OpenAI文本嵌入模型入門指南 原創(chuàng)
這篇綜合指南介紹了如何使用OpenAI文本嵌入模型在GenAI應(yīng)用程序中嵌入創(chuàng)建和語義搜索。
向量嵌入在AI中至關(guān)重要,它可以將復(fù)雜的非結(jié)構(gòu)化數(shù)據(jù)轉(zhuǎn)換成機器可以處理的數(shù)值向量。這些嵌入捕獲數(shù)據(jù)中的語義和關(guān)系,從而實現(xiàn)更有效的分析和內(nèi)容生成。
ChatGPT的創(chuàng)建者OpenAI提供了各種嵌入模型,這些模型提供高質(zhì)量的向量表示,可用于包括語義搜索、聚類和異常檢測在內(nèi)的各種應(yīng)用。這篇指南將探討如何利用OpenAI的文本嵌入模型來構(gòu)建響應(yīng)迅速的智能AI系統(tǒng)。
什么是向量嵌入和嵌入模型?
在我們深入討論之前,不妨先闡述幾個術(shù)語。首先,什么是向量嵌入?向量嵌入是許多AI概念的基礎(chǔ)。它是數(shù)據(jù)的數(shù)值表示,特別是非結(jié)構(gòu)化數(shù)據(jù),比如文本、視頻、音頻、圖片及其他數(shù)字媒體。它捕獲數(shù)據(jù)中的語義和關(guān)系,并為存儲系統(tǒng)和AI模型提供一種高效的方式來解讀、處理、存儲和檢索復(fù)雜的高維非結(jié)構(gòu)化數(shù)據(jù)。
所以,如果嵌入是數(shù)據(jù)的數(shù)值表示,那么如何將數(shù)據(jù)轉(zhuǎn)換成向量嵌入?這時候嵌入模型就有了用武之地。
嵌入模型是一種將非結(jié)構(gòu)化數(shù)據(jù)轉(zhuǎn)換成向量嵌入的專用算法。它旨在學(xué)習(xí)數(shù)據(jù)中的模式和關(guān)系,然后在高維空間中表示它們。關(guān)鍵思想是,相似的數(shù)據(jù)片段具有相似的向量表示,并且在高維空間中彼此更接近,從而允許AI模型更有效地處理和分析數(shù)據(jù)。
比如在自然語言處理(NLP)背景下,嵌入模型可能在學(xué)習(xí)后明白單詞“king”和“queen”是相關(guān)的,應(yīng)該在向量空間中彼此靠近,而像“banana”這樣的單詞將被放在更遠的位置。向量空間中的這種鄰近反映了單詞之間的語義關(guān)系。
嵌入模型和向量嵌入的一個常見用途在于檢索增強生成(RAG)系統(tǒng)。RAG系統(tǒng)不是僅僅依賴大語言模型(LLM)中的預(yù)訓(xùn)練知識,而是在生成輸出之前為LLM提供額外的上下文信息。這些額外的數(shù)據(jù)使用嵌入模型轉(zhuǎn)換成向量嵌入,然后存儲在像Milvus這樣的向量數(shù)據(jù)庫中。對于需要詳細的、基于事實的查詢響應(yīng)的組織和開發(fā)人員來說,RAG是理想的選擇,使得它在各個行業(yè)部門都很有價值。
OpenAI文本嵌入模型
ChatGPT背后的OpenAI公司提供了各種嵌入模型,它們非常適合處理語義搜索、聚類、推薦系統(tǒng)、異常檢測、多樣性測量和分類等任務(wù)。
鑒于OpenAI的受歡迎程度,許多開發(fā)人員可能會使用它的模型來嘗試RAG概念。雖然這些概念一般適用于嵌入模型,還是不妨關(guān)注OpenAI具體提供了什么。
在談?wù)揘LP時,一些OpenAI嵌入模型特別重要。
- text-embedding-ada- 002
- text-embedding-3-small
- text-embedding-3-large
下表提供了這些模型之間的直接比較。
模型? | 描述? | 輸出維度? | 最大輸入? | 價格? |
text- embedding-3- large | 功能最強大的嵌入模型, 同時適用于英文任務(wù)和 非英文任務(wù)。 | 3072 | 8.191 | 0.13美元/100萬 個token |
text- embedding-3- small | 比第二代ada嵌入模型 提高了性能。 | 1536 | 8.191 | 0.10美元/100萬 個token |
