利用LlamaIndex和本地PDF文檔,輕松打造知識(shí)圖譜GraphRAG
檢索增強(qiáng)生成(RAG)技術(shù)通過(guò)引入外部知識(shí)源,增強(qiáng)了大型語(yǔ)言模型的回答準(zhǔn)確性和上下文契合度。盡管RAG在處理復(fù)雜異構(gòu)信息時(shí)可能會(huì)忽略實(shí)體間的結(jié)構(gòu)和聯(lián)系,例如,向量數(shù)據(jù)庫(kù)可能錯(cuò)誤地將“員工”與“雇主”關(guān)聯(lián)得更緊密,而非“信息”。
知識(shí)圖譜的引入有效解決了這一局限。它采用節(jié)點(diǎn)和邊的三元組結(jié)構(gòu),如“雇主 — 提交 — 索賠”,清晰地表達(dá)了實(shí)體間的關(guān)系。這種結(jié)構(gòu)化的方法讓知識(shí)圖譜在處理復(fù)雜數(shù)據(jù)搜索時(shí)更為精確和高效。
1 技術(shù)實(shí)現(xiàn)
1.1 安裝依賴項(xiàng)
!pip install -q pypdf
!pip install -q python-dotenv
!pip install -q pyvis
!pip install -q transformers einops accelerate langchain bitsandbytes sentence_transformers langchain-community langchain-core
!pip install -q llama-index
!pip install -q llama-index-llms-huggingface
!pip install -q llama-index-embeddings-langchain
!pip install -q llama-index-embeddings-huggingface
- LlamaIndex:一個(gè)簡(jiǎn)單、靈活的數(shù)據(jù)框架,用于將自定義數(shù)據(jù)源連接到LLMs
- SimpleDirectoryReader:將本地文件數(shù)據(jù)加載到LlamaIndex的最簡(jiǎn)單方式
- KnowledgeGraphIndex:從非結(jié)構(gòu)化文本自動(dòng)構(gòu)建知識(shí)圖譜
- SimpleGraphStore:簡(jiǎn)單的圖存儲(chǔ)索引
- PyVis:一個(gè)Python庫(kù),用于可視化和構(gòu)建圖網(wǎng)絡(luò)
1.2 啟用診斷日志
日志可提供代碼執(zhí)行情況的寶貴信息。
import os, logging, sys
logging.basicConfig(stream=sys.stdout, level=logging.INFO)
logging.getLogger().addHandler(logging.StreamHandler(stream=sys.stdout))
1.3 連接Huggingface API
請(qǐng)更新至你的Hugging Face推理API端點(diǎn)。
from huggingface_hub import login
os.environ["HF_KEY"] = "Your Hugging Face access token goes here"
login(token=os.environ.get('HF_KEY'),add_to_git_credential=True)
1.4 加載PDF文檔
- Innovate BC Innovator Skills Initiative
- BC Arts Council Application Assistance
from llama_index.core import SimpleDirectoryReader
documents = SimpleDirectoryReader(input_dir="/content/", required_exts=".pdf").load_data()
2 構(gòu)建知識(shí)圖譜索引
2.1 使用HuggingFace創(chuàng)建本地嵌入
HuggingFaceEmbeddings 類是 LangChain 庫(kù)的一部分,它封裝了 Hugging Face 提供的句子轉(zhuǎn)換器模型,用于創(chuàng)建文本嵌入。這個(gè)類支持調(diào)用 Hugging Face 平臺(tái)上所有可用的句子嵌入模型,以執(zhí)行包括語(yǔ)義搜索、文檔聚類和問(wèn)答等任務(wù)。在本次練習(xí)中,采用了 Hugging Face 的 multi-qa-MiniLM-L6-cos-v1 句子轉(zhuǎn)換器模型。
from llama_index.embeddings.huggingface import HuggingFaceEmbedding
EMBEDDING_MODEL_NAME = "sentence-transformers/multi-qa-MiniLM-L6-cos-v1"
embed_model = HuggingFaceEmbedding(model_name=EMBEDDING_MODEL_NAME, embed_batch_size=10)
2.2 從ServiceContext遷移到Settings
LlamaIndex v0.10.0 版本推出了全新的全局 Settings 對(duì)象,用以取代之前的 ServiceContext 配置方式。
這個(gè) Settings 對(duì)象充當(dāng)著全局配置的角色,采用了按需實(shí)例化的機(jī)制。例如,LLM 或嵌入模型等屬性,只有在相關(guān)底層模塊真正需要時(shí),才會(huì)進(jìn)行加載。這樣的設(shè)計(jì)使得資源的使用更加高效,響應(yīng)更為迅速。
from llama_index.core import Settings
Settings.embed_model = embed_model
Settings.chunk_size = 256
Settings.chunk_overlap = 50
文檔在索引時(shí)會(huì)被劃分成有重疊的小塊,這個(gè)過(guò)程稱為“分塊”。通常塊的大小設(shè)為1024,重疊部分為20。鑒于我們的文檔較短,我們調(diào)整塊大小為256,并設(shè)置重疊為50,以優(yōu)化處理。
2.3 定義自定義提示
from llama_index.core import PromptTemplate
system_prompt = """<|SYSTEM|># You are an AI-enabled admin assistant.
