解稱為最優(yōu)可行解。
建模步驟
對(duì)運(yùn)籌學(xué)問題進(jìn)行準(zhǔn)確建模是很重要的任務(wù),也是很困難的任務(wù)。錯(cuò)誤的模型會(huì)導(dǎo)致錯(cuò)誤的解決方案,從而不能解決原來(lái)的問題。團(tuán)隊(duì)成員應(yīng)按照以下步驟進(jìn)行建模:
- 問題定義:定義項(xiàng)目的范圍,并確定三個(gè)要素:決策變量、目標(biāo)和限制(即約束)。
- 模型構(gòu)建:將問題定義轉(zhuǎn)化為數(shù)學(xué)關(guān)系。
- 模型求解:使用標(biāo)準(zhǔn)優(yōu)化算法。在獲得解后,需要進(jìn)行靈敏度分析,以找出由于某些參數(shù)的變化而導(dǎo)致的解的行為。
- 模型有效性:檢查模型是否按預(yù)期工作。
- 實(shí)現(xiàn):將模型和結(jié)果轉(zhuǎn)換為解決方案。
線性規(guī)劃
線性規(guī)劃(Linear Programming,也稱為L(zhǎng)P)是一種運(yùn)籌學(xué)技術(shù),當(dāng)當(dāng)所有的目標(biāo)和約束都是線性的(在變量中)并且當(dāng)所有的決策變量都是連續(xù)的時(shí)使用。線性規(guī)劃是最簡(jiǎn)單的運(yùn)籌學(xué)方法。
Python的SciPy庫(kù)包含用于解決線性編程問題的linprog函數(shù)。在使用linprog時(shí),編寫代碼要考慮的兩個(gè)注意事項(xiàng):
- 這個(gè)問題必須表述為一個(gè)最小化問題。
- 不等式必須表示為≤。
最小化問題
讓我們考慮以下要解決的最小化問題:
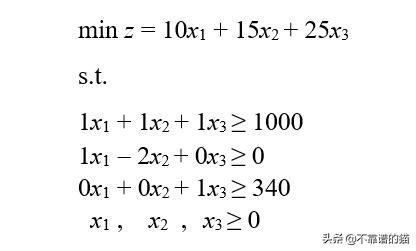
讓我們看一下Python代碼:
- # Import required libraries
- import numpy as np
- from scipy.optimize import linprog
- # Set the inequality constraints matrix
- # Note: the inequality constraints must be in the form of <=
- A = np.array([[-1, -1, -1], [-1, 2, 0], [0, 0, -1], [-1, 0, 0], [0, -1, 0], [0, 0, -1]])
- # Set the inequality constraints vector
- b = np.array([-1000, 0, -340, 0, 0, 0])
- # Set the coefficients of the linear objective function vector
- c = np.array([10, 15, 25])
- # Solve linear programming problem
- res = linprog(c, A_ub=A, b_ub=b)
- # Print results
- print('Optimal value:', round(res.fun, ndigits=2),
- '\nx values:', res.x,
- '\nNumber of iterations performed:', res.nit,
- '\nStatus:', res.message)
輸出結(jié)果:
- # Optimal value: 15100.0
- # x values: [6.59999996e+02 1.00009440e-07 3.40000000e+02]
- # Number of iterations performed: 7
- # Status: Optimization terminated successfully.
最大化問題
由于Python的SciPy庫(kù)中的linprog函數(shù)是用來(lái)解決最小化問題的,因此有必要對(duì)原始目標(biāo)函數(shù)進(jìn)行轉(zhuǎn)換。通過將目標(biāo)函數(shù)的系數(shù)乘以-1(即通過改變其符號(hào)),可以將最小化問題轉(zhuǎn)化為一個(gè)最大化問題。
讓我們考慮下面需要解決的最大化問題:
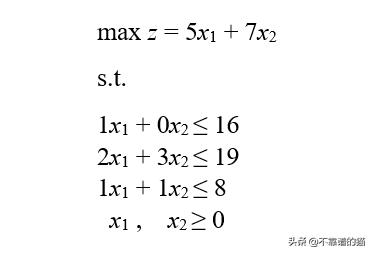
讓我們看一下Python的實(shí)現(xiàn):
- # Import required libraries
- import numpy as np
- from scipy.optimize import linprog
- # Set the inequality constraints matrix
- # Note: the inequality constraints must be in the form of <=
- A = np.array([[1, 0], [2, 3], [1, 1], [-1, 0], [0, -1]])
- # Set the inequality constraints vector
- b = np.array([16, 19, 8, 0, 0])
- # Set the coefficients of the linear objective function vector
- # Note: when maximizing, change the signs of the c vector coefficient
- c = np.array([-5, -7])
- # Solve linear programming problem
- res = linprog(c, A_ub=A, b_ub=b)
- # Print results
- print('Optimal value:', round(res.fun*-1, ndigits=2),
- '\nx values:', res.x,
- '\nNumber of iterations performed:', res.nit,
- '\nStatus:', res.message)
上述代碼的輸出結(jié)果為:
- # Optimal value: 46.0
- # x values: [5. 3.]
- # Number of iterations performed: 5
- # Status: Optimization terminated successfully.
最后
線性規(guī)劃為更好的決策提供了一種很好的優(yōu)化技術(shù)。Python的SciPy庫(kù)中的linprog函數(shù)允許只用幾行代碼就可以解決線性編程問題。雖然還有其他免費(fèi)的優(yōu)化軟件(如GAMS、AMPL、TORA、LINDO),但使用linprog函數(shù)可以節(jié)省大量時(shí)間。