Advanced RAG 07:在 RAG 系統(tǒng)中進(jìn)行表格數(shù)據(jù)處理的新思路 原創(chuàng) 精華
編者按: 目前,檢索增強(qiáng)生成(RAG)系統(tǒng)成為了將海量知識(shí)賦能于大模型的關(guān)鍵技術(shù)之一。然而,如何高效地處理半結(jié)構(gòu)化和非結(jié)構(gòu)化數(shù)據(jù),尤其是文檔中的表格數(shù)據(jù),仍然是 RAG 系統(tǒng)面臨的一大難題。
本文作者針對這一痛點(diǎn),提出了一種處理表格數(shù)據(jù)的新穎解決方案。作者首先系統(tǒng)性地梳理了RAG系統(tǒng)中表格處理的核心技術(shù),包括表格解析、索引結(jié)構(gòu)設(shè)計(jì)等,并評述了現(xiàn)有的一些開源解決方案。在此基礎(chǔ)上,作者提出了自己的創(chuàng)新之處——利用Nougat工具準(zhǔn)確高效地解析文檔中的表格內(nèi)容,使用語言模型對表格及其標(biāo)題進(jìn)行內(nèi)容摘要,最后構(gòu)建一種新型的document summary索引結(jié)構(gòu),并給出了完整的代碼實(shí)現(xiàn)細(xì)節(jié)。
這種方法的優(yōu)點(diǎn)是既能有效解析表格,又能全面考慮表格摘要與表格之間的關(guān)系,且無須使用多模態(tài) LLM ,能夠節(jié)省解析成本。讓我們拭目以待該方案在實(shí)踐中的進(jìn)一步應(yīng)用和發(fā)展。
作者 | Florian June
編譯 | 岳揚(yáng)
RAG 系統(tǒng)的實(shí)現(xiàn)是一項(xiàng)極具挑戰(zhàn)性的任務(wù),特別是需要解析和理解非結(jié)構(gòu)化文檔中的表格時(shí)。而對于經(jīng)過掃描操作數(shù)字化的文檔(scanned documents)或圖像格式的文檔(documents in image format)來說,實(shí)現(xiàn)這些操作就更加困難了。至少有三個(gè)方面的挑戰(zhàn):
- 經(jīng)過掃描操作數(shù)字化的文檔(scanned documents)或圖像格式的文檔(documents in image format)比較復(fù)雜,如文檔結(jié)構(gòu)的多樣性、文檔中可能包含一些非文本元素(non-text elements)以及文檔中可能同時(shí)存在手寫和印刷內(nèi)容,都會(huì)為表格信息的準(zhǔn)確自動(dòng)化提取帶來挑戰(zhàn)。不準(zhǔn)確的文檔解析會(huì)破壞表格結(jié)構(gòu),將不完整的表格信息轉(zhuǎn)換為向量表征(embedding)不僅無法有效捕捉表格的語義信息,還很容易導(dǎo)致 RAG 的最終輸出結(jié)果出現(xiàn)問題。
- 如何提取每個(gè)表格的標(biāo)題,如何將它們與對應(yīng)的那個(gè)具體表格關(guān)聯(lián)起來。
- 如何通過合理的索引結(jié)構(gòu)設(shè)計(jì),將表格中的關(guān)鍵語義信息高效組織和存儲(chǔ)起來。
本文首先介紹了如何在檢索增強(qiáng)生成(Retrieval Augmented Generation, RAG)模型中管理和處理表格數(shù)據(jù)。然后回顧了一些現(xiàn)有的開源解決方案,最后在當(dāng)前的技術(shù)基礎(chǔ)上,設(shè)計(jì)和實(shí)現(xiàn)了一種新穎的表格數(shù)據(jù)管理方法。
01 RAG表格數(shù)據(jù)相關(guān)核心技術(shù)介紹
1.1 Table Parsing 表格數(shù)據(jù)的解析
該模塊的主要功能是從非結(jié)構(gòu)化文檔或圖像中準(zhǔn)確提取表格結(jié)構(gòu)(table structure)。
附加需求: 最好能提取出相應(yīng)的表格標(biāo)題,方便開發(fā)人員將表格標(biāo)題與表格關(guān)聯(lián)起來。
根據(jù)我目前的理解,有以下幾種方法,如圖 1 所示:
圖 1:Table parser(表格解析器)。圖片由原文作者提供。
(a). 利用多模態(tài)LLM(如GPT-4V[1])識(shí)別表格,并從每個(gè) PDF 頁面中提取信息。
- 輸入:圖像格式的 PDF 頁面
- 輸出:JSON或其他格式的表格數(shù)據(jù)。如果多模態(tài) LLM 無法提取表格數(shù)據(jù),則應(yīng)對 PDF 圖像進(jìn)行總結(jié)并返回內(nèi)容摘要。
(b). 利用專業(yè)的表格檢測模型(如Table Transformer[2])來識(shí)別表格結(jié)構(gòu)。
- 輸入:PDF 頁面圖像
- 輸出:表格圖像
