5W字長文 Agent多智能體探秘:架構(gòu)設(shè)計、交互模式與應(yīng)用實踐深度剖析 精華
一、引言
在人工智能領(lǐng)域,代理是一類借助大語言模型(LLM)來決定應(yīng)用程序控制流的系統(tǒng)。隨著開發(fā)的推進,這類系統(tǒng)往往會變得愈發(fā)復(fù)雜,給管理和擴展帶來諸多難題。比如,你可能會遭遇以下狀況:
- 工具選擇困境:代理可調(diào)用的工具繁多,導(dǎo)致在決策下一步使用哪個工具時表現(xiàn)欠佳。
- 上下文管理難題:上下文信息過于繁雜,單個代理難以有效追蹤和處理。
- 專業(yè)領(lǐng)域需求多樣:系統(tǒng)內(nèi)需要涵蓋多個專業(yè)領(lǐng)域,像規(guī)劃師、研究員、數(shù)學(xué)專家等角色。
為化解這些問題,一種有效的策略是把應(yīng)用程序拆分成多個較小的獨立代理,進而組合成多代理系統(tǒng)。這些獨立代理的復(fù)雜程度差異較大,簡單的可能僅涉及一個提示和一次LLM調(diào)用,復(fù)雜的則可能像ReAct代理那般(甚至更復(fù)雜?。?/p>
隨著代理框架的蓬勃發(fā)展,眾多公司紛紛構(gòu)建自己的多代理系統(tǒng),期望找到解決所有代理任務(wù)的通用方案。兩年前,研究人員設(shè)計出一款名為ChatDev的多代理協(xié)作系統(tǒng)。ChatDev宛如一家虛擬軟件公司,通過各類智能代理來運作,這些代理分別承擔(dān)著首席執(zhí)行官、首席產(chǎn)品官、美工、編碼器、審閱者、測試人員等不同角色,與常規(guī)的軟件工程公司極為相似。
所有這些代理協(xié)同作業(yè)、相互通信,成功開發(fā)出一款視頻游戲。這一成果讓不少人認為,任何軟件工程任務(wù)都能借助這種多代理架構(gòu)完成,其中每個AI都有著獨特的分工。然而,實際的實驗表明,并非所有問題都能靠同一架構(gòu)解決。在某些情形下,更為簡單的架構(gòu)反而能提供更高效、成本效益更好的解決方案。
1.1 單代理與多代理架構(gòu)
起初,單代理方法看似可行(即一個人工智能代理能處理從瀏覽器導(dǎo)航到文件操作等所有事務(wù))。但隨著時間推移,任務(wù)復(fù)雜度攀升,工具數(shù)量增多,單代理模式便開始面臨挑戰(zhàn)。
當代理出現(xiàn)異常行為時,我們會察覺到一些影響,這可能源于以下因素:
- 工具過載:工具數(shù)量過多,使得代理在選擇工具及其使用時機時陷入困惑。
- 上下文臃腫:代理的上下文窗口不斷擴大,其中包含的工具數(shù)量過多。
- 錯誤頻發(fā):由于職責(zé)范圍過寬,代理開始產(chǎn)生次優(yōu)甚至錯誤的結(jié)果。
當我們著手自動化多個不同的子任務(wù)(如數(shù)據(jù)提取、報告生成)時,或許就該考慮劃分職責(zé)了。通過采用多個AI代理,每個代理專注于自身的領(lǐng)域和工具包,這樣不僅能提升解決方案的清晰度和質(zhì)量,還能簡化代理的開發(fā)流程。
二、多智能體架構(gòu)
正如您所看到的,單代理和多代理架構(gòu)既有優(yōu)勢也有劣勢。當任務(wù)簡單且定義明確,并且沒有特定資源限制時,單代理架構(gòu)是理想之選。另一方面,當用例復(fù)雜且動態(tài),需要更專業(yè)的知識和協(xié)作,或者具有可擴展性和適應(yīng)性要求時,多代理架構(gòu)則能發(fā)揮很大的作用。
2.1 多智能體系統(tǒng)中的模式
在多代理系統(tǒng)中,連接代理的方式有好幾種:
2.1.1 并行
多個代理同時處理任務(wù)的不同部分。
示例:我們希望使用3個代理同時對給定文本進行總結(jié)、翻譯和情感分析。
代碼:
from typing import Dict, Any, TypedDict
from langgraph.graph import StateGraph, END
from langchain_core.runnables import RunnableConfig
from textblob import TextBlob
import re
import time
class AgentState(TypedDict):
text: str
summary: str
translation: str
sentiment: str
summary_time: float
translation_time: float
sentiment_time: float
def summarize_agent(state: AgentState) -> Dict[str, Any]:
print("Summarization Agent: Running")
start_time = time.time()
try:
text = state["text"]
ifnot text.strip():
return {
"summary": "No text provided for summarization.",
"summary_time": 0.0
}
time.sleep(2)
sentences = re.split(r'(?<=[.!?]) +', text.strip())
scored_sentences = [(s, len(s.split())) for s in sentences if s]
top_sentences = [s for s, _ in sorted(scored_sentences, key=lambda x: x[1], reverse=True)[:2]]
summary = " ".join(top_sentences) if top_sentences else"Text too short to summarize."
