機(jī)器學(xué)習(xí)|從0開發(fā)大模型之復(fù)現(xiàn)DeepSeek的aha moment
前面一篇文章介紹了??《從0開發(fā)大模型之DeepSeek的GRPO》??,并且實現(xiàn)了一個簡單版本的 GRPO? 代碼,不過從工程領(lǐng)域來看,并沒有復(fù)現(xiàn)DeepSeek-R1,于是最近申請了48G的顯存,結(jié)合一些開源的方案復(fù)現(xiàn)aha monent,并給出完整的代碼和工具鏈。
1、什么是 aha monent
DeepSeek-R1 論文中提到,模型讓作者「見證了強(qiáng)化學(xué)習(xí)的力量和美感」,在DeepSeek-R1-Zero的中間版本,「頓悟時刻」來了:模型學(xué)會了以人類的語氣進(jìn)行反思。
aha monent
2、使用什么的基座模型和訓(xùn)練數(shù)據(jù)
- 由于顯卡只有48G,可以用基座模型Qwen2.5,模型大?。?.5B,1.5B,3B
- 訓(xùn)練數(shù)據(jù)有很多:(可以直接在huggingface上找到)
a.AI-MO/NuminaMath-TIR:包括72K行的數(shù)學(xué)問題,解決方案和答案,是從NuminaMath-CoT 數(shù)據(jù)集提煉出來的
b.FreedomIntelligence/medical-o1-verifiable-problem:包括40K行的醫(yī)學(xué)數(shù)據(jù),不過沒有相關(guān)的推理過程
c.https://raw.githubusercontent.com/hkust-nlp/simpleRL-reason/refs/heads/main/train/data/math_level3to5_data_processed_with_qwen_prompt.json:在simpleRL-reason的開源項目中的訓(xùn)練數(shù)據(jù)集
3、如何訓(xùn)練
3.1、設(shè)計獎勵函數(shù)
從上一篇??《從0開發(fā)大模型之DeepSeek的GRPO》??中已經(jīng)了解GRPO的原理,其中一部分是包括獎勵函數(shù)的設(shè)計,其中如何設(shè)計這里就省略,本文暫時參考其他復(fù)現(xiàn)R1的項目設(shè)使用了5個函數(shù):
- accuracy_reward:驗證答案的準(zhǔn)確性,對就返回1,不對就返回0
- format_reward:驗證格式的準(zhǔn)確性,如果符合^<think>.*?</think><answer>.*?</answer>$的返回則返回1,否則就返回0
- reasoning_steps_reward:有推理步驟的,類似(Step \d+:|^\d+\.|\n-|\n\*|First,|Second,|Next,|Finally,),最大值返回3,否則返回0
- cosine_reward:基于答案的長度做余弦,分為正確答案最大長度,正確答案最小長度,錯誤答案最大長度,錯誤答案最小長度
- repetition_penalty_reward:計算N-gram 重復(fù)獎勵
- length_reward:參考kimi1.5的論文(https://arxiv.org/abs/2501.12599)
a.正確答案長度獎勵: reward = 0.5 - (len - min_len)/(max_len - min_len)
b.錯誤答案長度獎勵: reward = min(0, 0.5 - (len - min_len)/(max_len - min_len))
3.2、使用vLLM
為了提升性能和節(jié)省顯存,這里使用了vLLM,vLLM是一個開源的大模型推理加速框架,通過PagedAttention高效地管理attention中緩存的張量,實現(xiàn)比HuggingFace Transformers高14-24倍的吞吐量,從本文實驗過程中發(fā)現(xiàn),之前需要60G顯存的,基本40G就能跑起來。
由于vLLM的加載模型和Huggingface的可以直接兼容,所以可以參考如下代碼跑起來:
from vllm import LLM, SamplingParams
if __name__ == '__main__':
model_path = "{模型名稱}"
model = LLM(model=model_path,
tensor_parallel_size=1,
trust_remote_code=True,
max_model_len=10000,
enforce_eager=True,
gpu_memory_utilizatinotallow=0.5,
block_size=32)
sampling_params = SamplingParams(temperature=0, max_tokens=1, prompt_logprobs=20)
prompt = "vLLM是如何實現(xiàn)的?"
