如何使用支持向量機學習非線性數(shù)據(jù)集
支持向量機(SVM)
什么是支持向量機呢?支持向量機是監(jiān)督機器學習模型,可對數(shù)據(jù)進行分類分析。實際上,支持向量機算法是尋找能將實例進行分離的優(yōu)秀超平面的過程。
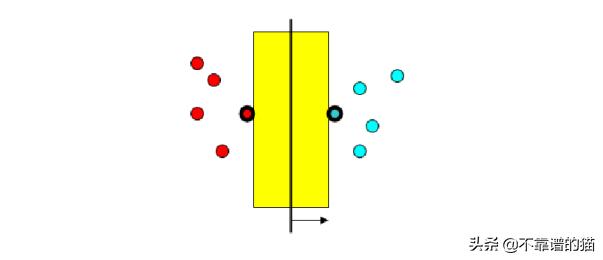
如果數(shù)據(jù)像上面那樣是線性可分離的,那么我們用一個線性分類器就能將兩個類分開。如果我們的數(shù)據(jù)是非線性可分的,我們應該怎么做呢?就像這樣:
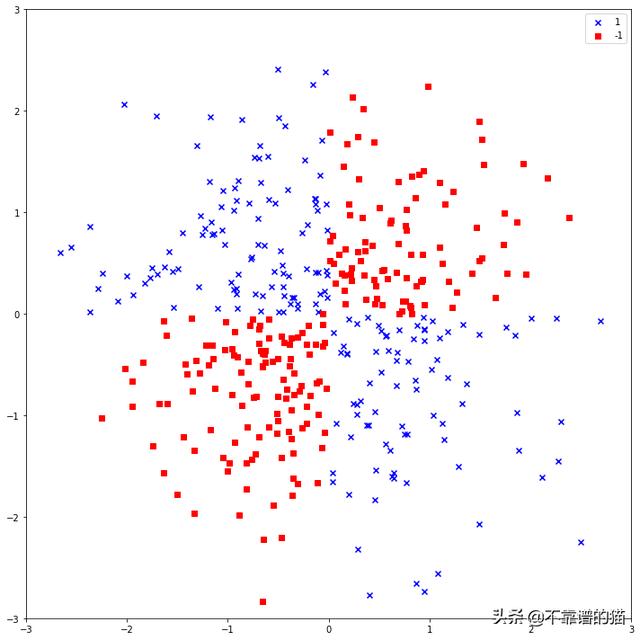
正如我們所看到的,即使來自不同類的數(shù)據(jù)點是可分離的,我們也不能簡單地畫一條直線來進行分類。
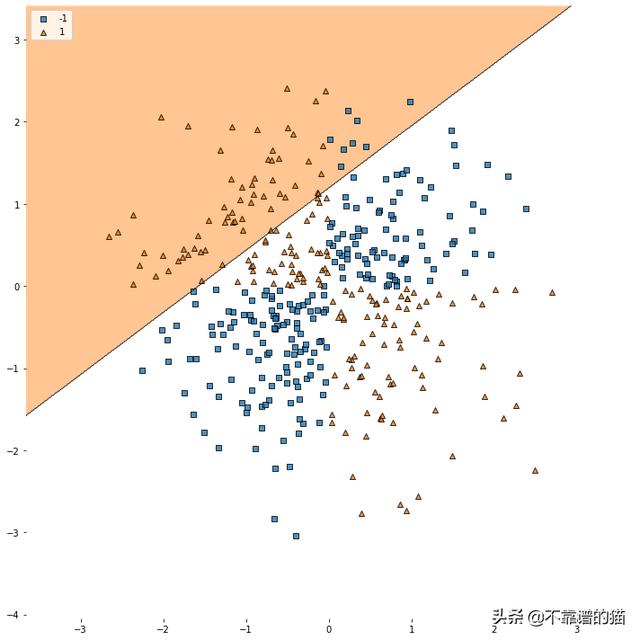
那么我們?nèi)绾问褂弥С窒蛄繖C來擬合非線性機器學習數(shù)據(jù)集呢?