(c). 使用開源框架,如 unstructured[3] 或其他也采用目標(biāo)檢測模型(object detection models)的框架(這篇文章[4]詳細(xì)介紹了 unstructured 的表格檢測過程)。這些框架可以對整個(gè)文檔進(jìn)行全面解析,并從解析結(jié)果中提取與表格相關(guān)的內(nèi)容。
- 輸入:PDF或圖像格式的文檔
- 輸出:純文本或 HTML 格式的表格(從整個(gè)文檔的解析結(jié)果中獲得)
(d). 使用 Nougat[5]、Donut[6] 等端到端模型(end-to-end models),解析整個(gè)文檔并提取與表格相關(guān)的內(nèi)容。這種方法不需要 OCR 模型。
- 輸入:PDF 或圖像格式的文檔
- 輸出:LaTeX 或 JSON 格式的表格(從整個(gè)文檔的解析結(jié)果中獲得)
需要說明的是,無論使用哪種方法提取表格信息,都應(yīng)同時(shí)提取出表格標(biāo)題。因?yàn)樵诖蠖鄶?shù)情況下,表格標(biāo)題是文檔作者或論文作者對表格的簡要描述,可以在很大程度上概括整個(gè)表格的內(nèi)容。
在上述四種方法中,方法(d)可以較為方便地檢索表格標(biāo)題。 這對開發(fā)人員來說大有裨益,因?yàn)樗麄兛梢詫⒈砀駱?biāo)題與表格關(guān)聯(lián)起來。下面的實(shí)驗(yàn)將對此作進(jìn)一步說明。
1.2 Index Structure 如何索引表格數(shù)據(jù)
大致有以下幾類建立索引的方法:
(e). 只為圖像格式的表格建立索引。
(f). 只為純文本或JSON格式的表格建立索引。
(g). 只為LaTeX格式的表格建立索引。
(h). 只為表格的摘要建立索引。
(i). Small-to-big(譯者注:既包含細(xì)粒度索引,比如對每一行或表格摘要建立索引,也包含粗粒度索引,比如索引整個(gè)表格的圖像、純文本或 LaTeX 類型數(shù)據(jù),形成一種分層的、從小到大的索引結(jié)構(gòu)。) 或使用表格摘要建立索引結(jié)構(gòu),如圖2所示。
The content of the small chunk(譯者注:細(xì)粒度索引層級對應(yīng)的數(shù)據(jù)塊)比如將表格的每一行或摘要信息作為一個(gè)獨(dú)立的小數(shù)據(jù)塊。
The content of the big chunk(譯者注:粗粒度索引層級對應(yīng)的數(shù)據(jù)塊)可能是圖像格式、純文本格式或LaTeX格式的整個(gè)表格。
圖 2:以 small-to-big 的方式建立索引(上)和使用表格摘要建立索引(中、下)。圖片由原文作者提供。
如上所述,表格摘要通常是使用 LLM 處理生成的:
- 輸入:圖像格式、文本格式或 LaTeX 格式的表格
- 輸出:表格摘要
1.3 無需解析表格、建立索引或使用 RAG 技術(shù)的方法
有些算法不需要進(jìn)行表格數(shù)據(jù)的解析。
(j). 將相關(guān)圖像(PDF文檔頁)和用戶的 query 發(fā)送給 VQA 模型(如 DAN[7] 等)(譯者注:視覺問答(Visual Question Answering)模型的簡稱。是一種結(jié)合了計(jì)算機(jī)視覺和自然語言處理技術(shù)的模型,可用于回答關(guān)于圖像內(nèi)容的自然語言問題。)或多模態(tài) LLM,并返回答案。
- 要被索引的內(nèi)容: 圖像格式的文檔
- 發(fā)送給 VQA 模型或多模態(tài) LLM 的內(nèi)容:Query + 圖像形式的相應(yīng)文檔頁面
(k). 向 LLM 發(fā)送相關(guān)文本格式的 PDF 頁面和用戶的 query ,然后返回答案。
- 要被索引的內(nèi)容: 文本格式文檔
- 發(fā)送給 LLM 的內(nèi)容:Query + 文本格式的相應(yīng)文檔頁面
(l). 向多模態(tài) LLM(如 GPT-4V 等)發(fā)送相關(guān)文檔圖像(PDF 文檔頁面)、文本塊和用戶的 Query,然后直接返回答案。
- 要被索引的內(nèi)容: 圖像格式的文檔和文本格式的文檔塊(document chunks)
- 發(fā)送給多模態(tài) LLM 的內(nèi)容:Query + 相應(yīng)圖像格式的文檔 + 相應(yīng)文本塊(text chunks)
此外,下面還有一些不需要建立索引的方法,如圖 3 和圖 4 所示:
圖 3:Category (m)(譯者注:下面第一段介紹的內(nèi)容)。圖片由原文作者提供。
(m). 首先,使用(a)至(d)中的任何一種方法,將文檔中的所有表格解析為圖像形式。然后,將所有表格圖像和用戶的 query 直接發(fā)送給多模態(tài) LLM(如 GPT-4V 等),并返回答案。
- 要被索引的內(nèi)容: 無
- 發(fā)送給多模態(tài) LLM 的內(nèi)容:Query + 所有已經(jīng)轉(zhuǎn)換為圖像格式的表格
圖 4:Ctegory (n)(譯者注:下面第一段介紹的內(nèi)容)。圖片由原文作者提供。
