優(yōu)化文本嵌入,大幅提升RAG檢索速度
1 簡介
文本嵌入技術(shù)能夠?qū)⑽淖中畔⑥D(zhuǎn)換成高維向量表示的數(shù)字,提供了一種理解和處理文本數(shù)據(jù)的新方式,幫助我們更好地理解和處理文本數(shù)據(jù)。
這些向量,也就是數(shù)字?jǐn)?shù)組,能夠捕捉文本的深層特征,進(jìn)而支持多種應(yīng)用。比如理解語義、進(jìn)行文本分類、聚類、信息檢索,甚至優(yōu)化搜索結(jié)果排序等。
傳統(tǒng)上,嵌入向量的維度是固定的,通常取2的冪次方,大小介于64到4096之間。
現(xiàn)在,有了套娃嵌入技術(shù),我們可以根據(jù)不同的應(yīng)用需求,靈活調(diào)整嵌入向量的維度。這樣做的好處是顯而易見的:不僅能夠減少存儲需求,降低成本,還能大幅提升檢索效率。
2 文本嵌入
從輸入字符串到句子嵌入
我們先定義一個詞匯表,這個表把所有可能輸入的字符,包括字母、特殊符號、短詞和子詞,都映射到整數(shù)值。比如:
{
"a": 1,
"b": 2,
"c": 3,
...
"z": 26,
"the": 27,
" ": 28
}
經(jīng)過標(biāo)記化處理后,我們可以將令牌(token)列表輸入到編碼器模型中。這個模型經(jīng)過大量數(shù)據(jù)的訓(xùn)練,能夠?qū)⒚總€令牌轉(zhuǎn)換為高維數(shù)值向量嵌入。
例如,OpenAI的text-embedding-3-large模型的嵌入向量輸出維度為3072。
如果想要獲得單個句子嵌入,我們需要從多個令牌嵌入中提取信息。常見的做法是,對所有令牌嵌入求平均值。
3 套娃嵌入(Matryoshka Representation Learning)
套娃嵌入(Matryoshka Representation Learning)是一種先進(jìn)的文本表示技術(shù),由華盛頓大學(xué)、谷歌研究院和哈佛大學(xué)的學(xué)者們在2022年發(fā)表的論文《Matryoshka Representation Learning》中首次提出。
套娃嵌入技術(shù)能夠在單一的嵌入向量中嵌入多個層次的信息。
打個比方,它不是只訓(xùn)練一個單一維度為1024的嵌入向量,而是同時優(yōu)化一組不同大小的維度,如1024、512、256、128、64等。
這樣的設(shè)計讓嵌入向量像套娃一樣,外層包含著較為概括的信息,而內(nèi)層則逐漸包含更細(xì)致的信息。這種結(jié)構(gòu)讓我們能夠在幾乎不影響性能的情況下,根據(jù)實際需求來調(diào)整嵌入向量的長度,從而更好地適應(yīng)各種不同的應(yīng)用環(huán)境。
4 套娃嵌入的重要性
假設(shè)我們要在向量數(shù)據(jù)庫中存儲一大批文本嵌入向量。每個嵌入有 d 個維度。每個維度都是一個32位的浮點數(shù)。這樣算下來,存儲空間就需要n * d * 4 個字節(jié)。
如果我們想要計算這些向量的相似性,如點積或余弦相似性(只是歸一化的點積),維度 d 越高,需要做的數(shù)學(xué)計算量就越多。
點積公式
有了MRL技術(shù),如果我們更看重節(jié)省內(nèi)存和提高處理速度,從而減少成本,那我們可能只取前64個維度來用。如果我們追求最佳的性能,那就用上所有的維度。當(dāng)然,也可以選擇一個折中的維度數(shù)。
總的來說,MRL技術(shù)讓LLM用戶能夠在嵌入向量的存儲成本和性能之間找到一個平衡點。
5 Nomic AI的MRL應(yīng)用
Nomic的套娃文本嵌入模型nomic-embed-text-v1.5?是使用 matryoshka_dims = [768,512,256,128,64] 訓(xùn)練的。該模型在Hugging Face上公開可用。
這個編碼器模型還支持多種前綴,比如[search_query, search_document, classification, clustering],這意味著它能針對搜索查詢、搜索文檔、文本分類和聚類等特定任務(wù),提供更為精準(zhǔn)的嵌入結(jié)果。
以下是nomic-embed-text-v1.5在大規(guī)模文本嵌入基準(zhǔn)(MTEB)上的表現(xiàn):
讓我們使用PyTorch和Sentence Transformers庫在Python中實現(xiàn)該模型:
!pip install torch sentence_transformers einops
import torch
from sentence_transformers import SentenceTransformer
model = SentenceTransformer(
"nomic-ai/nomic-embed-text-v1.5",
device=device,
trust_remote_code=True,
prompts={
"search_query": "search_query: ",
"search_document": "search_document: ",
"classification": "classification: ",
"clustering": "clustering: ",
},
)
def embed_sentences(
model: SentenceTransformer,
sentences: list[str],
prompt_name: str,
matryoshka_dim: int,
device: str,
):
assert matryoshka_dim <= 768, "maximum dimension for nomic-embed-text-v1.5 is 768"
embeddings = model.encode(
sentences, prompt_name=prompt_name, device=device, convert_to_tensor=True
)
embeddings = torch.nn.functional.layer_norm(
embeddings, normalized_shape=(embeddings.shape[1],)
)
embeddings = embeddings[:, :matryoshka_dim]
embeddings = torch.nn.functional.normalize(embeddings, p=2, dim=1)
return embeddings.cpu()
使用 matryoshka_dim 參數(shù),可以將原本768維的嵌入向量進(jìn)行截斷,然后歸一化新的嵌入向量。
現(xiàn)在,可以設(shè)置我們期望的維度,對維基百科上的一些文本內(nèi)容以及相關(guān)問題進(jìn)行編碼,以供檢索增強(qiáng)生成(RAG)的應(yīng)用場景使用:
matryoshka_dim = 64
wikipedia_texts = [
"The dog (Canis familiaris or Canis lupus familiaris) is a domesticated descendant of the wolf.",
"Albert Einstein was born in Ulm in the Kingdom of Württemberg in the German Empire, on 14 March 1879.",
"Einstein excelled at physics and mathematics from an early age, and soon acquired the mathematical expertise normally only found in a child several years his senior.",
"Werner Karl Heisenberg was a German theoretical physicist, one of the main pioneers of the theory of quantum mechanics, and a principal scientist in the Nazi nuclear weapons program during World War II.",
"Steven Paul Jobs (February 24, 1955 - October 5, 2011) was an American businessman, inventor, and investor best known for co-founding the technology giant Apple Inc.",
"The cat (Felis catus), commonly referred to as the domestic cat or house cat, is the only domesticated species in the family Felidae.",
]
question = ["Where was Albert Einstein born?"]