使用SVM進行實驗
創(chuàng)建機器學習數(shù)據(jù)集
首先創(chuàng)建非線性機器學習數(shù)據(jù)集。Python代碼如下:
- # Import packages to visualize the classifer
- from matplotlib.colors import ListedColormap
- import matplotlib.pyplot as plt
- import warnings
- # Import packages to do the classifying
- import numpy as np
- from sklearn.svm import SVC
- # Create Dataset
- np.random.seed(0)
- X_xor = np.random.randn(200, 2)
- y_xor = np.logical_xor(X_xor[:, 0] > 0,
- X_xor[:, 1] > 0)
- y_xor = np.where(y_xor, 1, -1)
- fig = plt.figure(figsize=(10,10))
- plt.scatter(X_xor[y_xor == 1, 0],
- X_xor[y_xor == 1, 1],
- c='b', marker='x',
- label='1')
- plt.scatter(X_xor[y_xor == -1, 0],
- X_xor[y_xor == -1, 1],
- c='r',
- marker='s',
- label='-1')
- plt.xlim([-3, 3])
- plt.ylim([-3, 3])
- plt.legend(loc='best')
- plt.tight_layout()
- plt.show()
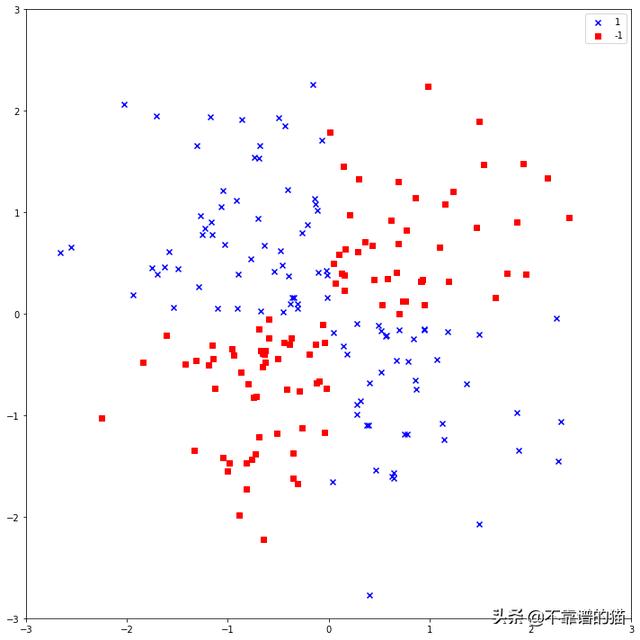
嘗試使用線性支持向量機
我們首先嘗試使用線性支持向量機,Python實現(xiàn)如下:
- # Import packages to do the classifying
- from mlxtend.plotting import plot_decision_regions
- import numpy as np