response = model.generate(prompt, sampling_params, use_tqdm=False)[0]
print(response, '\n\n', response.outputs)
3.3、使用Accelerate和deepspeed加速訓(xùn)練
Accelerate是PyTorch官方提供的分布式訓(xùn)練工具,而deepspeed是由Microsoft提供的分布式訓(xùn)練工具,最主要的區(qū)別在于支持的模型規(guī)模不同,deepspeed支持更大規(guī)模的模型,deepspeed還提供了更多的優(yōu)化策略和工具,例如ZeRO和Offload等,Accelerate更加穩(wěn)定和易于使用,適合中小規(guī)模的訓(xùn)練任務(wù),不過huggingface已經(jīng)集成了deepspeed,如果對于訓(xùn)練改幾行代碼即可,如下:
#!pip install accelerate
#!pip install deepspeed
import torch
import torch.nn.functional as F
from datasets import load_dataset
# 引入基礎(chǔ)庫accelerate
from accelerate import Accelerator
# 創(chuàng)建accelerator
accelerator = Accelerator()
# 修改設(shè)備信息
device = accelerator.device
model = torch.nn.Transformer().to(device)
optimizer = torch.optim.Adam(model.parameters())
dataset = load_dataset({需要加載的數(shù)據(jù)})
data = torch.utils.data.DataLoader(dataset, shuffle=True)
# 使用accelerator訓(xùn)練
model, optimizer, data = accelerator.prepare(model, optimizer, data)
model.train()
for epoch in range(10):
for source, targets in data:
source = source.to(device)
targets = targets.to(device)
optimizer.zero_grad()
output = model(source)
loss = F.cross_entropy(output, targets)
# 使用accelerator做backward
accelerator.backward(loss)
optimizer.step()
相關(guān)的配置可以參考zero3.yaml文件或者運行accelerate config。
4、完整的代碼
4.1、命令
需要安裝 python>=3.10 和必要的庫如下:
pip install transformers
pip install trl
pip install --upgrade trl
pip install latex2sympy2_extended math_verify
pip install flash_attn
pip install vllm
pip install deepspeed
pip install accelerate
運行的命令:
accelerate launch --config_file zero3.yaml 0-grpotrainer_r1.py
其中zero3.yaml配置:
compute_environment: LOCAL_MACHINE
debug: false
deepspeed_config:
deepspeed_multinode_launcher: standard
offload_optimizer_device: cpu
offload_param_device: cpu
zero_stage: 3
distributed_type: DEEPSPEED
downcast_bf16: 'no'
machine_rank: 0
main_training_function: main
mixed_precision: bf16
num_machines: 1
num_processes: 1
rdzv_backend: static
same_network: true
tpu_env: []
tpu_use_cluster: false
tpu_use_sudo: false
use_cpu: false
4.2、代碼
完整的訓(xùn)練代碼較大,請到本文的最后查看。
5、觀察aha moment
從上圖可以看出,模型從直接思考沒有解出問題,但是后面反復(fù)添加一些思考步驟就正確了。
6、注意事項
(1)安裝過程中錯誤:ImportError: FlashAttention2 has been toggled on, but it cannot be used due to the following error: the package flash_attn seems to be not installed. Please refer to the documentation of https://huggingface.co/docs/transformers/perf_infer_gpu_one#flashattention-2 to install Flash Attention 2.解決方案:pip install -U flash-attn
(2)安裝過程中錯誤:ImportError: vLLM is not available and use_vllm is set to True. Please install vLLM with pip install vllm to use it.解決方案:pip install -U vllm
(3)訓(xùn)練完的模型如何轉(zhuǎn)換為運行的模型?解決方案:
from deepspeed.utils.zero_to_fp32 import convert_zero_checkpoint_to_fp32_state_dict
convert_zero_checkpoint_to_fp32_state_dict(
checkpoint_dir="./output/GRPO-R1-1.5B",
output_dir="./output/GRPO-R1-1.5B",
tag="global_step9055", # 模型保存的step文件
)
(4)如果進(jìn)行模型測試?解決方案:
import torch
from transformers import AutoModelForCausalLM, AutoTokenizer
from peft import PeftConfig
# 加載Qwen模型
# model_name = "Qwen/Qwen2.5-1.5B"
# 加載本地模型
model_name = "./output/GRPO-R1-1.5B"
