Agent卡成PPT?6 大核彈級提速方案+一線大廠代碼級拆解
第一定律:延遲溯源核彈
LangSmith 不只是監(jiān)控工具,更是性能法醫(yī)!看 Uber Eats 如何用它揪出「幽靈延遲」:
# 實戰(zhàn)代碼片段 - 延遲熱力圖生成
import logging
import langsmith
# 配置日志記錄
langsmith_logger = logging.getLogger("langsmith")
langsmith_logger.setLevel(level=logging.DEBUG)
# 性能指標(biāo)獲取 ? 可以獲取延遲相關(guān)指標(biāo)
# ? 包括:latency_p50、latency_p99、first_token_p50、first_token_p99
- 導(dǎo)出性能數(shù)據(jù)
- 使用 Pandas 處理數(shù)據(jù)
- 使用 Seaborn/Matplotlib 生成熱力圖
import pandas as pd
import seaborn as sns
import matplotlib.pyplot as plt
# 從 LangSmith 導(dǎo)出數(shù)據(jù)
data = client.list_runs(...)
df = pd.DataFrame(data)
# 生成熱力圖
plt.figure(figsize=(10, 6))
sns.heatmap(df[['latency', 'metadata']], cmap='YlOrRd')
plt.title('LangSmith 延遲熱力圖')
plt.show()
某電商平臺發(fā)現(xiàn) 73% 延遲來自「商品推薦工具」的重復(fù)調(diào)用? LinkedIn 借助瀑布流視圖優(yōu)化 RAG 流程,首字節(jié)時間降低 220ms
第二定律:認(rèn)知操控術(shù)
▎動態(tài)劇本殺式交互
Perplexity 的 CEO 揭秘:當(dāng)用戶看到這樣的動畫效果,等待焦慮下降 61%↓
// 前端實現(xiàn)偽代碼 - 多層級狀態(tài)流
function renderAgentThinking(step) {
const stages = ["?? 檢索知識庫", "?? 構(gòu)建推理鏈", "?? 調(diào)用支付API"];
stages.slice(0, step+1).forEach((text, index) => {
createFakeProgressBar(index, text);
});
}
▎后臺刺客模式Klarna 客服機(jī)器人的「隱身術(shù)」:
- 用戶提問 →即時返回「正在深度分析...」
- 異步執(zhí)行:知識檢索 → 風(fēng)險審核 → 多模態(tài)生成
- 最終通過 WebSocket 推送完整報告
第三定律:LLM 調(diào)用熔斷機(jī)制
架構(gòu)演進(jìn)圖
ReAct 到 LangGraph 的生死躍遷
- 傳統(tǒng)多代理困境:每個工具調(diào)用觸發(fā) 3-5 次 LLM
- LangGraph 的破局代碼:
from langgraph.graph import StateGraph, START, END
from typing import TypedDict
# 定義狀態(tài)類型
class State(TypedDict):
topic: str
joke: str
story: str
combined_output: str
# 定義并行的 LLM 調(diào)用函數(shù)
def call_llm_1(state: State):
"""第一個 LLM 調(diào)用生成笑話"""
msg = llm.invoke(f"Write a joke about {state['topic']}")
return {"joke": msg.content}
def call_llm_2(state: State):
"""第二個 LLM 調(diào)用生成故事"""
msg = llm.invoke(f"Write a story about {state['topic']}")
return {"story": msg.content}
def aggregator(state: State):
"""聚合所有生成的內(nèi)容"""
combined = f"Here's a story, joke, and poem about {state['topic']}!\n\n"
combined += f"STORY:\n{state['story']}\n\n"
combined += f"JOKE:\n{state['joke']}\n\n"
return {"combined_output": combined}
# 構(gòu)建工作流
parallel_builder = StateGraph(State)
# 添加節(jié)點
parallel_builder.add_node("call_llm_1", call_llm_1)
parallel_builder.add_node("call_llm_2", call_llm_2)
parallel_builder.add_node("aggregator", aggregator)
# 添加邊連接節(jié)點
parallel_builder.add_edge(START, "call_llm_1")
parallel_builder.add_edge(START, "call_llm_2")
parallel_builder.add_edge("call_llm_1", "aggregator")
parallel_builder.add_edge("call_llm_2", "aggregator")
parallel_builder.add_edge("aggregator", END)
# 編譯工作流
parallel_workflow = parallel_builder.compile()
# 調(diào)用工作流
state = parallel_workflow.invoke({"topic": "cats"})
print(state["combined_output"])
第四定律:并行宇宙引擎
LangGraph 的三大時空折疊術(shù)
1?? 預(yù)加載加速:在用戶輸入時提前加載工具參數(shù)
# 預(yù)加載代碼示例
prefetch_tools(["sentiment_analysis", "geoip_lookup"])
2?? 分支預(yù)測:根據(jù)歷史數(shù)據(jù)并行可能路徑
3?? 碎片聚合:將 10 個小文檔處理合并為 1 個批量請求
▎輸入瘦身秘籍
- 用 LlamaIndex 自動清理冗余上下文
- 動態(tài)上下文加載:某法律 SaaS 節(jié)省 40% 輸入 tokens
終極結(jié)語:速度即正義
當(dāng) Agent延遲從 9.2s → 1.4s 時,某大廠CTO 說:"這不是優(yōu)化,是商業(yè)模式的降維打擊!"
本文轉(zhuǎn)載自??AI小智??,作者:AI小智
已于2025-4-21 09:54:22修改
贊
收藏
回復(fù)
分享
微博
QQ
微信
舉報
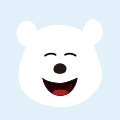
回復(fù)
相關(guān)推薦