processing_time = time.time() - start_time
print(f"Summarization Agent: Completed in {processing_time:.2f} seconds")
return {
"summary": summary,
"summary_time": processing_time
}
except Exception as e:
return {
"summary": f"Error in summarization: {str(e)}",
"summary_time": 0.0
}
def translate_agent(state: AgentState) -> Dict[str, Any]:
print("Translation Agent: Running")
start_time = time.time()
try:
text = state["text"]
ifnot text.strip():
return {
"translation": "No text provided for translation.",
"translation_time": 0.0
}
time.sleep(3)
translation = (
"El nuevo parque en la ciudad es una maravillosa adición. "
"Las familias disfrutan de los espacios abiertos, y a los ni?os les encanta el parque infantil. "
"Sin embargo, algunas personas piensan que el área de estacionamiento es demasiado peque?a."
)
processing_time = time.time() - start_time
print(f"Translation Agent: Completed in {processing_time:.2f} seconds")
return {
"translation": translation,
"translation_time": processing_time
}
except Exception as e:
return {
"translation": f"Error in translation: {str(e)}",
"translation_time": 0.0
}
def sentiment_agent(state: AgentState) -> Dict[str, Any]:
print("Sentiment Agent: Running")
start_time = time.time()
try:
text = state["text"]
ifnot text.strip():
return {
"sentiment": "No text provided for sentiment analysis.",
"sentiment_time": 0.0
}
time.sleep(1.5)
blob = TextBlob(text)
polarity = blob.sentiment.polarity
subjectivity = blob.sentiment.subjectivity
sentiment = "Positive"if polarity > 0else"Negative"if polarity < 0else"Neutral"
result = f"{sentiment} (Polarity: {polarity:.2f}, Subjectivity: {subjectivity:.2f})"
processing_time = time.time() - start_time
print(f"Sentiment Agent: Completed in {processing_time:.2f} seconds")
return {
"sentiment": result,
"sentiment_time": processing_time
}
except Exception as e:
return {
"sentiment": f"Error in sentiment analysis: {str(e)}",
"sentiment_time": 0.0
}
def join_parallel_results(state: AgentState) -> AgentState:
return state
def build_parallel_graph() -> StateGraph:
workflow = StateGraph(AgentState)
parallel_branches = {
"summarize_node": summarize_agent,
"translate_node": translate_agent,
"sentiment_node": sentiment_agent
}
for name, agent in parallel_branches.items():
workflow.add_node(name, agent)
workflow.add_node("branch", lambda state: state)
workflow.add_node("join", join_parallel_results)
workflow.set_entry_point("branch")
for name in parallel_branches:
workflow.add_edge("branch", name)
workflow.add_edge(name, "join")
workflow.add_edge("join", END)
return workflow.compile()
def main():
text = (
"The new park in the city is a wonderful addition. Families are enjoying the open spaces, "
"and children love the playground. However, some people think the parking area is too small."