model = AutoModelForCausalLM.from_pretrained(model_name, cache_dir="./model")
tokenizer = AutoTokenizer.from_pretrained(model_name)
model.eval()
device = torch.device("cuda"if torch.cuda.is_available() else"cpu")
print("device: ", device)
model.to(device)
chat_history_ids = None
whileTrue:
user_input = input("用戶: ")
if user_input.lower() == "exit":
break
new_user_input_ids = tokenizer.encode(user_input + tokenizer.eos_token, return_tensors='pt').to(device)
if chat_history_ids isnotNone:
input_ids = torch.cat([chat_history_ids, new_user_input_ids], dim=-1)
else:
input_ids = new_user_input_ids
chat_history_ids = model.generate(input_ids, max_length=1000, pad_token_id=tokenizer.eos_token_id)
bot_response = tokenizer.decode(chat_history_ids[:, input_ids.shape[-1]:][0], skip_special_tokens=True)
print("機(jī)器人: ", bot_response)
7、代碼
from typing import Optional, Dict
import re, logging, os, sys, torch, math
import transformers
from transformers import (
AutoModelForCausalLM,
set_seed,
)
from transformers.trainer_utils import get_last_checkpoint
import datasets
from datasets import load_dataset
from trl import ModelConfig, ScriptArguments, GRPOConfig, GRPOTrainer, get_peft_config
from dataclasses import dataclass, field
from latex2sympy2_extended import NormalizationConfig
from math_verify import LatexExtractionConfig, parse, verify
logger = logging.getLogger(__name__)
def verify_answer(contents, solution):
rewards = []
for content, sol in zip(contents, solution):
gold_parsed = parse(
sol,
extraction_mode="first_match",
extraction_cnotallow=[LatexExtractionConfig()],
)
print('-'*100)
print(f'\ncontent:{content}\nsol:{sol}')
if len(gold_parsed) != 0:
answer_parsed = parse(
content,
extraction_cnotallow=[
LatexExtractionConfig(
normalization_cnotallow=NormalizationConfig(
nits=False,
malformed_operators=False,
basic_latex=True,
equatinotallow=True,
boxed="all",
units=True,
),
# Ensures that boxed is tried first
boxed_match_priority=0,
try_extract_without_anchor=False,
)
],
extraction_mode="first_match",
)
# Reward 1 if the content is the same as the ground truth, 0 otherwise
reward = float(verify(answer_parsed, gold_parsed))
print('-'*100)
print(f'\nanswer_parsed:{answer_parsed}\ngold_parsed:{gold_parsed}\nreward:{reward}')
else:
reward = 1.0
print(f'Failed to parse gold solution: {sol}')
rewards.append(reward)
return rewards
def accuracy_reward(completions, solution, **kwargs):
"""Reward function that checks if the completion is the same as the ground truth."""
contents = [completion[0]["content"] for completion in completions]
rewards = verify_answer(contents, solution)
print(f'\naccuracy rewards:{rewards}')
return rewards
def format_reward(completions, **kwargs):
"""Reward function that checks if the completion has a specific format."""
pattern = r"^<think>.*?</think><answer>.*?</answer>$"
completion_contents = [completion[0]["content"] for completion in completions]
matches = [re.match(pattern, content) for content in completion_contents]
rewards = [1.0if match else0.0for match in matches]
print('-'*100)
print('\nformat rewards:', rewards)
return rewards
def reasoning_steps_reward(completions, **kwargs):
"""Reward function that checks for clear step-by-step reasoning.