(n). 使用通過(m)方法提取到的圖像格式的表格,然后使用 OCR 模型識(shí)別表格中的所有文本,然后直接將表格中的所有文本和用戶的 Query 發(fā)送到 LLM,并直接返回答案。
- 需要索引的內(nèi)容: 無
- 發(fā)送給 LLM 的內(nèi)容:用戶的 Query + 所有表格內(nèi)容(以文本格式發(fā)送)
值得注意的是,在處理文檔中的表格時(shí),有些方法并沒有使用 RAG(Retrieval-Augmented Generation)技術(shù):
- 第一類方法沒有使用 LLM,而是在特定數(shù)據(jù)集上進(jìn)行訓(xùn)練,使得 AI 模型(如基于 Transformer 架構(gòu)并受到 BERT 啟發(fā)的其他語言模型)來更好地支持表格理解任務(wù)的處理,比如 TAPAS [8]。
- 第二類方法是使用 LLM,采用預(yù)訓(xùn)練、微調(diào)方法或提示詞工程,使得 LLM 能夠完成表格理解任務(wù),如 GPT4Table[9]。
02 現(xiàn)有的表格處理開源解決方案
上一節(jié)總結(jié)并歸類了 RAG 系統(tǒng)中表格數(shù)據(jù)處理的關(guān)鍵技術(shù)。在提出本文要實(shí)現(xiàn)的解決方案之前,我們先來探索一些開源解決方案。
LlamaIndex 提出了四種方法[10],其中前三種均使用了多模態(tài)模型(multimodal models)。
- 檢索相關(guān)的PDF 頁面圖像并將其發(fā)送給 GPT-4V 響應(yīng)用戶 Query 。
- 將每個(gè) PDF 頁面均轉(zhuǎn)換為圖像格式,讓 GPT-4V 對每個(gè)頁面進(jìn)行圖像推理(image reasoning)。為圖像推理過程建立 Text Vector Store index(譯者注:將從圖像中推理出的文本信息轉(zhuǎn)換成向量形式,并建立索引),然后根據(jù) Image Reasoning Vector Store(譯者注:應(yīng)當(dāng)就是前面的索引,對前文建立的 Text Vector Store index 進(jìn)行查詢。) 查詢答案。
- 使用 Table Transformer 從檢索到的圖像中裁剪表格信息,然后將這些裁剪后的表格圖像發(fā)送到 GPT-4V 獲取 query responses (譯者注:向模型發(fā)送 Query 并獲取模型返回的答案)。
- 在裁剪后的表格圖像上應(yīng)用 OCR,并將數(shù)據(jù)發(fā)送給 GPT4/ GPT-3.5 來回答用戶的 query 。
總結(jié)一下上述四種方法:
- 第一種方法類似于本文中介紹的(j)方法,不需要進(jìn)行表格解析。但結(jié)果表明,即使答案就在圖像中,也無法產(chǎn)生正確答案。
- 第二種方法涉及到表格的解析,對應(yīng)于方法(a)。索引內(nèi)容可能是表格內(nèi)容或內(nèi)容摘要,完全取決于 GPT-4V 返回的結(jié)果,可能對應(yīng)于方法 (f) 或 (h)。這種方法的缺點(diǎn)是,GPT-4V 從文檔圖像中識(shí)別表格并提取其內(nèi)容的能力不穩(wěn)定,尤其是當(dāng)文檔圖像中包含表格、文本和其他圖像(在 PDF 文檔中這種情況很常見)時(shí)。
- 第三種方法與方法(m)類似,不需要編制索引。
- 第四種方法與方法(n)相似,也不需要編制索引。其結(jié)果表明,產(chǎn)生錯(cuò)誤答案的原因是無法從圖像中有效提取表格信息。
通過進(jìn)行測試發(fā)現(xiàn),第三種方法的整體效果最好。不過,根據(jù)我進(jìn)行的測試,第三種方法在檢測表格這方面就很吃力,更不用說正確提取并關(guān)聯(lián)合并表格標(biāo)題和表格內(nèi)容了。
Langchain 也對半結(jié)構(gòu)化數(shù)據(jù)的 RAG(Semi-structured RAG)[11] 技術(shù)提出了一些解決方案,核心技術(shù)包括:
- 使用 unstructured 進(jìn)行表格解析,這屬于(c)類方法。
- 索引方法是 document summary index (譯者注:將文檔摘要信息作為索引內(nèi)容),屬于(i)類方法。細(xì)粒度索引層級對應(yīng)的數(shù)據(jù)塊:表格摘要內(nèi)容,粗粒度索引層級對應(yīng)的數(shù)據(jù)塊:原始表格內(nèi)容(文本格式)。
如圖 5 所示:
圖 5 :Langchain 的 Semi-structured RAG 方案 。Source: Semi-structured RAG[11]
Semi-structured and Multi-modal RAG[12] 提出了三種解決方案,其架構(gòu)如圖 6 所示。
圖 6:Langchain 的 semi-structured and multi-modal RAG 方案。Source: Semi-structured and Multi-modal RAG[12].