- from sklearn.svm import SVC
- # Create a SVC classifier using a linear kernel
- svm = SVC(kernel='linear', C=1000, random_state=0)
- # Train the classifier
- svm.fit(X_xor, y_xor)
- # Visualize the decision boundaries
- fig = plt.figure(figsize=(10,10))
- plot_decision_regions(X_xor, y_xor, clf=svm)
- plt.legend(loc='upper left')
- plt.tight_layout()
- plt.show()
C是與錯誤分類相關的成本。C值越高,算法對數(shù)據(jù)集的正確分離就越嚴格。對于線性分類器,我們使用kernel='linear'。
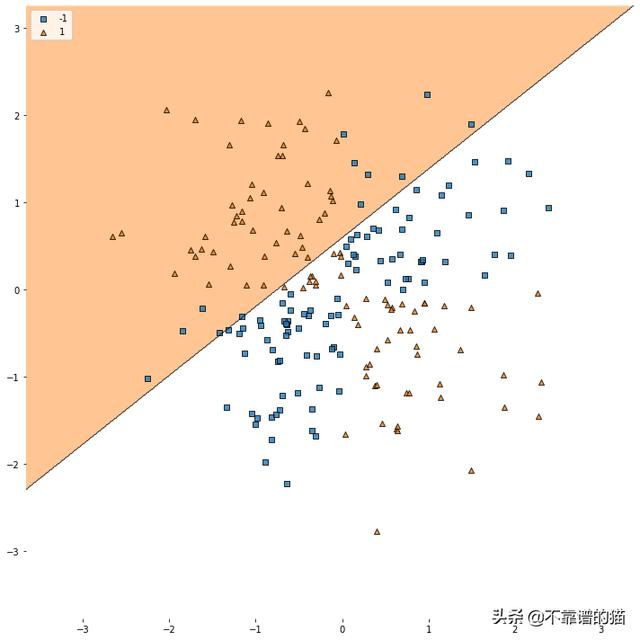
如我們所見,即使我們將成本設置得很高,但這條線也無法很好地分離紅點和藍點。
徑向基函數(shù)核
到目前為止,我們使用的線性分類器為:
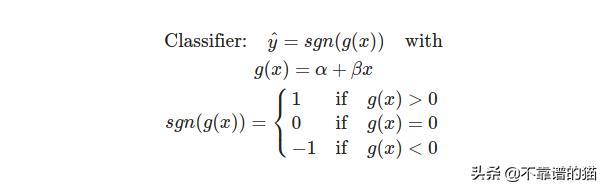
正如我們所看到的,g(x)是一個線性函數(shù)。當g(x) >為0時,預測值為1。當g(x) <0時,預測值為-1。但是由于我們不能使用線性函數(shù)處理像上面這樣的非線性數(shù)據(jù),我們需要將線性函數(shù)轉換成另一個函數(shù)。

這個分類器似乎是我們非線性數(shù)據(jù)的理想選擇。讓我們來看看Python的代碼:
- # Create a SVC classifier using an RBF kernel
- svm = SVC(kernel='rbf', random_state=0, gamma=1/100, C=1)
- # Train the classifier
- svm.fit(X_xor, y_xor)
- # Visualize the decision boundaries
- fig = plt.figure(figsize=(10,10))