Regex pattern:
Step \d+: - matches "Step 1:", "Step 2:", etc.
^\d+\. - matches numbered lists like "1.", "2.", etc. at start of line
\n- - matches bullet points with hyphens
\n\* - matches bullet points with asterisks
First,|Second,|Next,|Finally, - matches transition words
"""
pattern = r"(Step \d+:|^\d+\.|\n-|\n\*|First,|Second,|Next,|Finally,)"
completion_contents = [completion[0]["content"] for completion in completions]
matches = [len(re.findall(pattern, content)) for content in completion_contents]
# Magic nubmer 3 to encourage 3 steps and more, otherwise partial reward
return [min(1.0, count / 3) for count in matches]
def len_reward(completions: list[Dict[str, str]], solution: list[str], **kwargs) -> float:
"""Compute length-based rewards to discourage overthinking and promote token efficiency.
Taken from from the Kimi 1.5 tech report: https://arxiv.org/abs/2501.12599
Args:
completions: List of model completions
solutions: List of ground truth solutions
Returns:
List of rewards where:
- For correct answers: reward = 0.5 - (len - min_len)/(max_len - min_len)
- For incorrect answers: reward = min(0, 0.5 - (len - min_len)/(max_len - min_len))
"""
contents = [completion[0]["content"] for completion in completions]
# First check correctness of answers
correctness = verify_answer(contents, solution)
# Calculate lengths
lengths = [len(content) for content in contents]
min_len = min(lengths)
max_len = max(lengths)
# If all responses have the same length, return zero rewards
if max_len == min_len:
return [0.0] * len(completions)
rewards = []
for length, is_correct in zip(lengths, correctness):
lambda_val = 0.5 - (length - min_len) / (max_len - min_len)
reward = lambda_val if is_correct > 0.0else min(0, lambda_val)
rewards.append(float(reward))
return rewards
def get_cosine_scaled_reward(
min_value_wrong: float = -1.0,
max_value_wrong: float = -0.5,
min_value_correct: float = 0.5,
max_value_correct: float = 1.0,
max_len: int = 1000,
):
def cosine_scaled_reward(completions, solution, **kwargs):
"""Reward function that scales based on completion length using a cosine schedule.
Shorter correct solutions are rewarded more than longer ones.
Longer incorrect solutions are penalized less than shorter ones.
Args:
completions: List of model completions
solution: List of ground truth solutions
This function is parameterized by the following arguments:
min_value_wrong: Minimum reward for wrong answers
max_value_wrong: Maximum reward for wrong answers
min_value_correct: Minimum reward for correct answers
max_value_correct: Maximum reward for correct answers
max_len: Maximum length for scaling
"""
contents = [completion[0]["content"] for completion in completions]
rewards = []
correctness = verify_answer(contents, solution)
lengths = [len(content) for content in contents]
for gen_len, is_correct in zip(lengths, correctness):
# Apply cosine scaling based on length
progress = gen_len / max_len
cosine = math.cos(progress * math.pi)
if is_correct > 0:
min_value = min_value_correct
max_value = max_value_correct
else:
# Swap min/max for incorrect answers
min_value = max_value_wrong
max_value = min_value_wrong
reward = min_value + 0.5 * (max_value - min_value) * (1.0 + cosine)
rewards.append(float(reward))
return rewards
return cosine_scaled_reward
def get_repetition_penalty_reward(ngram_size: int, max_penalty: float):
"""
Computes N-gram repetition penalty as described in Appendix C.2 of https://arxiv.org/abs/2502.03373.