Option 1 類似于前文的方法(l)。這種方法涉及使用多模態(tài)嵌入(multimodal embeddings)(如CLIP[13]),將圖像和文本轉(zhuǎn)換為嵌入向量,然后使用相似性搜索算法(similarity search)檢索兩者,并將未經(jīng)處理的圖像和文本數(shù)據(jù)傳遞給多模態(tài) LLM ,讓它們一起處理并生成問題答案。
Option 2 利用多模態(tài) LLM (如 GPT-4V[14] 、LLaVA[15] 或 FUYU-8b[16]),處理圖像生成文本摘要(text summaries)。然后,將文本數(shù)據(jù)轉(zhuǎn)換為嵌入向量,再使用這些向量來搜索或檢索與用戶提出的 Query 相匹配的文本內(nèi)容,并將這些文本內(nèi)容傳遞給 LLM 生成答案。
- 表格數(shù)據(jù)的解析使用 unstructured,屬于(d)類方法。
- 索引方法是 document summary index (譯者注:將文檔摘要信息作為索引內(nèi)容),屬于(i)類方法,細(xì)粒度索引層級對應(yīng)的數(shù)據(jù)塊:表格摘要內(nèi)容,粗粒度索引層級對應(yīng)的數(shù)據(jù)塊:文本格式的表格內(nèi)容。
Option 3 使用多模態(tài) LLM (如 GPT-4V[14] 、LLaVA[15] 或 FUYU-8b[16])從圖像數(shù)據(jù)中生成文本摘要,然后將這些文本摘要嵌入向量化,利用這些嵌入向量,可以對圖像摘要進(jìn)行高效檢索(retrieve),在檢索到的每個(gè)圖像摘要中,都保留有一個(gè)對應(yīng)的原始圖像的引用(reference to the raw image),這屬于上文的 (i) 類方法,最后將未經(jīng)處理的圖像數(shù)據(jù)和文本塊傳遞給多模態(tài) LLM 以便生成答案。
03 本文提出的解決方案
前文對關(guān)鍵技術(shù)和現(xiàn)有解決方案進(jìn)行了總結(jié)、分類和討論。在此基礎(chǔ)上,我們提出了以下解決方案,如圖 7 所示。為簡化起見,圖中省略了一些 RAG 模塊,如 Re-ranking 和 query rewriting 。
圖 7:本文提出的解決方案。圖片由原文作者提供。
- 表格解析技術(shù):使用 Nougat ( (d) 類方法)。根據(jù)我的測試,這種工具的表格檢測能力比 unstructured( (c) 類技術(shù))更有效。此外,Nougat 還能很好地提取表格標(biāo)題,非常方便與表格進(jìn)行關(guān)聯(lián)。
- 用于索引和檢索文檔摘要的索引結(jié)構(gòu)( (i) 類方法):細(xì)粒度索引層級對應(yīng)的內(nèi)容包括表格內(nèi)容摘要,粗粒度索引層級對應(yīng)的內(nèi)容包括 LaTeX 格式的相應(yīng)表格和文本格式的表格標(biāo)題。我們使用 multi-vector retriever[17] (譯者注:一種用于檢索文檔摘要索引中內(nèi)容的檢索器,該檢索器可以同時(shí)處理多個(gè)向量,以便有效地檢索與 Query 相關(guān)的文檔摘要。)來實(shí)現(xiàn)。
- 表格內(nèi)容摘要的獲取方法:將表格和表格標(biāo)題發(fā)送給 LLM 進(jìn)行內(nèi)容摘要。
這種方法的優(yōu)點(diǎn)是既能有效解析表格,又能全面考慮表格摘要與表格之間的關(guān)系。省去了使用多模態(tài) LLM 的需求,從而節(jié)省了成本。
3.1 Nougat 的工作原理
Nougat[18] 基于 Donut[19] 架構(gòu)開發(fā),這種方法使用的算法能夠在沒有任何與 OCR 相關(guān)的輸入或模塊的情況下,通過隱式的方式自動(dòng)識(shí)別文本。
圖 8 :遵循 Donut[19] 的端到端架構(gòu)(End-to-end architecture)。 Swin Transformer 編碼器接收文檔圖像并將其轉(zhuǎn)換為 latent embeddings (譯者注:在一個(gè)潛在空間中編碼了圖像的信息),然后以自回歸的方式將其轉(zhuǎn)換為一系列 tokens 。來源:Nougat: Neural Optical Understanding for Academic Documents.[18]
Nougat 在解析公式方面的能力令人印象深刻[20],但它解析表格的能力也非常出色。如圖 9 所示,它可以關(guān)聯(lián)表格標(biāo)題,非常方便:
圖 9 :Nougat 的運(yùn)行結(jié)果,結(jié)果文件為 Mathpix Markdown 格式(可通過 vscode 插件打開),表格以 LaTeX 格式呈現(xiàn)。
在我對十幾篇論文進(jìn)行的一項(xiàng)測試中,我發(fā)現(xiàn)表格標(biāo)題總是固定在表格的下一行。這種一致性表明這并非偶然。因此,我們比較感興趣 Nougat 是如何實(shí)現(xiàn)這種功能的。
鑒于這是一個(gè)缺乏中間結(jié)果的端到端模型,它的效果很可能嚴(yán)重依賴于其訓(xùn)練數(shù)據(jù)。
根據(jù)代碼分析,表格標(biāo)題部分的存儲(chǔ)位置和方式,似乎與訓(xùn)練數(shù)據(jù)中表格的組織格式是相符的(緊隨 ??\end{table}?
?? 之后就是 ??caption_parts?
? )。
def format_element(
element: Element, keep_refs: bool = False, latex_env: bool = False
) -> List[str]:
"""
Formats a given Element into a list of formatted strings.
Args:
element (Element): The element to be formatted.
keep_refs (bool, optional): Whether to keep references in the formatting. Default is False.
latex_env (bool, optional): Whether to use LaTeX environment formatting. Default is False.
Returns:
List[str]: A list of formatted strings representing the formatted element.
"""
...
...
if isinstance(element, Table):
parts = [
"[TABLE%s]\n\begin{table}\n"
% (str(uuid4())[:5] if element.id is None else ":" + str(element.id))
]
parts.extend(format_children(element, keep_refs, latex_env))
caption_parts = format_element(element.caption, keep_refs, latex_env)
remove_trailing_whitespace(caption_parts)
parts.append("\end{table}\n")
if len(caption_parts) > 0:
parts.extend(caption_parts + ["\n"])
parts.append("[ENDTABLE]\n\n")
return parts
...
...