text- embedding- ada - 002 | 功能最強大的第二代嵌入 模型,取代16個第一代 模型。 | 1536 | 8.191 | 0.02美元/100萬 個token |
選擇合適的模型
與所有事情一樣,選擇模型需要權(quán)衡利弊。在全身心投入其中一個模型之前,確保你清楚地了解自己想要做什么、有哪些可用的資源以及期望從生成的輸出中獲得哪種程度的準確性。使用RAG系統(tǒng),你可能會權(quán)衡計算資源與查詢響應(yīng)的速度和準確性。
- text- embeddings -3-large:當(dāng)準確性和嵌入豐富度很重要時,這可能是首選的模型。它使用最多的CPU和內(nèi)存資源(價格更昂貴),需要最長的時間來生成輸出,但輸出將是高質(zhì)量的。典型的用例包括研究、高風(fēng)險應(yīng)用或處理非常復(fù)雜的文本。
- text-embedding-3-small:如果你更關(guān)心速度和效率,而不是獲得絕對最好的結(jié)果,該模型的資源密集程度較低,從而降低了成本,并縮短了響應(yīng)時間。典型的用例包括實時應(yīng)用或資源有限的情形。
- text-embedding-ada-002:雖然其他兩個模型是最新版本,但這是在OpenAI引入之前的主要模型。這種多功能模型在兩個極端之間提供了很好的中間地帶,提供了可靠的性能和合理的效率。
如何用OpenAI生成向量嵌入?
不妨逐步看看如何使用這每一種嵌入模型生成向量嵌入。無論選擇哪種模型,你都需要具備幾個要素才能入手,包括向量數(shù)據(jù)庫。
PyMilvus是用于Milvus的Python軟件開發(fā)工具包(SDK),在這種環(huán)境下很方便,因為它與所有這些OpenAI模型無縫集成。OpenAI Python庫是另一個選擇,它是OpenAI提供的SDK。
為了本教程,我將使用PyMilvus生成向量嵌入,并將它們存儲在Zilliz Cloud中,以便進行簡單的語義搜索。
Zilliz Cloud上手起來很簡單:
- 注冊一個免費的Zilliz Cloud帳戶。
- 設(shè)置無服務(wù)器集群,并獲取公共端點和API密鑰。
- 創(chuàng)建一個向量集合,并插入你的向量嵌入。
- 對存儲的嵌入進行語義搜索。
好了,現(xiàn)在我將解釋如何為上面討論的這三個模型生成向量嵌入。
text-embedding-ada-002text-embedding-ada-002
使用text-embedding-ada-002生成向量嵌入,并將其存儲在Zilliz Cloud中進行語義搜索:
from pymilvus.model.dense import OpenAIEmbeddingFunction
from pymilvus import MilvusClient
OPENAI_API_KEY = "your-openai-api-key"
ef = OpenAIEmbeddingFunction("text-embedding-ada-002", api_key=OPENAI_API_KEY)
docs = [
"Artificial intelligence was founded as an academic discipline in 1956.",
"Alan Turing was the first person to conduct substantial research in AI.",
"Born in Maida Vale, London, Turing was raised in southern England."
]
# Generate embeddings for documents
docs_embeddings = ef(docs)
queries = ["When was artificial intelligence founded",
"Where was Alan Turing born?"]
