LangGraph實(shí)戰(zhàn):可控的AI航空客服助手
當(dāng)智能助手代表用戶執(zhí)行操作時(shí),用戶幾乎總是應(yīng)該對是否執(zhí)行這些操作擁有最終決定權(quán)。否則,即使是智能助手的一點(diǎn)小失誤,或是它未能抵御的任何指令注入,都可能對用戶造成實(shí)際損害。
在這部分,我們將利用LangGraph的interrupt_before功能,在執(zhí)行任何工具之前,暫停流程并把控制權(quán)交還給用戶。
您的流程圖可能看起來像這樣:
流程圖示例
和之前一樣,我們首先定義狀態(tài):
狀態(tài)與智能助手
我們的流程圖狀態(tài)和LLM調(diào)用與第一部分基本相同,除了:
- 我們新增了一個(gè)user_info字段,它將由我們的流程圖主動填充
- 我們可以在Assistant對象中直接使用狀態(tài),而不是使用可配置的參數(shù)
from typing import Annotated
from langchain_anthropic import ChatAnthropic
from langchain_community.tools.tavily_search import TavilySearchResults
from langchain_core.prompts import ChatPromptTemplate
from langchain_core.runnables import Runnable, RunnableConfig
from typing_extensions import TypedDict
from langgraph.graph.message import AnyMessage, add_messages
class State(TypedDict):
messages: Annotated[list[AnyMessage], add_messages]
user_info: str
class Assistant:
def __init__(self, runnable: Runnable):
self.runnable = runnable
def __call__(self, state: State, config: RunnableConfig):
while True:
passenger_id = config.get("passenger_id", None)
result = self.runnable.invoke(state)
# 如果大型語言模型恰好返回了一個(gè)空響應(yīng),我們將重新請求一個(gè)實(shí)際的響應(yīng)。
if not result.tool_calls and (
not result.content
or isinstance(result.content, list)
and not result.content[0].get("text")
):
messages = state["messages"] + [("user", "請給出真實(shí)的輸出。")]
state = {**state, "messages": messages}
else:
break
return {"messages": result}
# Haiku模型更快更經(jīng)濟(jì),但準(zhǔn)確性較低
# llm = ChatAnthropic(model="claude-3-haiku-20240307")
llm = ChatAnthropic(model="claude-3-sonnet-20240229", temperature=1)
# 你也可以使用OpenAI或其他模型,盡管你可能需要調(diào)整提示
# from langchain_openai import ChatOpenAI
# llm = ChatOpenAI(model="gpt-4-turbo-preview")
assistant_prompt = ChatPromptTemplate.from_messages(
[
(
"system",
"你是一個(gè)樂于助人的瑞士航空客戶支持智能助手。"
"利用提供的工具搜索航班、公司政策和其他信息,以幫助解答用戶的疑問。"
"在搜索時(shí),要持之以恒。如果首次搜索沒有結(jié)果,就擴(kuò)大你的搜索范圍。"
"如果搜索依然一無所獲,繼續(xù)擴(kuò)大搜索范圍,不要輕言放棄。"
"\n\n當(dāng)前用戶:\n<User>\n{user_info}\n</User>"
"\n當(dāng)前時(shí)間:{time}。",
),
("placeholder", "{messages}"),
]
).partial(time=datetime.now())
part_2_tools = [
TavilySearchResults(max_results=1),
fetch_user_flight_information,
search_flights,
lookup_policy,
update_ticket_to_new_flight,
cancel_ticket,
search_car_rentals,
book_car_rental,
update_car_rental,
cancel_car_rental,
search_hotels,
book_hotel,
update_hotel,
cancel_hotel,
search_trip_recommendations,
book_excursion,
update_excursion,
cancel_excursion,
]
part_2_assistant_runnable = assistant_prompt | llm.bind_tools(part_2_tools)
定義流程圖
現(xiàn)在,創(chuàng)建流程圖。根據(jù)第一部分的反饋,我們做出兩個(gè)改變:
- 在使用工具之前加入一個(gè)中斷點(diǎn)。
- 在第一個(gè)節(jié)點(diǎn)中明確填充用戶狀態(tài),這樣智能助手就不必通過使用工具來了解用戶信息。
from langgraph.checkpoint.sqlite import SqliteSaver
from langgraph.graph import END, StateGraph