3.2 Nougat 的優(yōu)點(diǎn)和缺點(diǎn)
優(yōu)點(diǎn):
- Nougat 可以將以前的解析工具難以解析的部分(如公式和表格)準(zhǔn)確地解析為 LaTeX 源代碼。
- Nougat 的解析結(jié)果是一種類似于 Markdown 的半結(jié)構(gòu)化文檔。
- 能夠輕松獲取表格標(biāo)題,并方便地與表格進(jìn)行關(guān)聯(lián)。
缺點(diǎn):
- Nougat 的解析速度較慢,這一點(diǎn)可能會(huì)在大規(guī)模應(yīng)用時(shí)造成困難。
- 由于 Nougat 的訓(xùn)練數(shù)據(jù)集基本上都是科學(xué)論文,因此在具有類似結(jié)構(gòu)的文檔上這種技術(shù)表現(xiàn)出色。而在處理非拉丁文本文檔時(shí),其性能就會(huì)下降。
- Nougat 模型每一次只訓(xùn)練一篇科學(xué)論文的一頁,缺乏對其他頁面的了解。這可能會(huì)導(dǎo)致解析內(nèi)容中存在一些前后不一致的現(xiàn)象。因此,如果識(shí)別效果不佳,可以考慮將 PDF 分成單獨(dú)的幾頁,然后逐頁進(jìn)行解析。
- 雙欄論文(two-column papers)中的表格解析不如單欄論文(single-column papers)的解析效果好。
3.3 代碼實(shí)現(xiàn)
首先,安裝相關(guān)的 Python 軟件包:
pip install langchain
pip install chromadb
pip install nougat-ocr
安裝完成后,需要檢查 Python 軟件包的版本:
langchain 0.1.12
langchain-community 0.0.28
langchain-core 0.1.31
langchain-openai 0.0.8
langchain-text-splitters 0.0.1
chroma-hnswlib 0.7.3
chromadb 0.4.24
nougat-ocr 0.1.17
建立工作環(huán)境并導(dǎo)入軟件包:
import os
os.environ["OPENAI_API_KEY"] = "YOUR_OPEN_AI_KEY"
import subprocess
import uuid
from langchain_core.output_parsers import StrOutputParser
from langchain_core.prompts import ChatPromptTemplate
from langchain_openai import ChatOpenAI
from langchain.retrievers.multi_vector import MultiVectorRetriever
from langchain.storage import InMemoryStore
from langchain_community.vectorstores import Chroma
from langchain_core.documents import Document
from langchain_openai import OpenAIEmbeddings
from langchain_core.runnables import RunnablePassthrough
將論文 《Attention Is All You Need》[21] 下載到路徑 ??YOUR_PDF_PATH?
?,運(yùn)行 nougat 來解析 PDF 文件,并從解析結(jié)果中獲取 latex 格式的表格數(shù)據(jù)和文本格式的表格標(biāo)題。第一次執(zhí)行該程序會(huì)下載必要的模型文件到本地環(huán)境。
def june_run_nougat(file_path, output_dir):
# Run Nougat and store results as Mathpix Markdown
cmd = ["nougat", file_path, "-o", output_dir, "-m", "0.1.0-base", "--no-skipping"]
res = subprocess.run(cmd)
if res.returncode != 0:
print("Error when running nougat.")
return res.returncode
else:
print("Operation Completed!")
return 0
def june_get_tables_from_mmd(mmd_path):
f = open(mmd_path)
lines = f.readlines()
res = []
tmp = []
flag = ""
for line in lines:
if line == "\begin{table}\n":
flag = "BEGINTABLE"
elif line == "\end{table}\n":
flag = "ENDTABLE"
if flag == "BEGINTABLE":
tmp.append(line)
elif flag == "ENDTABLE":
tmp.append(line)
flag = "CAPTION"
elif flag == "CAPTION":
tmp.append(line)
flag = "MARKDOWN"
print('-' * 100)
print(''.join(tmp))
res.append(''.join(tmp))
tmp = []
return res
file_path = "YOUR_PDF_PATH"
output_dir = "YOUR_OUTPUT_DIR_PATH"
if june_run_nougat(file_path, output_dir) == 1:
import sys
sys.exit(1)
mmd_path = output_dir + '/' + os.path.splitext(file_path)[0].split('/')[-1] + ".mmd"
tables = june_get_tables_from_mmd(mmd_path)
函數(shù) june_get_tables_from_mmd 用于從一個(gè) mmd 文件中提取所有內(nèi)容(從 ??\begin{table}?
?? 到 ??\end{table}?
??,還包括 ??\end{table}?
? 后的第一行),如圖10所示。
圖 10:Nougat 的運(yùn)行結(jié)果,結(jié)果文件為 Mathpix Markdown 格式(可通過 vscode 插件打開),解析出來的表格內(nèi)容為 latex 格式。函數(shù) june_get_tables_from_mmd 的功能是提取紅框中的表格信息。圖片由原文作者提供。
不過,目前還沒有官方文件規(guī)定表格標(biāo)題必須放在表格下方,或者表格應(yīng)以 \begin{table} 開始,以 \end{table} 結(jié)束。因此,june_get_tables_from_mmd 是一種啟發(fā)式的方法(heuristic)。
以下是對 PDF 文檔的表格解析結(jié)果:
Operation Completed!
----------------------------------------------------------------------------------------------------
\begin{table}
\begin{tabular}{l c c c} \hline \hline Layer Type & Complexity per Layer & Sequential Operations & Maximum Path Length \ \hline Self-Attention & (O(n^{2}\cdot d)) & (O(1)) & (O(1)) \ Recurrent & (O(n\cdot d^{2})) & (O(n)) & (O(n)) \ Convolutional & (O(k\cdot n\cdot d^{2})) & (O(1)) & (O(log_{k}(n))) \ Self-Attention (restricted) & (O(r\cdot n\cdot d)) & (O(1)) & (O(n/r)) \ \hline \hline \end{tabular}
\end{table}
Table 1: Maximum path lengths, per-layer complexity and minimum number of sequential operations for different layer types. (n) is the sequence length, (d) is the representation dimension, (k) is the kernel size of convolutions and (r) the size of the neighborhood in restricted self-attention.
