基于BLIP-2和Gemini開發(fā)多模態(tài)搜索引擎代理 原創(chuàng)
本文將利用基于文本和圖像的聯(lián)合搜索功能來開發(fā)一個多模態(tài)時裝輔助代理應用程序。
簡介
傳統(tǒng)模型只能處理單一類型的數(shù)據(jù),例如文本、圖像或表格數(shù)據(jù)。多模態(tài)是人工智能研究界的一個流行概念,指的是模型能夠同時從多種類型的數(shù)據(jù)中學習。這項新技術(并不是很新,但在過去幾個月里有了顯著的改進)有許多潛在的應用,它將改變許多產(chǎn)品的用戶體驗。
這方面一個很好的例子是未來搜索引擎的新工作方式:用戶可以使用多種方式輸入查詢,例如文本、圖像、音頻等。另一個例子是改進人工智能驅(qū)動的客戶支持系統(tǒng),以實現(xiàn)語音和文本輸入。在電子商務中,他們通過允許用戶使用圖像和文本進行搜索來增強產(chǎn)品發(fā)現(xiàn)。我們將在本文中使用后者作為案例研究。
前沿的一些人工智能研究實驗室每月都會推出幾種支持多模態(tài)的模型。例如,OpenAI公司的CLIP和DALL-E;Salesforce公司的BLIP-2將圖像和文本結合在一起;Meta的ImageBind將多模態(tài)概念擴展到六種模態(tài)(文本、音頻、深度、溫度、圖像和慣性測量單元)。
在本文中,我們將通過解釋BLIP-2的架構、損失函數(shù)的工作方式及其訓練過程來對它展開詳細探索。我們還提供了一個實際用例,該用例結合了BLIP-2和Gemini兩種模型,以創(chuàng)建一個多模態(tài)時尚搜索代理,該代理可以幫助客戶根據(jù)文本或文本和圖像組合提示找到最佳服裝。
圖1:多模態(tài)搜索代理(圖片由作者使用Gemini提供)
與往常一樣,本文對應的示例代碼可在??我們的GitHub代碼倉庫??上獲取。
BLIP-2:多模態(tài)模型
BLIP-2(引導式語言圖像預訓練)(【引文1】)是一種視覺語言模型,旨在解決諸如視覺問答或基于兩種模態(tài)輸入(圖像和文本)的多模態(tài)推理等任務。正如我們將在下面看到的,該模型是為了解決視覺語言領域的兩個主要挑戰(zhàn)而開發(fā)的:
- 使用凍結的預訓練視覺編碼器和LLM降低計算成本,與視覺和語言網(wǎng)絡的聯(lián)合訓練相比,大幅減少所需的訓練資源。
- 通過引入Q-Former來改善視覺語言對齊。Q-Former使視覺和文本嵌入更加接近,從而提高了推理任務的性能和執(zhí)行多模態(tài)檢索的能力。
架構
BLIP-2的架構采用模塊化設計,集成了三個模塊:
- Visual Encoder:一種凍結的視覺模型,例如ViT,它從輸入圖像中提取視覺嵌入(然后用于下游任務)。
- 查詢轉(zhuǎn)換器(Q-Former):是此架構的關鍵。它由一個可訓練的輕量級轉(zhuǎn)換器組成,充當視覺模型和語言模型之間的中間層。它負責從視覺嵌入生成上下文化查詢,以便語言模型能夠有效地處理它們。
- LLM:一種凍結的預訓練LLM,可處理精煉的視覺嵌入以生成文本描述或答案。
圖2:BLIP-2架構(圖片來自作者本人)
損失函數(shù)
BLIP-2有三個損失函數(shù)來訓練Q-Former模塊:
- 圖像-文本對比損失(【引文2】):通過最大化成對的圖像-文本表示的相似性,同時推開不相似的圖像-文本對,來強制視覺和文本嵌入之間的對齊。
- 圖像-文本匹配損失(【引文3】):一種二元分類損失,旨在通過預測文本描述是否與圖像匹配(正,即目標=1)或不匹配(負,即目標=0)來使模型學習細粒度對齊。
- 基于圖像的文本生成損失(【引文4】):是LLM中使用的交叉熵損失,用于預測序列中下一個標記的概率。Q-Former架構不允許圖像嵌入和文本標記之間進行交互;因此,必須僅基于視覺信息生成文本,從而迫使模型提取相關的視覺特征。
對于圖像文本對比損失和圖像文本匹配損失,作者使用了批量負采樣技術。這意味著,如果我們的批量大小為512,則每個圖像文本對都有一個正樣本和511個負樣本。這種方法提高了效率,因為負樣本是從批次中抽取的,不需要搜索整個數(shù)據(jù)集。它還提供了一組更加多樣化的比較,從而實現(xiàn)更好的梯度估計和更快的收斂。
圖3:訓練損失解釋(圖片來自作者本人)
訓練過程
BLIP-2的訓練包含兩個階段:
第1階段——引導視覺語言表征:
- 該模型接收圖像作為輸入,然后使用凍結的視覺編碼器將其轉(zhuǎn)換為嵌入。
- 除了這些圖像,模型還會接收它們的文本描述,并將其轉(zhuǎn)換為嵌入。
- Q-Former使用圖像文本對比損失進行訓練,確保視覺嵌入與其對應的文本嵌入緊密對齊,并遠離不匹配的文本描述。同時,圖像文本匹配損失通過學習對給定文本是否正確描述圖像進行分類,幫助模型開發(fā)細粒度表示。