from langgraph.prebuilt import ToolNode, tools_condition
builder = StateGraph(State)
def user_info(state: State):
return {"user_info": fetch_user_flight_information.invoke({})}
# 新增:fetch_user_info節(jié)點(diǎn)首先執(zhí)行,這意味著我們的智能助手可以在
# 不采取任何行動的情況下查看用戶的航班信息
builder.add_node("fetch_user_info", user_info)
builder.set_entry_point("fetch_user_info")
builder.add_node("assistant", Assistant(part_2_assistant_runnable))
builder.add_node("action", create_tool_node_with_fallback(part_2_tools))
builder.add_edge("fetch_user_info", "assistant")
builder.add_conditional_edges(
"assistant", tools_condition, {"action": "action", END: END}
)
builder.add_edge("action", "assistant")
memory = SqliteSaver.from_conn_string(":memory:")
part_2_graph = builder.compile(
checkpointer=memory,
# 新增:流程圖在執(zhí)行“action”節(jié)點(diǎn)之前總是暫停。
# 用戶可以在智能助手繼續(xù)之前批準(zhǔn)或拒絕(甚至修改請求)
interrupt_before=["action"],
)
from IPython.display import Image, display
try:
display(Image(part_2_graph.get_graph(xray=True).draw_mermaid_png()))
except:
# 這需要一些額外的依賴項(xiàng),并且是可選的
pass
流程圖示例2
示例對話
現(xiàn)在,讓我們通過以下對話示例來測試我們新修訂的聊天機(jī)器人。
import shutil
import uuid
# 使用備份文件更新,以便我們可以從每個(gè)部分的起始點(diǎn)重新啟動
shutil.copy(backup_file, db)
thread_id = str(uuid.uuid4())
config = {
"configurable": {
# passenger_id在我們的航班工具中使用,以獲取用戶的航班信息
"passenger_id": "3442 587242",
# 通過thread_id訪問檢查點(diǎn)
"thread_id": thread_id,
}
}
_printed = set()
# 我們可以重復(fù)使用第一部分的教程問題,以觀察聊天機(jī)器人的表現(xiàn)。
for question in tutorial_questions:
events = part_2_graph.stream(
{"messages": ("user", question)}, config, stream_mode="values"
)
for event in events:
_print_event(event, _printed)
snapshot = part_2_graph.get_state(config)
while snapshot.next:
# 我們遇到了一個(gè)中斷!代理正試圖使用一個(gè)工具,而用戶可以批準(zhǔn)或拒絕它
# 注意:這段代碼位于你的流程圖之外。通常,你會將輸出流式傳輸?shù)接脩艚缑妗? # 然后,你會在用戶輸入時(shí),通過API調(diào)用觸發(fā)一個(gè)新的運(yùn)行。
user_input = input(
"你同意上述操作嗎?輸入'y'以繼續(xù);"
"否則,請說明你請求的更改。\n\n"
)
if user_input.strip() == "y":
# 繼續(xù)執(zhí)行
result = part_2_graph.invoke(
None,
config,
)
else:
# 通過提供關(guān)于請求更改/改變主意的說明,滿足工具調(diào)用
result = part_2_graph.invoke(
{
"messages": [
ToolMessage(
tool_call_id=event["messages"][-1].tool_calls[0]["id"],
cnotallow=f"API調(diào)用被用戶拒絕。理由:'{user_input}'. 繼續(xù)協(xié)助,考慮用戶的輸入。",
)
]
},
config,
)
snapshot = part_2_graph.get_state(config)
第二部分回顧
現(xiàn)在,我們的智能助手能夠節(jié)省一步來響應(yīng)我們的航班詳情。我們還完全控制了執(zhí)行的操作。這一切都是通過LangGraph的interrupts和checkpointers實(shí)現(xiàn)的。中斷暫停了流程圖的執(zhí)行,其狀態(tài)使用配置的檢查點(diǎn)器安全地持久化。用戶隨后可以在任何時(shí)候通過使用正確的配置運(yùn)行它來啟動它。
查看一個(gè)LangSmith示例跟蹤,以更好地理解流程圖是如何運(yùn)行的。注意從這個(gè)跟蹤中,你通常通過使用(None, config)調(diào)用流程圖來恢復(fù)流程。狀態(tài)從檢查點(diǎn)加載,就像它從未被中斷過一樣。
這個(gè)流程圖工作得很好!但當(dāng)需要我們參與每一個(gè)智能助手的行動的要求,十分影響使用體驗(yàn),并且助手在執(zhí)行查詢等動作時(shí)并不會影響實(shí)際業(yè)務(wù)。
本文轉(zhuǎn)載自?? AI小智??,作者: AI小智
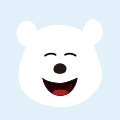