----------------------------------------------------------------------------------------------------
\begin{table}
\begin{tabular}{l c c c c} \hline \hline \multirow{2}{*}{Model} & \multicolumn{2}{c}{BLEU} & \multicolumn{2}{c}{Training Cost (FLOPs)} \ \cline{2-5} & EN-DE & EN-FR & EN-DE & EN-FR \ \hline ByteNet [18] & 23.75 & & & \ Deep-Att + PosUnk [39] & & 39.2 & & (1.0\cdot 10^{20}) \ GNMT + RL [38] & 24.6 & 39.92 & (2.3\cdot 10^{19}) & (1.4\cdot 10^{20}) \ ConvS2S [9] & 25.16 & 40.46 & (9.6\cdot 10^{18}) & (1.5\cdot 10^{20}) \ MoE [32] & 26.03 & 40.56 & (2.0\cdot 10^{19}) & (1.2\cdot 10^{20}) \ \hline Deep-Att + PosUnk Ensemble [39] & & 40.4 & & (8.0\cdot 10^{20}) \ GNMT + RL Ensemble [38] & 26.30 & 41.16 & (1.8\cdot 10^{20}) & (1.1\cdot 10^{21}) \ ConvS2S Ensemble [9] & 26.36 & **41.29** & (7.7\cdot 10^{19}) & (1.2\cdot 10^{21}) \ \hline Transformer (base model) & 27.3 & 38.1 & & (\mathbf{3.3\cdot 10^{18}}) \ Transformer (big) & **28.4** & **41.8** & & (2.3\cdot 10^{19}) \ \hline \hline \end{tabular}
\end{table}
Table 2: The Transformer achieves better BLEU scores than previous state-of-the-art models on the English-to-German and English-to-French newstest2014 tests at a fraction of the training cost.
----------------------------------------------------------------------------------------------------
\begin{table}
\begin{tabular}{c|c c c c c c c c|c c c c} \hline \hline & (N) & (d_{\text{model}}) & (d_{\text{ff}}) & (h) & (d_{k}) & (d_{v}) & (P_{drop}) & (\epsilon_{ls}) & train steps & PPL & BLEU & params \ \hline base & 6 & 512 & 2048 & 8 & 64 & 64 & 0.1 & 0.1 & 100K & 4.92 & 25.8 & 65 \ \hline \multirow{4}{*}{(A)} & \multicolumn{1}{c}{} & & 1 & 512 & 512 & & & & 5.29 & 24.9 & \ & & & & 4 & 128 & 128 & & & & 5.00 & 25.5 & \ & & & & 16 & 32 & 32 & & & & 4.91 & 25.8 & \ & & & & 32 & 16 & 16 & & & & 5.01 & 25.4 & \ \hline (B) & \multicolumn{1}{c}{} & & \multicolumn{1}{c}{} & & 16 & & & & & 5.16 & 25.1 & 58 \ & & & & & 32 & & & & & 5.01 & 25.4 & 60 \ \hline \multirow{4}{*}{(C)} & 2 & \multicolumn{1}{c}{} & & & & & & & & 6.11 & 23.7 & 36 \ & 4 & & & & & & & & 5.19 & 25.3 & 50 \ & 8 & & & & & & & & 4.88 & 25.5 & 80 \ & & 256 & & 32 & 32 & & & & 5.75 & 24.5 & 28 \ & 1024 & & 128 & 128 & & & & 4.66 & 26.0 & 168 \ & & 1024 & & & & & & 5.12 & 25.4 & 53 \ & & 4096 & & & & & & 4.75 & 26.2 & 90 \ \hline \multirow{4}{*}{(D)} & \multicolumn{1}{c}{} & & & & & 0.0 & & 5.77 & 24.6 & \ & & & & & & 0.2 & & 4.95 & 25.5 & \ & & & & & & & 0.0 & 4.67 & 25.3 & \ & & & & & & & 0.2 & 5.47 & 25.7 & \ \hline (E) & \multicolumn{1}{c}{} & \multicolumn{1}{c}{} & & \multicolumn{1}{c}{} & & & & & 4.92 & 25.7 & \ \hline big & 6 & 1024 & 4096 & 16 & & 0.3 & 300K & **4.33** & **26.4** & 213 \ \hline \hline \end{tabular}
\end{table}
Table 3: Variations on the Transformer architecture. Unlisted values are identical to those of the base model. All metrics are on the English-to-German translation development set, newstest2013. Listed perplexities are per-wordpiece, according to our byte-pair encoding, and should not be compared to per-word perplexities.