- plot_decision_regions(X_xor, y_xor, clf=svm)
- plt.legend(loc='upper left')
- plt.tight_layout()
- plt.show()
gamma是1 / sigma。請記住,sigma是調(diào)節(jié)函數(shù)。因此,gamma值越小,sigma值就越大,分類器對各個點之間的距離就越不敏感。
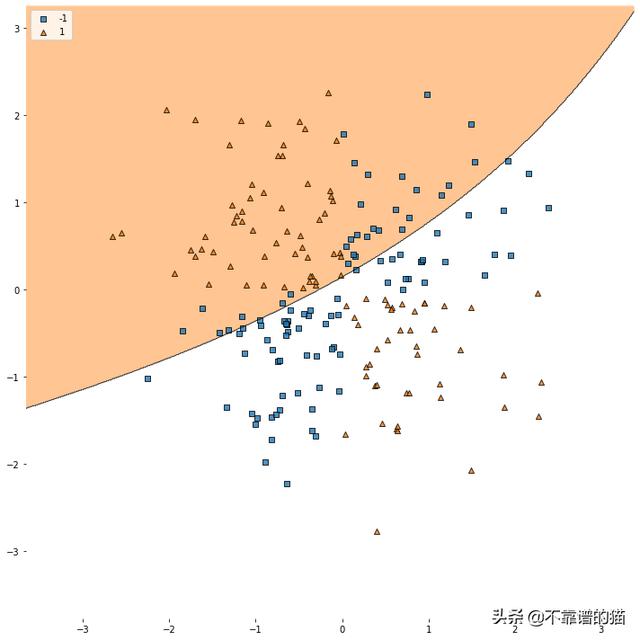
讓我們把伽瑪放大看看會發(fā)生什么
- # Create a SVC classifier using an RBF kernel
- svm = SVC(kernel='rbf', random_state=0, gamma=1, C=1)
- # Train the classifier
- svm.fit(X_xor, y_xor)
- # Visualize the decision boundaries
- fig = plt.figure(figsize=(10,10))
- plot_decision_regions(X_xor, y_xor, clf=svm)
- plt.legend(loc='upper left')
- plt.tight_layout()
- plt.show()
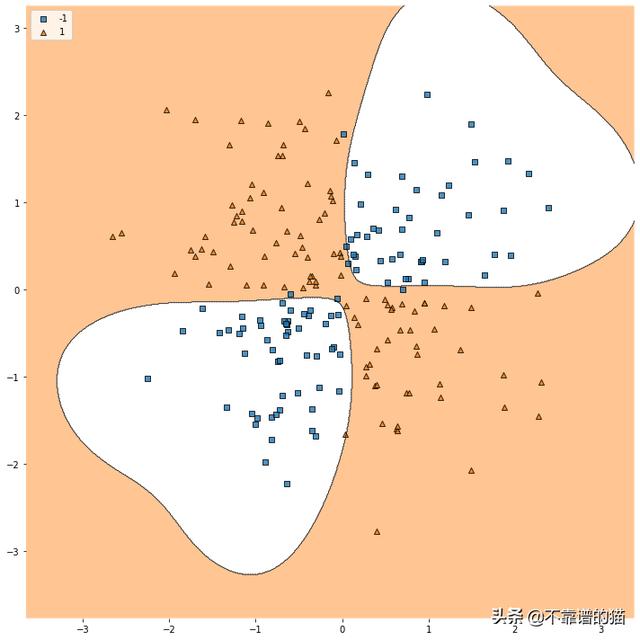
好像將伽瑪值提高100倍可以提高分類器對訓練集的準確性。把伽馬值再乘以10會怎么樣呢?
- # Create a SVC classifier using an RBF kernel
- svm = SVC(kernel='rbf', random_state=0, gamma=10, C=1)
- # Train the classifier
- svm.fit(X_xor, y_xor)
- # Visualize the decision boundaries
- fig = plt.figure(figsize=(10,10))
- plot_decision_regions(X_xor, y_xor, clf=svm)
- plt.legend(loc='upper left')
- plt.tight_layout()