圖4:第一階段訓練過程(圖片來自作者本人)
第2階段——引導視覺到語言的生成:
- 預訓練語言模型被集成到架構中,以根據(jù)先前學習的表示生成文本。
- 通過使用基于圖像的文本生成損失,將重點從對齊轉(zhuǎn)移到文本生成,從而提高模型的推理和文本生成能力。
圖5:第二階段訓練過程(圖片由作者提供)
使用BLIP-2和Gemini創(chuàng)建多模態(tài)時尚搜索代理
在本節(jié)中,我們將利用BLIP-2的多模態(tài)功能構建一個時尚代理搜索代理,該代理可以接收輸入的文本和/或圖像并返回建議。對于代理的對話功能,我們將使用VertexAI中托管的Gemini 1.5 Pro;對于界面,我們將構建一個Streamlit應用實現(xiàn)。
本實例中使用的時尚數(shù)據(jù)集是根據(jù)MIT許可證授權的,可以通過以下鏈接訪問:??時尚產(chǎn)品圖像數(shù)據(jù)集??,它包含超過44,000張時尚產(chǎn)品圖像。
實現(xiàn)此目的的第一步是設置一個向量數(shù)據(jù)庫。這使代理能夠根據(jù)商店中可用商品的圖像嵌入以及輸入中的文本或圖像嵌入執(zhí)行向量化搜索。我們使用Docker和docker-compose來幫助我們設置環(huán)境:
- Docker-Compose與Postgres(數(shù)據(jù)庫)和允許向量化搜索的PGVector擴展一起使用。
services:
postgres:
container_name: container-pg
image: ankane/pgvector
hostname: localhost
ports:
- "5432:5432"
env_file:
- ./env/postgres.env
volumes:
- postgres-data:/var/lib/postgresql/data
restart: unless-stopped
pgadmin:
container_name: container-pgadmin
image: dpage/pgadmin4
depends_on:
- postgres
ports:
- "5050:80"
env_file:
- ./env/pgadmin.env
restart: unless-stopped
volumes:
postgres-data:
Postgres對應的.env文件定義部分,其中包含用于登錄數(shù)據(jù)庫的變量。
POSTGRES_DB=postgres
POSTGRES_USER=admin
POSTGRES_PASSWORD=root
Pgadmin對應的.env文件定義部分,其中包含用于登錄UI以手動查詢數(shù)據(jù)庫的變量(可選)。
PGADMIN_DEFAULT_EMAIL=admin@admin.com
PGADMIN_DEFAULT_PASSWORD=root
連接功能對應的.env文件部分,包含使用Langchain連接到PGVector所需的所有組件。
DRIVER=psycopg
HOST=localhost
PORT=5432
DATABASE=postgres
USERNAME=admin
PASSWORD=root
一旦設置并運行Vector DB(docker-compose up -d),就該創(chuàng)建代理和工具來執(zhí)行多模態(tài)搜索了。我們構建了兩個代理來解決此場景應用:一個用于了解用戶的請求,另一個用于提供建議:
- 分類器:負責接收來自客戶的輸入消息并提取用戶正在尋找的衣服類別,例如T恤、褲子、鞋子、運動衫或襯衫。它還將返回客戶想要的商品數(shù)量,以便我們可以從Vector DB中檢索準確的數(shù)量。
from langchain_core.output_parsers import PydanticOutputParser
from langchain_core.prompts import PromptTemplate
from langchain_google_vertexai import ChatVertexAI
from pydantic import BaseModel, Field
class ClassifierOutput(BaseModel):
"""
模型輸出的數(shù)據(jù)結構。
"""
category: list = Field(
description="A list of clothes category to search for ('t-shirt', 'pants', 'shoes', 'jersey', 'shirt')."
