RichRAG框架:為用戶提供豐富全面且令人滿意的回答
檢索增強型生成(RAG)使LLMs能夠利用檢索到的可靠信息,從而返回更可靠的響應(yīng)?,F(xiàn)有研究主要關(guān)注需要簡潔明確答案的特定問題,但用戶意圖通常是復(fù)雜和多方面的,需要豐富和全面的答案。
一個多方面查詢需要全面回答的情境示例
為了解決這一重要但未被充分探索的問題,提出了一種名為RichRAG的新型RAG框架:
子方面探索器:識別輸入問題潛在的子方面。
多方面檢索器:構(gòu)建與這些子方面相關(guān)的多樣化外部文檔候選池。
生成式列表智能排序器:關(guān)鍵模塊,為最終生成器提供最有價值的文檔排名列表。
RichRAG的整體框架。在底部描述了排名器的訓(xùn)練階段
1 問題定義
在基本的RAG設(shè)置中,包含知識庫C、固定檢索器R和作為生成器的固定LLM G。對于多方面的問題q,其下屬的各個方面表示為S={s1, ..., sn},這些子方面有相應(yīng)的子回答A={a1, ..., an}。目標(biāo)是使RichRAG生成的回答r不僅與真實回答匹配,而且全面覆蓋各個子回答,確?;卮鸬呢S富性和完整性。
2 子方面探索器
利用LLM構(gòu)建子方面探索器E,預(yù)測輸入查詢的子方面。該模塊輸入提示pse和用戶查詢q,生成一系列子方面。
3 多方面檢索器
根據(jù)查詢的子方面,使用多方面檢索器收集與各種子方面相關(guān)的文檔,構(gòu)建多樣化的候選文檔池。這包括針對每個子方面檢索文檔的過程和合并檢索到的文檔以創(chuàng)建候選池。
4 生成式列表智能排序器
為了從候選池中篩選出最有價值的文檔,設(shè)計了一個基于生成模型的排名模型。該模型輸入用戶查詢、識別的子方面和所有候選項,直接生成頂級文檔ID的排名列表。排名器采用兩階段優(yōu)化:監(jiān)督式微調(diào)和強化學(xué)習(xí)。
4.1 監(jiān)督式微調(diào)
使用貪婪算法構(gòu)建銀牌目標(biāo)排名列表,支持排名器的監(jiān)督式微調(diào)。通過覆蓋效用函數(shù),測量每個剩余文檔的增量方面覆蓋增益。
4.2 強化學(xué)習(xí)
使用強化學(xué)習(xí)策略探索更好的排名可能性,以最終回答的質(zhì)量作為排名列表的獎勵,采用直接偏好優(yōu)化(DPO)算法優(yōu)化排名器,并引入單邊重要性采樣策略(US3)構(gòu)建有價值的訓(xùn)練樣本。
在兩個公開可用的數(shù)據(jù)集上進行的實驗結(jié)果證明,RichRAG框架能夠有效地為用戶提供全面且令人滿意的回答。
所有模型的總體結(jié)果。最佳和次佳結(jié)果分別用粗體和下劃線標(biāo)出
不同子方面數(shù)量的子集實驗,RichRAG框架在所有子集上都優(yōu)于所有基線,框架在多樣化搜索場景中的魯棒性
Prompt模板:標(biāo)注問題方面,并將長答案拆分成相應(yīng)的子答案
Your task is to adjust the results of query-facets mining. The query-facets are extensions of the
original query in various generic perspectives, rather than some specific facts. Given a query that
requires information from multiple query-facets, you should return all query-facets of the query
to fully answer it query. Note that each query has at least two query-facets. I will give you the
long-form answer to the original query to help you explore query-facets based on the perspectives
of its answer. But refrain from using the additional information from the answer to generate the
query-facets. Then you should segment the original long-form answer into several sub-answers
that each are paired with a query-facet. Please return each query-facet of the original query and its
corresponding sub-answers. The query-facets and sub-answers should be one-to-one and returned
in JSON format. You need to follow the following rules:
1. The answers are only used to help you determine the generic direction. You mustn’t generate
query-facets based on the contents of answers and the facets mustn’t contain the answers’
additional information beyond the input query.
2. Sub-answers are constructed by segmenting the original answer, you cannot generate or reorder
the original answer to create sub-answers.
3. The sub-answers should be complete. You must ensure that when the sub-answers are joined
together in order, the complete original answer should be formed.
4. The generated query-facets should be sufficiently generic and contain no specific information
about the sub-answers.
5. **You should ensure that generated query-facets cover all perspectives original answer.**
6. **You should ensure that all sub-answers cover all contents of the original answer.**
7. **The number of query surfaces must range from 2 to 7.**
8. **You should ensure that each query-facet is sufficiently generic and can be easily derived from
the original query.**
9. **You should ensure each query-facet contains no information from the answer.**
10. **You should rewrite or combine the query-facets to be more generic if some query-facets do
not meet the above requirements.**
11. The returned results should be in JSON format and contain the following key: results, which
is a list of JSON data. Each item of results should contain the following keys: query-facet, and
sub-answer.
12. I will give you some demonstrations, you should learn the pattern of them to mine query-facets
and split sub-answers.
**Demonstration**
{demonstrations}
Query: {query}
Answer: {answer}
Results:
對于RAG整個框架的更多技術(shù),PaperAgent團隊RAG專欄進行過詳細的歸納總結(jié):高級RAG之36技(術(shù))。
高級RAG之36技試看私信獲取:RAG專欄
RichRAG: Crafting Rich Responses for Multi-faceted Queries in Retrieval-Augmented Generation
https://arxiv.org/pdf/2406.12566
本文轉(zhuǎn)載自??PaperAgent??
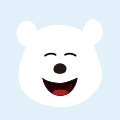