Reference implementation from: https://github.com/eddycmu/demystify-long-cot/blob/release/openrlhf/openrlhf/reward/repetition.py
Args:
ngram_size: size of the n-grams
max_penalty: Maximum (negative) penalty for wrong answers
"""
if max_penalty > 0:
raise ValueError(f"max_penalty {max_penalty} should not be positive")
def zipngram(text: str, ngram_size: int):
words = text.lower().split()
return zip(*[words[i:] for i in range(ngram_size)])
def repetition_penalty_reward(completions, **kwargs) -> float:
"""
reward function the penalizes repetitions
ref implementation: https://github.com/eddycmu/demystify-long-cot/blob/release/openrlhf/openrlhf/reward/repetition.py
Args:
completions: List of model completions
"""
contents = [completion[0]["content"] for completion in completions]
rewards = []
for completion in contents:
if completion == "":
rewards.append(0.0)
continue
if len(completion.split()) < ngram_size:
rewards.append(0.0)
continue
ngrams = set()
total = 0
for ng in zipngram(completion, ngram_size):
ngrams.add(ng)
total += 1
scaling = 1 - len(ngrams) / total
reward = scaling * max_penalty
rewards.append(reward)
return rewards
return repetition_penalty_reward
SYSTEM_PROMPT = (
"A conversation between User and Assistant. The user asks a question, and the Assistant solves it. The assistant "
"first thinks about the reasoning process in the mind and then provides the user with the answer. The reasoning "
"process and answer are enclosed within <think> </think> and <answer> </answer> tags, respectively, i.e., "
"<think> reasoning process here </think><answer> answer here </answer>"
)
@dataclass
class R1GRPOScriptArguments(ScriptArguments):
reward_funcs: list[str] = field(
default_factory = lambda: ["accuracy", "format"],
metadata = {
"help": f"List of reward functions. Available options: 'accuracy', 'format', 'reasoning_steps', 'len', 'get_cosine_scaled', 'get_repetition_penalty'"
},
)
cosine_min_value_wrong: float = field(
default=0.0,
metadata={"help": "Minimum reward for wrong answers"},
)
cosine_max_value_wrong: float = field(
default=-0.5,
metadata={"help": "Maximum reward for wrong answers"},
)
cosine_min_value_correct: float = field(
default=0.5,
metadata={"help": "Minimum reward for correct answers"},
)
cosine_max_value_correct: float = field(
default=1.0,
metadata={"help": "Maximum reward for correct answers"},
)
cosine_max_len: int = field(
default=1000,
metadata={"help": "Maximum length for scaling"},
)
repetition_n_grams: int = field(
default=3,
metadata={"help": "Number of n-grams for repetition penalty reward"},
)
repetition_max_penalty: float = field(
default=-1.0,
metadata={"help": "Maximum (negative) penalty for for repetition penalty reward"},
)
@dataclass
class R1GRPOConfig(GRPOConfig):
"""
args for callbacks, benchmarks etc
"""
benchmarks: list[str] = field(
default_factory=lambda: [], metadata={"help": "The benchmarks to run after training."}
)
callbacks: list[str] = field(
default_factory=lambda: [], metadata={"help": "The callbacks to run during training."}
)
system_prompt: Optional[str] = field(
default=None, metadata={"help": "The optional system prompt to use for benchmarking."}
)
def main(script_args, training_args, model_args):
# Set seed for reproducibility
set_seed(training_args.seed)
###############
# Setup logging
###############
logging.basicConfig(
format="%(asctime)s - %(levelname)s - %(name)s - %(message)s",
datefmt="%Y-%m-%d %H:%M:%S",
handlers=[logging.StreamHandler(sys.stdout)],
)
log_level = training_args.get_process_log_level()
logger.setLevel(log_level)
datasets.utils.logging.set_verbosity(log_level)
transformers.utils.logging.set_verbosity(log_level)
transformers.utils.logging.enable_default_handler()
transformers.utils.logging.enable_explicit_format()
# Log on each process a small summary
logger.warning(
f"Process rank: {training_args.local_rank}, device: {training_args.device}, n_gpu: {training_args.n_gpu}"
+ f" distributed training: {bool(training_args.local_rank != -1)}, 16-bits training: {training_args.fp16}"
)
logger.info(f"Model parameters {model_args}")
logger.info(f"Script parameters {script_args}")
logger.info(f"Data parameters {training_args}")
# Check for last checkpoint
last_checkpoint = None
if os.path.isdir(training_args.output_dir):
last_checkpoint = get_last_checkpoint(training_args.output_dir)
logger.info(f"Last checkpoint detected, resuming training at {last_checkpoint=}.")