)
number_of_items: int = Field(description="The number of items we should retrieve.")
class Classifier:
"""
用于輸入文本分類的分類器類。
"""
def __init__(self, model: ChatVertexAI) -> None:
"""
通過創(chuàng)建鏈來初始化 Chain 類。
參數(shù):
model (ChatVertexAI): 大型語言模型 (LLM)。
"""
super().__init__()
parser = PydanticOutputParser(pydantic_object=ClassifierOutput)
text_prompt = """
You are a fashion assistant expert on understanding what a customer needs and on extracting the category or categories of clothes a customer wants from the given text.
Text:
{text}
Instructions:
1. Read carefully the text.
2. Extract the category or categories of clothes the customer is looking for, it can be:
- t-shirt if the custimer is looking for a t-shirt.
- pants if the customer is looking for pants.
- jacket if the customer is looking for a jacket.
- shoes if the customer is looking for shoes.
- jersey if the customer is looking for a jersey.
- shirt if the customer is looking for a shirt.
3. If the customer is looking for multiple items of the same category, return the number of items we should retrieve. If not specfied but the user asked for more than 1, return 2.
4. If the customer is looking for multiple category, the number of items should be 1.
5. Return a valid JSON with the categories found, the key must be 'category' and the value must be a list with the categories found and 'number_of_items' with the number of items we should retrieve.
Provide the output as a valid JSON object without any additional formatting, such as backticks or extra text. Ensure the JSON is correctly structured according to the schema provided below.
{format_instructions}
Answer:
"""
prompt = PromptTemplate.from_template(
text_prompt, partial_variables={"format_instructions": parser.get_format_instructions()}
)
self.chain = prompt | model | parser
def classify(self, text: str) -> ClassifierOutput:
"""
根據(jù)文本上下文從模型獲取類別。
參數(shù):
text (str): 用戶消息。
返回值:
ClassifierOutput:模型的答案。
"""
try:
return self.chain.invoke({"text": text})
except Exception as e:
raise RuntimeError(f"Error invoking the chain: {e}")
- 助手:負責使用從Vector DB中檢索到的個性化建議進行回答。在這種情況下,我們還利用Gemini的多模態(tài)功能來分析檢索到的圖像并給出更好的答案。
from langchain_core.output_parsers import PydanticOutputParser
from langchain_core.prompts import PromptTemplate
from langchain_google_vertexai import ChatVertexAI
from pydantic import BaseModel, Field
class AssistantOutput(BaseModel):
"""
模型輸出的數(shù)據(jù)結構。
"""
answer: str = Field(description="A string with the fashion advice for the customer.")
class Assistant:
"""
提供時尚建議的代理類。
"""
def __init__(self, model: ChatVertexAI) -> None:
"""
通過創(chuàng)建鏈來初始化鏈類。
參數(shù):
model (ChatVertexAI): LLM模型.