----------------------------------------------------------------------------------------------------
\begin{table}
\begin{tabular}{c|c|c} \hline
**Parser** & **Training** & **WSJ 23 F1** \ \hline Vinyals & Kaiser et al. (2014) [37] & WSJ only, discriminative & 88.3 \ Petrov et al. (2006) [29] & WSJ only, discriminative & 90.4 \ Zhu et al. (2013) [40] & WSJ only, discriminative & 90.4 \ Dyer et al. (2016) [8] & WSJ only, discriminative & 91.7 \ \hline Transformer (4 layers) & WSJ only, discriminative & 91.3 \ \hline Zhu et al. (2013) [40] & semi-supervised & 91.3 \ Huang & Harper (2009) [14] & semi-supervised & 91.3 \ McClosky et al. (2006) [26] & semi-supervised & 92.1 \ Vinyals & Kaiser el al. (2014) [37] & semi-supervised & 92.1 \ \hline Transformer (4 layers) & semi-supervised & 92.7 \ \hline Luong et al. (2015) [23] & multi-task & 93.0 \ Dyer et al. (2016) [8] & generative & 93.3 \ \hline \end{tabular}
\end{table}
Table 4: The Transformer generalizes well to English constituency parsing (Results are on Section 23 of WSJ)* [5] Kyunghyun Cho, Bart van Merrienboer, Caglar Gulcehre, Fethi Bougares, Holger Schwenk, and Yoshua Bengio. Learning phrase representations using rnn encoder-decoder for statistical machine translation. _CoRR_, abs/1406.1078, 2014.
然后使用 LLM 對表格數(shù)據(jù)進(jìn)行總結(jié):
# Prompt
prompt_text = """You are an assistant tasked with summarizing tables and text. \
Give a concise summary of the table or text. The table is formatted in LaTeX, and its caption is in plain text format: {element} """
prompt = ChatPromptTemplate.from_template(prompt_text)
# Summary chain
model = ChatOpenAI(temperature = 0, model = "gpt-3.5-turbo")
summarize_chain = {"element": lambda x: x} | prompt | model | StrOutputParser()
# Get table summaries
table_summaries = summarize_chain.batch(tables, {"max_concurrency": 5})
print(table_summaries)
以下是對《Attention Is All You Need》[21]中四個(gè)表格的內(nèi)容摘要,如圖11所示:
圖 11:《Attention Is All You Need》[21]中四個(gè)表格的內(nèi)容摘要。
使用 Multi-Vector Retriever (譯者注:一種用于檢索文檔摘要索引中內(nèi)容的檢索器,該檢索器可以同時(shí)處理多個(gè)向量,以便有效地檢索與 Query 相關(guān)的文檔摘要。)構(gòu)建 document summary index structure [17](譯者注:一種索引結(jié)構(gòu),用于存儲(chǔ)文檔的摘要信息,并可根據(jù)需要檢索或查詢這些摘要信息)。
# The vectorstore to use to index the child chunks
vectorstore = Chroma(collection_name = "summaries", embedding_function = OpenAIEmbeddings())
# The storage layer for the parent documents
store = InMemoryStore()
id_key = "doc_id"
# The retriever (empty to start)
retriever = MultiVectorRetriever(
vectorstore = vectorstore,
docstore = store,
id_key = id_key,
search_kwargs={"k": 1} # Solving Number of requested results 4 is greater than number of elements in index..., updating n_results = 1
)
# Add tables
table_ids = [str(uuid.uuid4()) for _ in tables]
summary_tables = [
Document(page_content = s, metadata = {id_key: table_ids[i]})
for i, s in enumerate(table_summaries)
]
retriever.vectorstore.add_documents(summary_tables)
retriever.docstore.mset(list(zip(table_ids, tables)))
一切準(zhǔn)備就緒后,建立一個(gè)簡單的 RAG pipeline 并執(zhí)行用戶的 queries :
# Prompt template
template = """Answer the question based only on the following context, which can include text and tables, there is a table in LaTeX format and a table caption in plain text format:
{context}
Question: {question}
"""
prompt = ChatPromptTemplate.from_template(template)
# LLM
model = ChatOpenAI(temperature = 0, model = "gpt-3.5-turbo")
# Simple RAG pipeline
chain = (
{"context": retriever, "question": RunnablePassthrough()}
| prompt
| model
| StrOutputParser()
)
print(chain.invoke("when layer type is Self-Attention, what is the Complexity per Layer?")) # Query about table 1
print(chain.invoke("Which parser performs worst for BLEU EN-DE")) # Query about table 2
print(chain.invoke("Which parser performs best for WSJ 23 F1")) # Query about table 4
運(yùn)行結(jié)果如下,這幾個(gè)問題都得到了準(zhǔn)確的回答,如圖 12 所示:
圖 12 :對三個(gè)用戶 queries 的回答結(jié)果。第一行對應(yīng)于《Attention Is All You Need》中的表 1,第二行對應(yīng)于表 2,第三行對應(yīng)于表 4。
總體代碼如下:
import os
os.environ["OPENAI_API_KEY"] = "YOUR_OPEN_AI_KEY"
import subprocess
import uuid
from langchain_core.output_parsers import StrOutputParser
from langchain_core.prompts import ChatPromptTemplate
from langchain_openai import ChatOpenAI
from langchain.retrievers.multi_vector import MultiVectorRetriever
from langchain.storage import InMemoryStore
from langchain_community.vectorstores import Chroma
from langchain_core.documents import Document
from langchain_openai import OpenAIEmbeddings
from langchain_core.runnables import RunnablePassthrough
def june_run_nougat(file_path, output_dir):
# Run Nougat and store results as Mathpix Markdown
cmd = ["nougat", file_path, "-o", output_dir, "-m", "0.1.0-base", "--no-skipping"]
res = subprocess.run(cmd)
if res.returncode != 0:
print("Error when running nougat.")
return res.returncode
else:
print("Operation Completed!")