- plt.show()
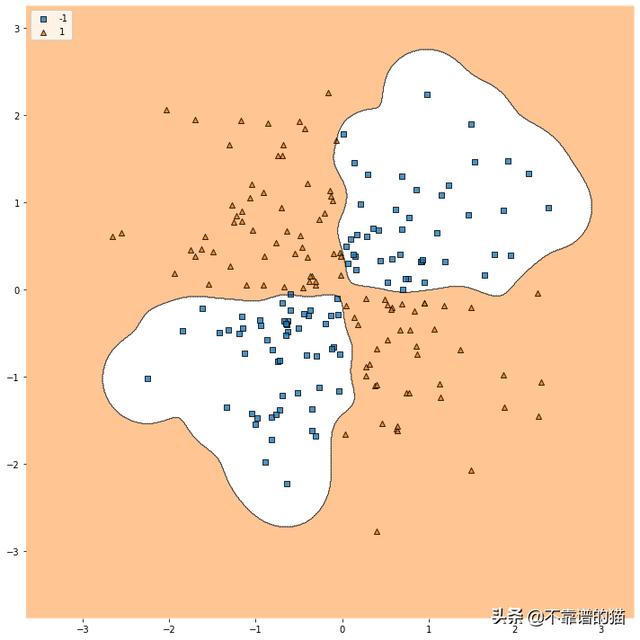
這是否意味著如果我們將伽瑪提高到10000,它將更加準確呢?事實上,如果伽瑪值太大,則分類器最終會對差異不敏感。
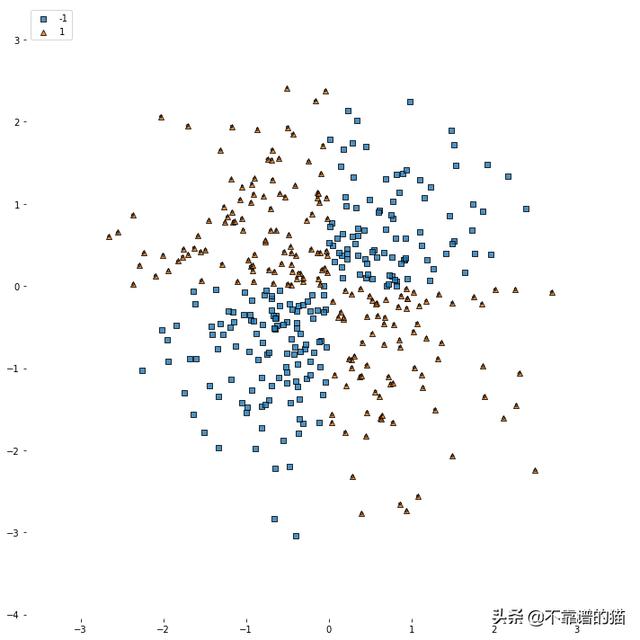
讓我們增加C。C是與整個機器學習數(shù)據(jù)集的錯誤分類相關的成本。換句話說,增加C將增加對整個數(shù)據(jù)集的敏感性,而不僅僅是單個數(shù)據(jù)點。
- from ipywidgets import interact, interactive, fixed, interact_manual
- import ipywidgets as widgets
- warnings.filterwarnings("ignore")
- @interact(x=[1, 10, 1000, 10000, 100000])
- def svc(x=1):
- # Create a SVC classifier using an RBF kernel
- svm = SVC(kernel='rbf', random_state=0, gamma=.01, C=x)
- # Train the classifier
- svm.fit(X_xor, y_xor)
- # Visualize the decision boundaries
- fig = plt.figure(figsize=(10,10))
- plot_decision_regions(X_xor, y_xor, clf=svm)
- plt.legend(loc='upper left')
- plt.tight_layout()
- plt.show()
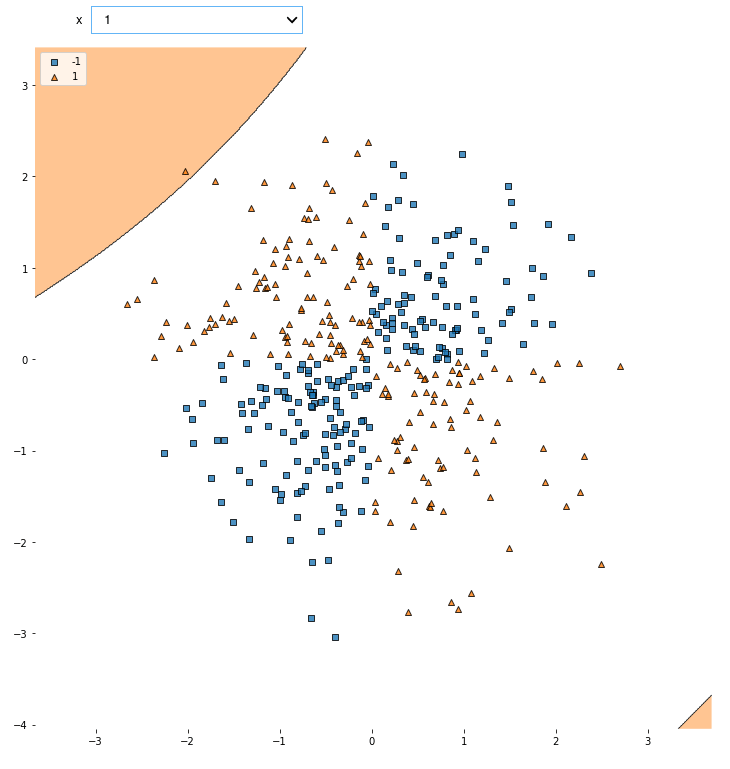
我們已經(jīng)找到了參數(shù),因此我們的SVM分類器可以成功地將兩組點分開。
最后
我希望本文能讓您對SVM分類器是什么以及如何使用它來學習非線機器學習性數(shù)據(jù)集有一個直觀的認識。如果數(shù)據(jù)是高維的,您則無法通過可視化來判斷分類器的性能。好的做法是根據(jù)訓練集進行訓練,并在測試集上使用混淆矩陣或f1-分數(shù)等指標。