"""
super().__init__()
parser = PydanticOutputParser(pydantic_object=AssistantOutput)
text_prompt = """
You work for a fashion store and you are a fashion assistant expert on understanding what a customer needs.
Based on the items that are available in the store and the customer message below, provide a fashion advice for the customer.
Number of items: {number_of_items}
Images of items:
{items}
Customer message:
{customer_message}
Instructions:
1. Check carefully the images provided.
2. Read carefully the customer needs.
3. Provide a fashion advice for the customer based on the items and customer message.
4. Return a valid JSON with the advice, the key must be 'answer' and the value must be a string with your advice.
Provide the output as a valid JSON object without any additional formatting, such as backticks or extra text. Ensure the JSON is correctly structured according to the schema provided below.
{format_instructions}
Answer:
"""
prompt = PromptTemplate.from_template(
text_prompt, partial_variables={"format_instructions": parser.get_format_instructions()}
)
self.chain = prompt | model | parser
def get_advice(self, text: str, items: list, number_of_items: int) -> AssistantOutput:
"""
根據(jù)文本和項上下文從模型中獲取建議。
參數(shù):
text (str): 用戶消息。
items (list): 為客戶找到的項。
number_of_items (int): 要檢索的項數(shù)。
Returns:
AssistantOutput: 模型的答案。
"""
try:
return self.chain.invoke({"customer_message": text, "items": items, "number_of_items": number_of_items})
except Exception as e:
raise RuntimeError(f"Error invoking the chain: {e}")
在工具方面,我們基于BLIP-2定義了一個工具。它由一個函數(shù)組成,該函數(shù)接收文本或圖像作為輸入并返回規(guī)范化的嵌入。根據(jù)輸入,嵌入是使用BLIP-2的文本嵌入模型或圖像嵌入模型生成的。
from typing import Optional
import numpy as np
import torch
import torch.nn.functional as F
from PIL import Image
from PIL.JpegImagePlugin import JpegImageFile
from transformers import AutoProcessor, Blip2TextModelWithProjection, Blip2VisionModelWithProjection
PROCESSOR = AutoProcessor.from_pretrained("Salesforce/blip2-itm-vit-g")
TEXT_MODEL = Blip2TextModelWithProjection.from_pretrained("Salesforce/blip2-itm-vit-g", torch_dtype=torch.float32).to(
"cpu"
)
IMAGE_MODEL = Blip2VisionModelWithProjection.from_pretrained(
"Salesforce/blip2-itm-vit-g", torch_dtype=torch.float32
).to("cpu")
def generate_embeddings(text: Optional[str] = None, image: Optional[JpegImageFile] = None) -> np.ndarray:
"""
使用Blip2模型從文本或圖像中生成嵌入。
參數(shù):
text (Optional[str]): 客戶輸入文本
image (Optional[Image]): 客戶輸入圖像
返回值:
np.ndarray: 嵌入向量
"""
if text:
inputs = PROCESSOR(text=text, return_tensors="pt").to("cpu")
outputs = TEXT_MODEL(**inputs)
embedding = F.normalize(outputs.text_embeds, p=2, dim=1)[:, 0, :].detach().numpy().flatten()
else:
inputs = PROCESSOR(images=image, return_tensors="pt").to("cpu", torch.float16)
outputs = IMAGE_MODEL(**inputs)
embedding = F.normalize(outputs.image_embeds, p=2, dim=1).mean(dim=1).detach().numpy().flatten()