)
initial_state: AgentState = {
"text": text,
"summary": "",
"translation": "",
"sentiment": "",
"summary_time": 0.0,
"translation_time": 0.0,
"sentiment_time": 0.0
}
print("\nBuilding new graph...")
app = build_parallel_graph()
print("\nStarting parallel processing...")
start_time = time.time()
config = RunnableConfig(parallel=True)
result = app.invoke(initial_state, cnotallow=config)
total_time = time.time() - start_time
print("\n=== Parallel Task Results ===")
print(f"Input Text:\n{text}\n")
print(f"Summary:\n{result['summary']}\n")
print(f"Translation (Spanish):\n{result['translation']}\n")
print(f"Sentiment Analysis:\n{result['sentiment']}\n")
print("\n=== Processing Times ===")
processing_times = {
"summary": result["summary_time"],
"translation": result["translation_time"],
"sentiment": result["sentiment_time"]
}
for agent, time_taken in processing_times.items():
print(f"{agent.capitalize()}: {time_taken:.2f} seconds")
print(f"\nTotal Wall Clock Time: {total_time:.2f} seconds")
print(f"Sum of Individual Processing Times: {sum(processing_times.values()):.2f} seconds")
print(f"Time Saved by Parallel Processing: {sum(processing_times.values()) - total_time:.2f} seconds")
if __name__ == "__main__":
main()
輸出:
Building new graph...
Starting parallel processing...
Sentiment Agent: Running
Summarization Agent: Running
Translation Agent: Running
Sentiment Agent: Completed in 1.50 seconds
Summarization Agent: Completed in 2.00 seconds
Translation Agent: Completed in 3.00 seconds
=== Parallel Task Results ===
Input Text:
The new park in the city is a wonderful addition. Families are enjoying the open spaces, and children love the playground. However, some people think the parking area is too small.
Summary:
Families are enjoying the open spaces, and children love the playground. The new park in the city is a wonderful addition.
Translation (Spanish):
El nuevo parque en la ciudad es una maravillosa adición. Las familias disfrutan de los espacios abiertos, y a los ni?os les encanta el parque infantil. Sin embargo, algunas personas piensan que el área de estacionamiento es demasiado peque?a.
Sentiment Analysis:
Positive (Polarity: 0.31, Subjectivity: 0.59)
=== Processing Times ===
Summary: 2.00 seconds
Translation: 3.00 seconds
Sentiment: 1.50 seconds
Total Wall Clock Time: 3.01 seconds
Sum of Individual Processing Times: 6.50 seconds
Time Saved by Parallel Processing: 3.50 seconds
并行模式特點:
- 并行性:三個任務(wù)(總結(jié)、翻譯、情感分析)同時運行,減少了總處理時間。
- 獨立性:每個代理獨立處理輸入文本,執(zhí)行過程中無需代理間通信。
- 協(xié)調(diào)性:隊列確保結(jié)果被安全收集并按順序顯示。
- 實際用例:總結(jié)、翻譯和情感分析是常見的自然語言處理(NLP)任務(wù),并行處理對較大文本特別有益。
2.1.2 順序
任務(wù)按順序依次處理,前一個代理的輸出成為下一個代理的輸入。
示例:多步審批流程。
代碼:
from typing import Dict
from langgraph.graph import StateGraph, MessagesState, END
from langchain_core.runnables import RunnableConfig
from langchain_core.messages import HumanMessage, AIMessage
import json
def team_lead_agent(state: MessagesState, config: RunnableConfig) -> Dict:
print("Agent (Team Lead): Starting review")
messages = state["messages"]
proposal = json.loads(messages[0].content)
title = proposal.get("title", "")
amount = proposal.get("amount", 0.0)
ifnot title or amount <= 0:
status = "Rejected"
comment = "Team Lead: Proposal rejected due to missing title or invalid amount."
goto = END
else:
status = "Approved by Team Lead"
comment = "Team Lead: Proposal is complete and approved."