Your goal is to answer questions accurately using only the context provided.
"""
# 這將包裝 llama-index 內(nèi)部的默認(rèn)提示
query_wrapper_prompt = PromptTemplate("<|USER|>{query_str}<|ASSISTANT|>")
LLM_MODEL_NAME = "meta-llama/Llama-2-7b-chat-hf"
2.4 設(shè)置LLM
import torch
from llama_index.llms.huggingface import HuggingFaceLLM
llm = HuggingFaceLLM(
context_window=4096,
max_new_tokens=512,
generate_kwargs={"temperature": 0.1, "do_sample": False},
system_prompt=system_prompt,
query_wrapper_prompt=query_wrapper_prompt,
tokenizer_name=LLM_MODEL_NAME,
model_name=LLM_MODEL_NAME,
device_map="auto",
# 如果使用CUDA以減少內(nèi)存使用,請(qǐng)取消注釋此行
model_kwargs={"torch_dtype": torch.float16 , "load_in_8bit":True}
)
Settings.llm = llm
2.5 構(gòu)建知識(shí)圖譜索引
from llama_index.core.storage.storage_context import StorageContext
from llama_index.core import KnowledgeGraphIndex
from llama_index.core.graph_stores import SimpleGraphStore
# 設(shè)置存儲(chǔ)上下文
graph_store = SimpleGraphStore()
storage_context = StorageContext.from_defaults(graph_store=graph_store)
index = KnowledgeGraphIndex.from_documents(documents=documents,
max_triplets_per_chunk=3,
storage_cnotallow=storage_context,
embed_model=embed_model,
include_embeddings=True)
- max_triplets_per_chunk:指的是每個(gè)文本塊中能夠提取的最大三元組數(shù)量。降低這個(gè)數(shù)值可以提升處理效率,因?yàn)樗鼫p少了需要處理的三元組數(shù)量。
- include_embeddings:用于決定索引中是否包含嵌入項(xiàng)。默認(rèn)設(shè)置為False,因?yàn)樯汕度腠?xiàng)在計(jì)算上可能相當(dāng)耗費(fèi)資源。
2.6 可視化知識(shí)圖譜
from pyvis.network import Network
g = index.get_networkx_graph()
net = Network(notebook=True, cdn_resources="in_line", directed=True)
net.from_nx(g)
net.save_graph("rag_graph.html")
from IPython.display import HTML, display
HTML(filename="rag_graph.html")
2.7 查詢
query_engine = index.as_query_engine(llm=llm, similarity_top_k=5)
done = False
while not done:
print("*"*30)
question = input("Enter your question: ")
response = query_engine.query(question)
print(response)
done = input("End the chat? (y/n): ") == "y"
3 結(jié)語(yǔ)
傳統(tǒng)的向量型RAG和圖RAG在數(shù)據(jù)存儲(chǔ)與展示上各有側(cè)重。向量數(shù)據(jù)庫(kù)擅長(zhǎng)通過(guò)相似性來(lái)比較對(duì)象,利用數(shù)值來(lái)衡量對(duì)象間的距離。而知識(shí)圖譜則專注于揭示復(fù)雜的聯(lián)系和對(duì)象間的依賴性,通過(guò)節(jié)點(diǎn)和邊進(jìn)行深入的語(yǔ)義分析和邏輯推理。這兩種方法各自適用于不同的應(yīng)用場(chǎng)景。
本文轉(zhuǎn)載自 ??AI科技論談??,作者: AI科技論談
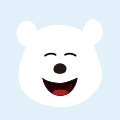