return embedding
請注意,我們使用不同的嵌入模型創(chuàng)建與PGVector的連接,因為它是強制性的,但由于我們將直接存儲由BLIP-2生成的嵌入,因此不會使用它。
在下面的循環(huán)中,我們遍歷所有服裝類別,加載圖像,并創(chuàng)建要存儲在向量數(shù)據(jù)庫中的嵌入并將其附加到列表中。此外,我們將圖像的路徑存儲為文本,以便我們可以在Streamlit應用中展示它。最后,我們存儲起類別,以便根據(jù)分類器代理預測的類別過濾結果。
import glob
import os
from dotenv import load_dotenv
from langchain_huggingface.embeddings import HuggingFaceEmbeddings
from langchain_postgres.vectorstores import PGVector
from PIL import Image
from blip2 import generate_embeddings
load_dotenv("env/connection.env")
CONNECTION_STRING = PGVector.connection_string_from_db_params(
driver=os.getenv("DRIVER"),
host=os.getenv("HOST"),
port=os.getenv("PORT"),
database=os.getenv("DATABASE"),
user=os.getenv("USERNAME"),
password=os.getenv("PASSWORD"),
)
vector_db = PGVector(
embeddings=HuggingFaceEmbeddings(model_name="nomic-ai/modernbert-embed-base"), # 這對我們的情況來說并不重要
collection_name="fashion",
connection=CONNECTION_STRING,
use_jsonb=True,
)
if __name__ == "__main__":
# 生成圖像嵌入
# 以文本形式保存圖像的路徑
# 在元數(shù)據(jù)中保存類別
texts = []
embeddings = []
metadatas = []
for category in glob.glob("images/*"):
cat = category.split("/")[-1]
for img in glob.glob(f"{category}/*"):
texts.append(img)
embeddings.append(generate_embeddings(image=Image.open(img)).tolist())
metadatas.append({"category": cat})
vector_db.add_embeddings(texts, embeddings, metadatas)
現(xiàn)在,我們可以構建Streamlit應用程序,以便與我們的代理聊天并征求建議了。聊天從代理詢問它可以提供什么幫助開始,并為客戶提供一個組件框來編寫消息和/或上傳文件。
一旦客戶回復,工作流程如下:
- 分類代理可以識別顧客正在尋找哪些類別的衣服以及他們想要多少件。
- 如果客戶上傳文件,該文件將被轉(zhuǎn)換為嵌入,我們將根據(jù)客戶想要的衣服類別和單位數(shù)量在向量數(shù)據(jù)庫中尋找類似的項目。
- 然后,檢索到的項目和客戶的輸入信息被發(fā)送給代理代理,以產(chǎn)生與檢索到的圖像一起呈現(xiàn)的推薦信息。
- 如果客戶沒有上傳文件,流程是相同的,但我們不是生成用于檢索的圖像嵌入,而是創(chuàng)建文本嵌入。
import os
import streamlit as st
from dotenv import load_dotenv
from langchain_google_vertexai import ChatVertexAI
from langchain_huggingface.embeddings import HuggingFaceEmbeddings
from langchain_postgres.vectorstores import PGVector
from PIL import Image
import utils
from assistant import Assistant
from blip2 import generate_embeddings
from classifier import Classifier
load_dotenv("env/connection.env")
load_dotenv("env/llm.env")
CONNECTION_STRING = PGVector.connection_string_from_db_params(
driver=os.getenv("DRIVER"),
host=os.getenv("HOST"),
port=os.getenv("PORT"),
database=os.getenv("DATABASE"),
user=os.getenv("USERNAME"),
password=os.getenv("PASSWORD"),
)
vector_db = PGVector(
embeddings=HuggingFaceEmbeddings(model_name="nomic-ai/modernbert-embed-base"), #這對我們的情況來說并不重要
collection_name="fashion",
connection=CONNECTION_STRING,
use_jsonb=True,
)
model = ChatVertexAI(model_name=os.getenv("MODEL_NAME"), project=os.getenv("PROJECT_ID"), temperarture=0.0)
classifier = Classifier(model)
assistant = Assistant(model)
st.title("Welcome to ZAAI's Fashion Assistant")
user_input = st.text_input("Hi, I'm ZAAI's Fashion Assistant. How can I help you today?")