if last_checkpoint isnotNoneand training_args.resume_from_checkpoint isNone:
logger.info(f"Checkpoint detected, resuming training at {last_checkpoint=}.")
# Load the dataset
dataset = load_dataset(script_args.dataset_name, name=script_args.dataset_config)
# Get reward functions
REWARD_FUNCS_REGISTRY = {
"accuracy": accuracy_reward,
"format": format_reward,
"reasoning_steps": reasoning_steps_reward,
"cosine": get_cosine_scaled_reward(
min_value_wrnotallow=script_args.cosine_min_value_wrong,
max_value_wrnotallow=script_args.cosine_max_value_wrong,
min_value_correct=script_args.cosine_min_value_correct,
max_value_correct=script_args.cosine_max_value_correct,
max_len=script_args.cosine_max_len,
),
"repetition_penalty": get_repetition_penalty_reward(
ngram_size=script_args.repetition_n_grams,
max_penalty=script_args.repetition_max_penalty,
),
"length": len_reward,
}
reward_funcs = [REWARD_FUNCS_REGISTRY[func] for func in script_args.reward_funcs]
# Format into conversation
def make_conversation(example):
return {
"prompt": [
{"role": "system", "content": SYSTEM_PROMPT},
{"role": "user", "content": example["problem"]},
],
}
dataset = dataset.map(make_conversation)
for split in dataset:
if"messages"in dataset[split].column_names:
dataset[split] = dataset[split].remove_columns("messages")
logger.info("*** Initializing model kwargs ***")
torch_dtype = (
model_args.torch_dtype if model_args.torch_dtype in ["auto", None] else getattr(torch, model_args.torch_dtype)
)
training_args.gradient_checkpointing = True
model_kwargs = dict(
revision = model_args.model_revision,
trust_remote_code = model_args.trust_remote_code,
attn_implementation = model_args.attn_implementation,
torch_dtype = torch_dtype,
use_cache = Falseif training_args.gradient_checkpointing elseTrue,
)
model = AutoModelForCausalLM.from_pretrained(model_args.model_name_or_path,
load_in_4bit=False, **model_kwargs)
print(model_args.model_name_or_path)
#############################
# Initialize the R1GRPO trainer
#############################
trainer = GRPOTrainer(
model = model,
reward_funcs = reward_funcs,
args = training_args,
train_dataset = dataset[script_args.dataset_train_split],
eval_dataset = dataset[script_args.dataset_test_split] if training_args.eval_strategy != "no"elseNone,
peft_config = get_peft_config(model_args),
)
###############
# Training loop
###############
logger.info("*** Train ***")
checkpoint = None
if training_args.resume_from_checkpoint isnotNone:
checkpoint = training_args.resume_from_checkpoint
elif last_checkpoint isnotNone:
checkpoint = last_checkpoint
train_result = trainer.train(resume_from_checkpoint=checkpoint)
metrics = train_result.metrics
metrics["train_samples"] = len(dataset[script_args.dataset_train_split])
trainer.log_metrics("train", metrics)