question_embedding = embed_sentences(
model,
sentences=question,
prompt_name="search_query",
matryoshka_dim=matryoshka_dim,
device=device,
)
document_embeddings = embed_sentences(
model,
sentences=wikipedia_texts,
prompt_name="search_document",
matryoshka_dim=matryoshka_dim,
device=device,
)
print(f"document_embeddings.shape: {document_embeddings.shape}")
print(f"question_embedding.shape: {question_embedding.shape}")
>> document_embeddings.shape: torch.Size([6, 64])
>> question_embedding.shape: torch.Size([1, 64])
我們可以用散點圖可視化套娃文本嵌入的前兩個維度。不過,需要注意的是,這個嵌入模型并沒有專門針對二維展示進(jìn)行優(yōu)化。
散點圖展示了維基百科文本和相關(guān)問題的套娃嵌入結(jié)果
接下來,將我們的文檔嵌入存儲在向量數(shù)據(jù)庫中。這里使用的是Faiss。Faiss是Meta Research的開源庫,用于高效相似性搜索和密集向量的聚類。
!pip install faiss-cpu
import faiss
index = faiss.IndexFlatIP(matryoshka_dim)
index.add(document_embeddings)
通過“精確搜索內(nèi)積”的方法,我們構(gòu)建了一個名為IndexFlatIP的向量數(shù)據(jù)庫,它使用的是點積相似性度量。因為我們使用的嵌入向量已經(jīng)過歸一化處理,所以點積和余弦相似性在這種情況下是等價的。
index 現(xiàn)在是一個包含六個文本嵌入的向量數(shù)據(jù)庫:
print(index.ntotal)
>> 6
搜索與我們的問題最相似的嵌入,并檢索前k個結(jié)果:
distances, indices = index.search(question_embedding, k=6)
print(indices)
print(distances)
>> [[1 2 3 4 0 5]]
>> [[0.9633528 0.729192 0.63353264 0.62068397 0.512541 0.43155164]]
我們最相似的文本在數(shù)據(jù)庫中的索引是1,相似性得分為0.96(最高是1.0)。
# results with d=64
print(question)
print(wikipedia_texts[1])
>> ['Where was Albert Einstein born?']
>> 'Albert Einstein was born in Ulm in the Kingdom of Württemberg in the German Empire, on 14 March 1879.'
這里也用matryoshka_dim=768重新運行了代碼,得到了類似的結(jié)果。然而,更高的維度需要更多的內(nèi)存和更多的計算。
# results with d=768
print(indices)
print(distances)
>> [[1 2 4 3 0 5]]
>> [[0.92466116 0.645744 0.54405797 0.54004824 0.39331824 0.37972206]]
6 MRL & 量化
如果我們想要進(jìn)一步壓縮我們的嵌入,可以使用MRL和二進(jìn)制向量量化。二進(jìn)制量化將嵌入向量中所有大于零的數(shù)字轉(zhuǎn)換為一,其余的轉(zhuǎn)換為零。
從完整的嵌入向量到小巧的二進(jìn)制版本
使用二進(jìn)制量化,一個維度為 d 的嵌入向量只需要 d / 8? 字節(jié)的內(nèi)存,這比32位浮點數(shù)的 d * 4 字節(jié)減少了32倍。然而,這種減少是以性能為代價的。
7 結(jié)語
在訓(xùn)練過程中,嵌入模型采用了套娃損失函數(shù),以優(yōu)化多個嵌入維度。
通過套娃表示學(xué)習(xí),LLM用戶可以在減少文本嵌入大小和接受輕微性能損失之間進(jìn)行權(quán)衡。
較小的嵌入向量占用的內(nèi)存更少,計算量也更小,長期來看有助于節(jié)省成本。同時,它們的計算速度也更快,因此具有更高的檢索速度,這對于像RAG這樣的應(yīng)用程序來說尤其重要。
本文轉(zhuǎn)載自 ??AI科技論談??,作者: AI科技論談
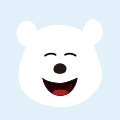