# Generate embeddings for queries
query_embeddings = ef(queries)
# Connect to Zilliz Cloud with Public Endpoint and API Key
client = MilvusClient(
uri=ZILLIZ_PUBLIC_ENDPOINT,
token=ZILLIZ_API_KEY)
COLLECTION = "documents"
if client.has_collection(collection_name=COLLECTION):
client.drop_collection(collection_name=COLLECTION)
client.create_collection(
collection_name=COLLECTION,
dimension=ef.dim,
auto_id=True)
for doc, embedding in zip(docs, docs_embeddings):
client.insert(COLLECTION, {"text": doc, "vector": embedding})
results = client.search(
collection_name=COLLECTION,
data=query_embeddings,
consistency_level="Strong",
output_fields=["text"])
text-embedding-3-small
使用text-embedding-3-small生成向量嵌入,并將其存儲在Zilliz Cloud中進行語義搜索:
from pymilvus import model, MilvusClient
OPENAI_API_KEY = "your-openai-api-key"
ef = model.dense.OpenAIEmbeddingFunction(
model_name="text-embedding-3-small",
api_key=OPENAI_API_KEY,
)
# Generate embeddings for documents
docs = [
"Artificial intelligence was founded as an academic discipline in 1956.",
"Alan Turing was the first person to conduct substantial research in AI.",
"Born in Maida Vale, London, Turing was raised in southern England."
]
docs_embeddings = ef.encode_documents(docs)
# Generate embeddings for queries
queries = ["When was artificial intelligence founded",
"Where was Alan Turing born?"]
query_embeddings = ef.encode_queries(queries)
# Connect to Zilliz Cloud with Public Endpoint and API Key
client = MilvusClient(
uri=ZILLIZ_PUBLIC_ENDPOINT,
token=ZILLIZ_API_KEY)
COLLECTION = "documents"
if client.has_collection(collection_name=COLLECTION):
client.drop_collection(collection_name=COLLECTION)
client.create_collection(
collection_name=COLLECTION,
dimension=ef.dim,
auto_id=True)
for doc, embedding in zip(docs, docs_embeddings):
client.insert(COLLECTION, {"text": doc, "vector": embedding})
results = client.search(
collection_name=COLLECTION,
data=query_embeddings,
consistency_level="Strong",
output_fields=["text"])
text-embedding-3-large
使用text-embedding-3-large生成向量嵌入,并將其存儲在Zilliz Cloud中進行語義搜索:
from pymilvus.model.dense import OpenAIEmbeddingFunction
from pymilvus import MilvusClient
OPENAI_API_KEY = "your-openai-api-key"
ef = OpenAIEmbeddingFunction("text-embedding-3-large", api_key=OPENAI_API_KEY)
docs = [
"Artificial intelligence was founded as an academic discipline in 1956.",
"Alan Turing was the first person to conduct substantial research in AI.",
"Born in Maida Vale, London, Turing was raised in southern England."
]
# Generate embeddings for documents
docs_embeddings = ef(docs)
queries = ["When was artificial intelligence founded",
"Where was Alan Turing born?"]
# Generate embeddings for queries
query_embeddings = ef(queries)
# Connect to Zilliz Cloud with Public Endpoint and API Key
client = MilvusClient(
uri=ZILLIZ_PUBLIC_ENDPOINT,
token=ZILLIZ_API_KEY)
COLLECTION = "documents"
if client.has_collection(collection_name=COLLECTION):
client.drop_collection(collection_name=COLLECTION)
client.create_collection(
collection_name=COLLECTION,
dimension=ef.dim,
auto_id=True)
for doc, embedding in zip(docs, docs_embeddings):
client.insert(COLLECTION, {"text": doc, "vector": embedding})
results = client.search(
collection_name=COLLECTION,
data=query_embeddings,
consistency_level="Strong",
output_fields=["text"])
結(jié)論
雖然本教程只是觸及表面,但這些腳本足以讓你開始上手向量嵌入。值得一提的是,這些絕不是唯一可用的模型。這份全面的??AI模型列表??都與Milvus協(xié)同工作。不管你的AI用例是什么,你可能都會找到一個可以滿足需求的模型。
如果想進一步了解Milvus、Zilliz Cloud、RAG系統(tǒng)和向量數(shù)據(jù)庫等方面,敬請訪問Zilliz.com。
原文標(biāo)題:Beginner’s Guide to OpenAI Text Embedding Models,作者:Jason Myers
鏈接:???https://thenewstack.io/beginners-guide-to-openai-text-embedding-models/??
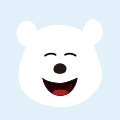