uploaded_file = st.file_uploader("Upload an image", type=["jpg", "jpeg", "png"])
if st.button("Submit"):
#了解用戶的要求
classification = classifier.classify(user_input)
if uploaded_file:
image = Image.open(uploaded_file)
image.save("input_image.jpg")
embedding = generate_embeddings(image=image)
else:
# 在用戶不上傳圖像時創(chuàng)建文本嵌入
embedding = generate_embeddings(text=user_input)
# 創(chuàng)建要檢索的項目和路徑的列表
retrieved_items = []
retrieved_items_path = []
for item in classification.category:
clothes = vector_db.similarity_search_by_vector(
embedding, k=classification.number_of_items, filter={"category": {"$in": [item]}}
)
for clothe in clothes:
retrieved_items.append({"bytesBase64Encoded": utils.encode_image_to_base64(clothe.page_content)})
retrieved_items_path.append(clothe.page_content)
#得到助理的建議
assistant_output = assistant.get_advice(user_input, retrieved_items, len(retrieved_items))
st.write(assistant_output.answer)
cols = st.columns(len(retrieved_items)+1)
for col, retrieved_item in zip(cols, ["input_image.jpg"]+retrieved_items_path):
col.image(retrieved_item)
user_input = st.text_input("")
else:
st.warning("Please provide text.")
上面這兩個例子運行結果如下所示:
圖6顯示了一個例子,其中客戶上傳了一張紅色T恤的圖片并要求代理商完成服裝制作。
圖6:文本和圖像輸入的示例(圖片來自作者本人)
圖7顯示了一個更直接的例子,客戶要求代理向他們展示黑色T恤。
圖7:文本輸入示例(圖片來自作者本人)
結論
多模態(tài)AI已不再僅僅是一個研究課題。它正在業(yè)界用于重塑客戶與公司產(chǎn)品目錄的互動方式。在本文中,我們探討了如何結合使用BLIP-2和Gemini等多模態(tài)模型來解決實際問題,并以可擴展的方式為客戶提供更加個性化的體驗。
其中,我們深入探索了BLIP-2的架構,展示了它如何彌合文本和圖像模態(tài)之間的差距。為了擴展其功能,我們開發(fā)了一個代理系統(tǒng),每個代理專門負責不同的任務。該系統(tǒng)集成了LLM(Gemini)和向量數(shù)據(jù)庫,可以使用文本和圖像嵌入檢索產(chǎn)品目錄。我們還利用Gemini的多模態(tài)推理來改進銷售輔助代理的響應,使其更像真實的人類。
總之,借助BLIP-2、Gemini和PG Vector等工具,多模態(tài)搜索和檢索的未來已經(jīng)實現(xiàn),未來的搜索引擎將與我們今天使用的搜索引擎大不相同。
參考文獻
【1】Junnan Li、Dongxu Li、Silvio Savarese、Steven Hoi,2023年。BLIP-2: Bootstrapping Language-Image Pre-training with Frozen Image Encoders and Large Language Models(BLIP-2:使用凍結圖像編碼器和大型語言模型進行引導語言圖像預訓練),arXiv:2301.12597。
【2】Prannay Khosla、Piotr Teterwak、Chen Wang、Aaron Sarna、Yonglong Tian、Phillip Isola、Aaron Maschinot、Ce Liu、Dilip Krishnan,2020年。Supervised Contrastive Learning(監(jiān)督對比學習),arXiv:2004.11362。
【3】Junnan Li、Ramprasaath R. Selvaraju、Akhilesh Deepak Gotmare、Shafiq Joty、Caiming Xiong、Steven Hoi,2021年。Align before Fuse: Vision and Language Representation Learning with Momentum Distillation(融合前對齊:使用動量蒸餾進行視覺和語言表征學習),arXiv:2107.07651。
【4】李東,南陽,王文輝,魏福如,劉曉東,王宇,高劍鋒,周明,Hsiao-Wen Hon。2019。Unified Language Model Pre-training for Natural Language Understanding and Generation(自然語言理解和生成的統(tǒng)一語言模型預訓練),arXiv:1905.03197。
譯者介紹
朱先忠,51CTO社區(qū)編輯,51CTO專家博客、講師,濰坊一所高校計算機教師,自由編程界老兵一枚。
原文標題:??Multimodal Search Engine Agents Powered by BLIP-2 and Gemini??,作者:Luís Roque,Rafael Guedes
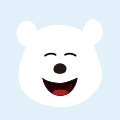