Emu3:開啟多模態(tài)人工智能新紀(jì)元 —— 視頻、圖像、文本三合一模型
隨著人工智能技術(shù)的不斷進(jìn)步,人們對(duì)能夠處理多種模態(tài)數(shù)據(jù)的模型需求日益增長(zhǎng)。圖像、文本和視頻作為三種主要的信息載體,各自具有獨(dú)特的優(yōu)勢(shì)和特點(diǎn)。然而,傳統(tǒng)的人工智能模型往往只能處理單一類型的數(shù)據(jù),無法充分發(fā)揮多模態(tài)數(shù)據(jù)的潛力。為了打破這一局限,北京智源人工智能研究院的科研團(tuán)隊(duì)經(jīng)過不懈努力,成功研發(fā)出了 Emu3 原生多模態(tài)世界模型。
一、Emu3 的技術(shù)特點(diǎn)
1. 多模態(tài)統(tǒng)一理解與生成
Emu3 實(shí)現(xiàn)了視頻、圖像、文本三種模態(tài)的統(tǒng)一理解與生成。這意味著它可以同時(shí)處理和關(guān)聯(lián)不同模態(tài)的數(shù)據(jù),從而更好地理解和模擬物理世界。例如,當(dāng)給定一段文本描述時(shí),Emu3 可以生成相應(yīng)的圖像或視頻;反之,當(dāng)輸入一張圖像或一段視頻時(shí),它也能夠生成準(zhǔn)確的文本描述。這種多模態(tài)的交互能力為人工智能在各個(gè)領(lǐng)域的應(yīng)用提供了更廣闊的空間。
2. 創(chuàng)新的技術(shù)架構(gòu)
Emu3 只基于下一個(gè) token 預(yù)測(cè),無需擴(kuò)散模型或組合式方法。它將圖像、文本和視頻編碼為一個(gè)離散空間,在多模態(tài)混合序列上從頭開始聯(lián)合訓(xùn)練一個(gè) transformer 模型。這種創(chuàng)新的架構(gòu)設(shè)計(jì)不僅簡(jiǎn)化了模型的復(fù)雜性,還提高了模型的靈活性和效率。同時(shí),它也為多模態(tài)人工智能的發(fā)展提供了新的思路和方法。
3. 卓越的性能表現(xiàn)
在圖像生成、視覺語言理解、視頻生成任務(wù)中,Emu3 的表現(xiàn)超過了 SDXL、LLava - 1.6、OpenSORA 等知名開源模型。這充分證明了 Emu3 在多模態(tài)處理方面的強(qiáng)大實(shí)力。例如,在圖像生成任務(wù)中,Emu3 能夠生成更加逼真、細(xì)膩的圖像,細(xì)節(jié)豐富度和色彩還原度都達(dá)到了很高的水平;在視覺語言理解任務(wù)中,它能夠準(zhǔn)確地理解圖像中的內(nèi)容,并生成與之對(duì)應(yīng)的文本描述;在視頻生成任務(wù)中,它可以生成高質(zhì)量、流暢的視頻,具有很高的視覺效果和藝術(shù)價(jià)值。
二、Emu3 的應(yīng)用前景
1. 機(jī)器人大腦
Emu3 的多模態(tài)處理能力可以為機(jī)器人提供更加智能的大腦。通過對(duì)圖像、文本和視頻的綜合理解,機(jī)器人可以更好地感知周圍環(huán)境,與人類進(jìn)行更加自然的交互,并執(zhí)行更加復(fù)雜的任務(wù)。例如,在家庭服務(wù)機(jī)器人中,Emu3 可以幫助機(jī)器人識(shí)別不同的物品、理解人類的指令,并根據(jù)環(huán)境變化做出相應(yīng)的反應(yīng)。
2. 自動(dòng)駕駛
在自動(dòng)駕駛領(lǐng)域,Emu3 可以通過對(duì)攝像頭拍攝的圖像和視頻進(jìn)行實(shí)時(shí)分析,識(shí)別道路標(biāo)志、車輛和行人等物體,并預(yù)測(cè)它們的運(yùn)動(dòng)軌跡。同時(shí),它還可以結(jié)合地圖信息和交通規(guī)則等文本數(shù)據(jù),為自動(dòng)駕駛系統(tǒng)提供更加準(zhǔn)確的決策依據(jù)。這將大大提高自動(dòng)駕駛的安全性和可靠性。
3. 多模態(tài)對(duì)話和推理
