RAG高級(jí)優(yōu)化:基于問題生成的文檔檢索增強(qiáng) 原創(chuàng)
我們將在本文中介紹一種文本增強(qiáng)技術(shù),該技術(shù)利用額外的問題生成來改進(jìn)矢量數(shù)據(jù)庫(kù)中的文檔檢索。通過生成和合并與每個(gè)文本片段相關(guān)的問題,增強(qiáng)系統(tǒng)標(biāo)準(zhǔn)檢索過程,從而增加了找到相關(guān)文檔的可能性,這些文檔可以用作生成式問答的上下文。
實(shí)現(xiàn)步驟
通過用相關(guān)問題豐富文本片段,我們的目標(biāo)是顯著提高識(shí)別文檔中包含用戶查詢答案的最相關(guān)部分的準(zhǔn)確性。具體的方案實(shí)現(xiàn)一般包含以下步驟:
- 文檔解析和文本分塊:處理PDF文檔并將其劃分為可管理的文本片段。
- 問題增強(qiáng):使用語(yǔ)言模型在文檔和片段級(jí)別生成相關(guān)問題。
- 矢量存儲(chǔ)創(chuàng)建:使用??向量模型?計(jì)算文檔的嵌入,并創(chuàng)建FAISS矢量存儲(chǔ)。
- 檢索和答案生成:使用FAISS查找最相關(guān)的文檔,并根據(jù)提供的上下文生成答案。
我們可以通過設(shè)置,指定在文檔級(jí)或片段級(jí)進(jìn)行問題增強(qiáng)。
class QuestionGeneration(Enum):
"""
Enum class to specify the level of question generation for document processing.
Attributes:
DOCUMENT_LEVEL (int): Represents question generation at the entire document level.
FRAGMENT_LEVEL (int): Represents question generation at the individual text fragment level.
"""
DOCUMENT_LEVEL = 1
FRAGMENT_LEVEL = 2
方案實(shí)現(xiàn)
問題生成
def generate_questions(text: str) -> List[str]:
"""
Generates a list of questions based on the provided text using OpenAI.
Args:
text (str): The context data from which questions are generated.
Returns:
List[str]: A list of unique, filtered questions.
"""
llm = ChatOpenAI(model="gpt-4o-mini", temperature=0)
prompt = PromptTemplate(
input_variables=["context", "num_questions"],
template="Using the context data: {context}\n\nGenerate a list of at least {num_questions} "
"possible questions that can be asked about this context. Ensure the questions are "
"directly answerable within the context and do not include any answers or headers. "
"Separate the questions with a new line character."
)
chain = prompt | llm.with_structured_output(QuestionList)
input_data = {"context": text, "num_questions": QUESTIONS_PER_DOCUMENT}
result = chain.invoke(input_data)
# Extract the list of questions from the QuestionList object
questions = result.question_list
filtered_questions = clean_and_filter_questions(questions)
return list(set(filtered_questions))
處理主流程
def process_documents(content: str, embedding_model: OpenAIEmbeddings):
"""
Process the document content, split it into fragments, generate questions,
create a FAISS vector store, and return a retriever.
Args:
content (str): The content of the document to process.
embedding_model (OpenAIEmbeddings): The embedding model to use for vectorization.
Returns:
VectorStoreRetriever: A retriever for the most relevant FAISS document.
"""
# Split the whole text content into text documents
text_documents = split_document(content, DOCUMENT_MAX_TOKENS, DOCUMENT_OVERLAP_TOKENS)
print(f'Text content split into: {len(text_documents)} documents')
documents = []
counter = 0
for i, text_document in enumerate(text_documents):
text_fragments = split_document(text_document, FRAGMENT_MAX_TOKENS, FRAGMENT_OVERLAP_TOKENS)
print(f'Text document {i} - split into: {len(text_fragments)} fragments')
for j, text_fragment in enumerate(text_fragments):
documents.append(Document(
page_cnotallow=text_fragment,
metadata={"type": "ORIGINAL", "index": counter, "text": text_document}
))
counter += 1
if QUESTION_GENERATION == QuestionGeneration.FRAGMENT_LEVEL:
questions = generate_questions(text_fragment)
documents.extend([
Document(page_cnotallow=question, metadata={"type": "AUGMENTED", "index": counter + idx, "text": text_document})
for idx, question in enumerate(questions)
])
counter += len(questions)
print(f'Text document {i} Text fragment {j} - generated: {len(questions)} questions')
if QUESTION_GENERATION == QuestionGeneration.DOCUMENT_LEVEL:
questions = generate_questions(text_document)
documents.extend([
Document(page_cnotallow=question, metadata={"type": "AUGMENTED", "index": counter + idx, "text": text_document})
for idx, question in enumerate(questions)
])
counter += len(questions)
print(f'Text document {i} - generated: {len(questions)} questions')
for document in documents:
print_document("Dataset", document)
print(f'Creating store, calculating embeddings for {len(documents)} FAISS documents')
vectorstore = FAISS.from_documents(documents, embedding_model)
print("Creating retriever returning the most relevant FAISS document")
return vectorstore.as_retriever(search_kwargs={"k": 1})
該技術(shù)為提高基于向量的文檔檢索系統(tǒng)的信息檢索質(zhì)量提供了一種方法。此實(shí)現(xiàn)使用了大模型的API,這可能會(huì)根據(jù)使用情況產(chǎn)生成本。
本文轉(zhuǎn)載自公眾號(hào)哎呀AIYA
原文鏈接:??https://mp.weixin.qq.com/s/bjI02uOeAGXSelCApb0yOQ??
?著作權(quán)歸作者所有,如需轉(zhuǎn)載,請(qǐng)注明出處,否則將追究法律責(zé)任
標(biāo)簽
已于2024-9-14 14:18:55修改
贊
收藏
回復(fù)
分享
微博
QQ
微信
舉報(bào)
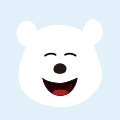
回復(fù)
相關(guān)推薦