goto = "dept_manager"
print(f"Agent (Team Lead): Review complete - {status}")
messages.append(AIMessage(
cnotallow=json.dumps({"status": status, "comment": comment}),
additional_kwargs={"agent": "team_lead", "goto": goto}
))
return {"messages": messages}
def dept_manager_agent(state: MessagesState, config: RunnableConfig) -> Dict:
print("Agent (Department Manager): Starting review")
messages = state["messages"]
team_lead_msg = next((m for m in messages if m.additional_kwargs.get("agent") == "team_lead"), None)
proposal = json.loads(messages[0].content)
amount = proposal.get("amount", 0.0)
if json.loads(team_lead_msg.content)["status"] != "Approved by Team Lead":
status = "Rejected"
comment = "Department Manager: Skipped due to Team Lead rejection."
goto = END
elif amount > 100000:
status = "Rejected"
comment = "Department Manager: Budget exceeds limit."
goto = END
else:
status = "Approved by Department Manager"
comment = "Department Manager: Budget is within limits."
goto = "finance_director"
print(f"Agent (Department Manager): Review complete - {status}")
messages.append(AIMessage(
cnotallow=json.dumps({"status": status, "comment": comment}),
additional_kwargs={"agent": "dept_manager", "goto": goto}
))
return {"messages": messages}
def finance_director_agent(state: MessagesState, config: RunnableConfig) -> Dict:
print("Agent (Finance Director): Starting review")
messages = state["messages"]
dept_msg = next((m for m in messages if m.additional_kwargs.get("agent") == "dept_manager"), None)
proposal = json.loads(messages[0].content)
amount = proposal.get("amount", 0.0)
if json.loads(dept_msg.content)["status"] != "Approved by Department Manager":
status = "Rejected"
comment = "Finance Director: Skipped due to Dept Manager rejection."
elif amount > 50000:
status = "Rejected"
comment = "Finance Director: Insufficient budget."
else:
status = "Approved"
comment = "Finance Director: Approved and feasible."
print(f"Agent (Finance Director): Review complete - {status}")
messages.append(AIMessage(
cnotallow=json.dumps({"status": status, "comment": comment}),
additional_kwargs={"agent": "finance_director", "goto": END}
))
return {"messages": messages}
def route_step(state: MessagesState) -> str:
for msg in reversed(state["messages"]):
goto = msg.additional_kwargs.get("goto")
if goto:
print(f"Routing: Agent {msg.additional_kwargs.get('agent')} set goto to {goto}")
return goto
return END
builder = StateGraph(MessagesState)
builder.add_node("team_lead", team_lead_agent)
builder.add_node("dept_manager", dept_manager_agent)
builder.add_node("finance_director", finance_director_agent)
builder.set_entry_point("team_lead")
builder.add_conditional_edges("team_lead", route_step, {
"dept_manager": "dept_manager",
END: END
})
builder.add_conditional_edges("dept_manager", route_step, {
"finance_director": "finance_director",
END: END
})
builder.add_conditional_edges("finance_director", route_step, {
END: END
})
workflow = builder.compile()
def main():
initial_state = {
"messages": [
HumanMessage(
cnotallow=json.dumps({
"title": "New Equipment Purchase",
"amount": 40000.0,
"department": "Engineering"
})
)
]
}
result = workflow.invoke(initial_state)
messages = result["messages"]
proposal = json.loads(messages[0].content)
print("\n=== Approval Results ===")
print(f"Proposal Title: {proposal['title']}")
final_status = "Unknown"
comments = []
for msg in messages[1:]:
if isinstance(msg, AIMessage):
try:
data = json.loads(msg.content)
if"status"in data:
final_status = data["status"]
if"comment"in data:
comments.append(data["comment"])
except Exception:
continue
print(f"Final Status: {final_status}")
print("Comments:")
for comment in comments:
print(f" - {comment}")
if __name__ == "__main__":
main()
輸出(金額 = 40,000美元):
Agent (Team Lead): Starting review
Agent (Team Lead): Review complete - Approved by Team Lead
Routing: Agent team_lead set goto to dept_manager
Agent (Department Manager): Starting review
Agent (Department Manager): Review complete - Approved by Department Manager
Routing: Agent dept_manager set goto to finance_director
Agent (Finance Director): Starting review
Agent (Finance Director): Review complete - Approved
Routing: Agent finance_director set goto to __end__
=== Approval Results ===
Proposal Title: New Equipment Purchase
Final Status: Approved
Comments:
- Team Lead: Proposal is complete and approved.