Emu3 可以應(yīng)用于多模態(tài)對(duì)話和推理系統(tǒng)中,實(shí)現(xiàn)更加自然、智能的人機(jī)交互。例如,在智能客服領(lǐng)域,它可以通過對(duì)用戶的語音、圖像和文本輸入進(jìn)行綜合分析,理解用戶的問題,并給出準(zhǔn)確的回答;在智能教育領(lǐng)域,它可以根據(jù)學(xué)生的學(xué)習(xí)情況和需求,生成個(gè)性化的教學(xué)內(nèi)容和輔導(dǎo)方案。
三、Emu3 本地部署實(shí)踐
1、克隆代碼
git clone https://github.com/baaivision/Emu3
2、安裝依賴
cd Emu3
pip install -r requirements.txt
3、模型推理
使用Transformers運(yùn)行Emu3-Gen/Stage1進(jìn)行圖像生成
from PIL import Image
from transformers import AutoTokenizer, AutoModel, AutoImageProcessor, AutoModelForCausalLM
from transformers.generation.configuration_utils import GenerationConfig
from transformers.generation import LogitsProcessorList, PrefixConstrainedLogitsProcessor, UnbatchedClassifierFreeGuidanceLogitsProcessor
import torch
from emu3.mllm.processing_emu3 import Emu3Processor
# model path
EMU_HUB = "BAAI/Emu3-Gen"
VQ_HUB = "BAAI/Emu3-VisionTokenizer"
# prepare model and processor
model = AutoModelForCausalLM.from_pretrained(
EMU_HUB,
device_map="cuda:0",
torch_dtype=torch.bfloat16,
attn_implementatinotallow="flash_attention_2",
trust_remote_code=True,
)
tokenizer = AutoTokenizer.from_pretrained(EMU_HUB, trust_remote_code=True, padding_side="left")
image_processor = AutoImageProcessor.from_pretrained(VQ_HUB, trust_remote_code=True)
image_tokenizer = AutoModel.from_pretrained(VQ_HUB, device_map="cuda:0", trust_remote_code=True).eval()
processor = Emu3Processor(image_processor, image_tokenizer, tokenizer)
# prepare input
POSITIVE_PROMPT = " masterpiece, film grained, best quality."
NEGATIVE_PROMPT = "lowres, bad anatomy, bad hands, text, error, missing fingers, extra digit, fewer digits, cropped, worst quality, low quality, normal quality, jpeg artifacts, signature, watermark, username, blurry."
classifier_free_guidance = 3.0
prompt = "a portrait of young girl."