return 0
def june_get_tables_from_mmd(mmd_path):
f = open(mmd_path)
lines = f.readlines()
res = []
tmp = []
flag = ""
for line in lines:
if line == "\begin{table}\n":
flag = "BEGINTABLE"
elif line == "\end{table}\n":
flag = "ENDTABLE"
if flag == "BEGINTABLE":
tmp.append(line)
elif flag == "ENDTABLE":
tmp.append(line)
flag = "CAPTION"
elif flag == "CAPTION":
tmp.append(line)
flag = "MARKDOWN"
print('-' * 100)
print(''.join(tmp))
res.append(''.join(tmp))
tmp = []
return res
file_path = "YOUR_PDF_PATH"
output_dir = "YOUR_OUTPUT_DIR_PATH"
if june_run_nougat(file_path, output_dir) == 1:
import sys
sys.exit(1)
mmd_path = output_dir + '/' + os.path.splitext(file_path)[0].split('/')[-1] + ".mmd"
tables = june_get_tables_from_mmd(mmd_path)
# Prompt
prompt_text = """You are an assistant tasked with summarizing tables and text. \
Give a concise summary of the table or text. The table is formatted in LaTeX, and its caption is in plain text format: {element} """
prompt = ChatPromptTemplate.from_template(prompt_text)
# Summary chain
model = ChatOpenAI(temperature = 0, model = "gpt-3.5-turbo")
summarize_chain = {"element": lambda x: x} | prompt | model | StrOutputParser()
# Get table summaries
table_summaries = summarize_chain.batch(tables, {"max_concurrency": 5})
print(table_summaries)
# The vectorstore to use to index the child chunks
vectorstore = Chroma(collection_name = "summaries", embedding_function = OpenAIEmbeddings())
# The storage layer for the parent documents
store = InMemoryStore()
id_key = "doc_id"
# The retriever (empty to start)
retriever = MultiVectorRetriever(
vectorstore = vectorstore,
docstore = store,
id_key = id_key,
search_kwargs={"k": 1} # Solving Number of requested results 4 is greater than number of elements in index..., updating n_results = 1
)
# Add tables
table_ids = [str(uuid.uuid4()) for _ in tables]
summary_tables = [
Document(page_content = s, metadata = {id_key: table_ids[i]})
for i, s in enumerate(table_summaries)
]
retriever.vectorstore.add_documents(summary_tables)
retriever.docstore.mset(list(zip(table_ids, tables)))
# Prompt template
template = """Answer the question based only on the following context, which can include text and tables, there is a table in LaTeX format and a table caption in plain text format:
{context}
Question: {question}
"""
prompt = ChatPromptTemplate.from_template(template)
# LLM
model = ChatOpenAI(temperature = 0, model = "gpt-3.5-turbo")
# Simple RAG pipeline
chain = (
{"context": retriever, "question": RunnablePassthrough()}
| prompt
| model
| StrOutputParser()
)
print(chain.invoke("when layer type is Self-Attention, what is the Complexity per Layer?")) # Query about table 1
print(chain.invoke("Which parser performs worst for BLEU EN-DE")) # Query about table 2
print(chain.invoke("Which parser performs best for WSJ 23 F1")) # Query about table 4
04 Conclusion
本文討論了在 RAG 系統(tǒng)中表格處理操作的關(guān)鍵技術(shù)和現(xiàn)有解決方案,并提出了一種解決方案及其實(shí)現(xiàn)方法。
在本文中,我們使用了 Nougat 來解析表格。不過,如果有更快、更有效的解析工具可用,我們會(huì)考慮替換掉 Nougat 。我們對工具的態(tài)度是先有正確的 idea ,然后再找工具來實(shí)現(xiàn)它,而不是依賴于某個(gè)工具。
在本文中,我們將所有表格內(nèi)容輸入到 LLM 。但是,在實(shí)際場景中,我們需要考慮到表格大小超出 LLM 上下文長度的情況。我們可以通過使用有效的分塊(chunking)方法來解決這個(gè)問題。
Thanks for reading!
——
Florian June
An artificial intelligence researcher, mainly write articles about Large Language Models, data structures and algorithms, and NLP.
END
參考資料
[1]??https://openai.com/research/gpt-4v-system-card??
[2]??https://github.com/microsoft/table-transformer??
[3]??https://unstructured-io.github.io/unstructured/best_practices/table_extraction_pdf.html??
[4]??https://pub.towardsai.net/advanced-rag-02-unveiling-pdf-parsing-b84ae866344e??
[5]??https://github.com/facebookresearch/nougat??
[6]??https://github.com/clovaai/donut/??
[7]??https://arxiv.org/pdf/1611.00471.pdf??
[8]??https://aclanthology.org/2020.acl-main.398.pdf??
[9]??https://arxiv.org/pdf/2305.13062.pdf??
[10]??https://docs.llamaindex.ai/en/stable/examples/multi_modal/multi_modal_pdf_tables.html??
[13]??https://openai.com/research/clip??
[14]??https://openai.com/research/gpt-4v-system-card??
[15]??https://llava.hliu.cc/??
[16]??https://www.adept.ai/blog/fuyu-8b??
[17]??https://python.langchain.com/docs/modules/data_connection/retrievers/multi_vector??
[18]??https://arxiv.org/pdf/2308.13418.pdf??
[19]??https://arxiv.org/pdf/2111.15664.pdf??
[21]??https://arxiv.org/pdf/1706.03762.pdf??
本文經(jīng)原作者授權(quán),由 Baihai IDP 編譯。如需轉(zhuǎn)載譯文,請聯(lián)系獲取授權(quán)。
原文鏈接:
??https://ai.plainenglish.io/advanced-rag-07-exploring-rag-for-tables-5c3fc0de7af6??
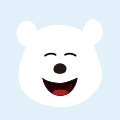