trainer.save_metrics("train", metrics)
trainer.save_state()
##################################
# Save model and create model card
##################################
logger.info("*** Save model ***")
trainer.save_model(training_args.output_dir)
logger.info(f"Model saved to {training_args.output_dir}")
# Save everything else on main process
kwargs = {
"dataset_name": script_args.dataset_name,
"tags": ["GRPOTrainer-R1"],
}
if trainer.accelerator.is_main_process:
trainer.create_model_card(**kwargs)
# Restore k,v cache for fast inference
trainer.model.config.use_cache = True
trainer.model.config.save_pretrained(training_args.output_dir)
script_config = {
"dataset_name": "AI-MO/NuminaMath-TIR",
"dataset_config": "default",
"reward_funcs": [
"accuracy",
"format",
"reasoning_steps",
]
}
training_config = {
"output_dir": "output/GRPO-R1-1.5B", # 模型輸出目錄
"overwrite_output_dir": True, # 是否覆蓋輸出目錄
"do_eval": True, # 是否進(jìn)行評估
"eval_strategy": "steps", # 評估策略,按步數(shù)進(jìn)行評估
"eval_steps": 100, # 每100步進(jìn)行一次評估
"per_device_train_batch_size": 4, # 每個設(shè)備上的訓(xùn)練批次大小
"per_device_eval_batch_size": 4, # 每個設(shè)備上的評估批次大小
"gradient_accumulation_steps": 8, # 梯度累積步數(shù)
"learning_rate": 1.0e-06, # 學(xué)習(xí)率
"num_train_epochs": 1.0, # 訓(xùn)練的總輪數(shù)
"max_steps": -1, # 最大訓(xùn)練步數(shù),-1表示不限制
"lr_scheduler_type": "cosine", # 學(xué)習(xí)率調(diào)度器類型,使用余弦退火
"warmup_ratio": 0.1, # 預(yù)熱比例
"log_level": "info", # 日志記錄級別
"logging_strategy": "steps", # 日志記錄策略,按步數(shù)記錄
"logging_steps": 100, # 每100步記錄一次日志
"save_strategy": "no", # 保存策略,不保存
"seed": 42, # 隨機(jī)種子
"bf16": True, # 是否使用bfloat16精度
"gradient_checkpointing": True, # 是否使用梯度檢查點
"gradient_checkpointing_kwargs": {
"use_reentrant": False# 梯度檢查點的額外參數(shù),是否使用reentrant模式
},
"max_prompt_length": 128, # 最大提示長度
"num_generations": 4, # 生成的數(shù)量
"max_completion_length": 256, # 最大完成長度
"use_vllm": True, # 是否使用vLLM
"vllm_device": "auto", # vLLM設(shè)備,自動選擇
"vllm_gpu_memory_utilization": 0.8, # vLLM GPU內(nèi)存利用率
"resume_from_checkpoint": "output/GRPO-R1-1.5B", # 恢復(fù)檢查點,如果沒有l(wèi)atest文件,需要添加latest文件類似`global_step9055`
}
model_config = {
"model_name_or_path": "Qwen/Qwen2.5-1.5B-Instruct",
"model_revision": "main",
"torch_dtype": "bfloat16",
"attn_implementation": "flash_attention_2",
}
if __name__ == "__main__":
script_args = R1GRPOScriptArguments(**script_config)
training_args = R1GRPOConfig(**training_config)
model_args = ModelConfig(**model_config)
main(script_args, training_args, model_args)
參考
(1)??https://github.com/agentica-project/deepscaler??
(4)??https://github.com/hkust-nlp/simpleRL-reason??
(5)??https://mp.weixin.qq.com/s/RbQnInTa00ZISvJL7vORzA??
(6)?https://zhuanlan.zhihu.com/p/629644249??
本文轉(zhuǎn)載自??周末程序猿??,作者:周末程序猿
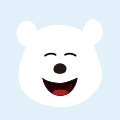