prompt += POSITIVE_PROMPT
kwargs = dict(
mode='G',
ratio="1:1",
image_area=model.config.image_area,
return_tensors="pt",
padding="longest",
)
pos_inputs = processor(text=prompt, **kwargs)
neg_inputs = processor(text=NEGATIVE_PROMPT, **kwargs)
# prepare hyper parameters
GENERATION_CONFIG = GenerationConfig(
use_cache=True,
eos_token_id=model.config.eos_token_id,
pad_token_id=model.config.pad_token_id,
max_new_tokens=40960,
do_sample=True,
top_k=2048,
)
h = pos_inputs.image_size[:, 0]
w = pos_inputs.image_size[:, 1]
constrained_fn = processor.build_prefix_constrained_fn(h, w)
logits_processor = LogitsProcessorList([
UnbatchedClassifierFreeGuidanceLogitsProcessor(
classifier_free_guidance,
model,
unconditional_ids=neg_inputs.input_ids.to("cuda:0"),
),
PrefixConstrainedLogitsProcessor(
constrained_fn ,
num_beams=1,
),
])
# generate
outputs = model.generate(
pos_inputs.input_ids.to("cuda:0"),
GENERATION_CONFIG,
logits_processor=logits_processor,
attention_mask=pos_inputs.attention_mask.to("cuda:0"),
)
mm_list = processor.decode(outputs[0])
for idx, im in enumerate(mm_list):
if not isinstance(im, Image.Image):
continue
im.save(f"result_{idx}.png")
使用Transformers運(yùn)行Emu3-Chat/Stage1以實(shí)現(xiàn)視覺語言理解
from PIL import Image
from transformers import AutoTokenizer, AutoModel, AutoImageProcessor, AutoModelForCausalLM
from transformers.generation.configuration_utils import GenerationConfig
import torch
from emu3.mllm.processing_emu3 import Emu3Processor
# model path
EMU_HUB = "BAAI/Emu3-Chat"
VQ_HUB = "BAAI/Emu3-VisionTokenier"
# prepare model and processor
model = AutoModelForCausalLM.from_pretrained(
EMU_HUB,
device_map="cuda:0",
torch_dtype=torch.bfloat16,
attn_implementatinotallow="flash_attention_2",
trust_remote_code=True,
)
# used for Emu3-Chat
tokenizer = AutoTokenizer.from_pretrained(EMU_HUB, trust_remote_code=True, padding_side="left")
# used for Emu3-Stage1
# tokenizer = AutoTokenizer.from_pretrained(
# EMU_HUB,
# trust_remote_code=True,
# chat_template="{image_prompt}{text_prompt}",
# padding_side="left",
# )
image_processor = AutoImageProcessor.from_pretrained(VQ_HUB, trust_remote_code=True)
image_tokenizer = AutoModel.from_pretrained(VQ_HUB, device_map="cuda:0", trust_remote_code=True).eval()
processor = Emu3Processor(image_processor, image_tokenizer, tokenizer)
# prepare input
text = "Please describe the image"
image = Image.open("assets/demo.png")
inputs = processor(
text=text,
image=image,
mode='U',
return_tensors="pt",
padding="longest",
)
# prepare hyper parameters
GENERATION_CONFIG = GenerationConfig(
pad_token_id=tokenizer.pad_token_id,
bos_token_id=tokenizer.bos_token_id,
eos_token_id=tokenizer.eos_token_id,
max_new_tokens=1024,
)
# generate
outputs = model.generate(
inputs.input_ids.to("cuda:0"),
GENERATION_CONFIG,
attention_mask=pos_inputs.attention_mask.to("cuda:0"),
)
outputs = outputs[:, inputs.input_ids.shape[-1]:]
print(processor.batch_decode(outputs, skip_special_tokens=True)[0])
四、結(jié)語
Emu3 作為北京智源人工智能研究院推出的原生多模態(tài)世界模型,代表了人工智能領(lǐng)域的一次重大突破。它的多模態(tài)統(tǒng)一理解與生成能力、創(chuàng)新的技術(shù)架構(gòu)和卓越的性能表現(xiàn),為我們展示了人工智能未來的發(fā)展方向。雖然它還面臨著一些挑戰(zhàn),但隨著技術(shù)的不斷進(jìn)步和完善,相信 Emu3 將在機(jī)器人大腦、自動(dòng)駕駛、多模態(tài)對(duì)話和推理等領(lǐng)域發(fā)揮重要作用,為我們的生活帶來更多的便利和創(chuàng)新。
相關(guān)資料
github地址:??https://github.com/baaivision/Emu3??
Emu3-VisionTokenizer模型:??https://modelscope.cn/models/BAAI/Emu3-VisionTokenizer??
Emu3-Gen模型:??https://modelscope.cn/models/BAAI/Emu3-Gen??
Emu3-Chat模型:??https://modelscope.cn/models/BAAI/Emu3-Chat??
本文轉(zhuǎn)載自??小兵的AI視界??,作者: 小兵
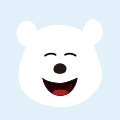