- Department Manager: Budget is within limits.
- Finance Director: Approved and feasible.
順序執(zhí)行特點:
- 順序執(zhí)行:代理按順序運行:團隊領(lǐng)導(dǎo) → 部門經(jīng)理 → 財務(wù)總監(jiān)。
- 中斷機制:如果任何一個代理拒絕,循環(huán)將中斷,跳過剩余代理。
- 對象修改:每個代理修改共享的提案對象,更新狀態(tài)和評論。
協(xié)調(diào)方式:
- 結(jié)果存儲在列表中,但提案對象在代理之間傳遞狀態(tài)。
- 不使用多進程,確保單線程、有序的工作流程。
2.1.3 循環(huán)
代理以迭代循環(huán)的方式運作,基于其他代理的反饋持續(xù)改進它們的輸出。
示例:評估用例,如代碼編寫與代碼測試。
代碼:
from typing import Dict, Any, List
from langgraph.graph import StateGraph, END
from langchain_core.runnables import RunnableConfig
import textwrap
class EvaluationState(Dict[str, Any]):
code: str = ""
feedback: str = ""
passed: bool = False
iteration: int = 0
max_iterations: int = 3
history: List[Dict] = []
def __init__(self, *args, **kwargs):
super().__init__(*args, **kwargs)
self.setdefault("code", "")
self.setdefault("feedback", "")
self.setdefault("passed", False)
self.setdefault("iteration", 0)
self.setdefault("max_iterations", 3)
self.setdefault("history", [])
def code_writer_agent(state: EvaluationState, config: RunnableConfig) -> Dict[str, Any]:
print(f"Iteration {state['iteration'] + 1} - Code Writer: Generating code")
print(f"Iteration {state['iteration'] + 1} - Code Writer: Received feedback: {state['feedback']}")
iteration = state["iteration"] + 1
feedback = state["feedback"]
if iteration == 1:
code = textwrap.dedent("""
def factorial(n):
result = 1
for i in range(1, n + 1):
result *= i
return result
""")
writer_feedback = "Initial code generated."
elif"factorial(0)"in feedback.lower():
code = textwrap.dedent("""
def factorial(n):
if n == 0:
return 1
result = 1
for i in range(1, n + 1):
result *= i
return result
""")
writer_feedback = "Fixed handling for n=0."
elif"factorial(-1)"in feedback.lower() or"negative"in feedback.lower():
code = textwrap.dedent("""
def factorial(n):
if n < 0:
raise ValueError("Factorial not defined for negative numbers")
if n == 0:
return 1
result = 1
for i in range(1, n + 1):
result *= i
return result
""")
writer_feedback = "Added error handling for negative inputs."
else:
code = state["code"]
writer_feedback = "No further improvements identified."
print(f"Iteration {iteration} - Code Writer: Code generated")
return {
"code": code,
"feedback": writer_feedback,
"iteration": iteration
}
def code_tester_agent(state: EvaluationState, config: RunnableConfig) -> Dict[str, Any]:
print(f"Iteration {state['iteration']} - Code Tester: Testing code")
code = state["code"]
try:
test_cases = [
(0, 1),
(1, 1),
(5, 120),
(-1, None),
]
namespace = {}
exec(code, namespace)
factorial = namespace.get('factorial')
ifnot callable(factorial):
return {"passed": False, "feedback": "No factorial function found."}
feedback_parts = []
passed = True
for input_val, expected in test_cases:
try:
result = factorial(input_val)
if expected isNone:
passed = False
feedback_parts.append(f"Test failed: factorial({input_val}) should raise an error.")
elif result != expected:
passed = False
feedback_parts.append(f"Test failed: factorial({input_val}) returned {result}, expected {expected}.")
except ValueError as ve:
if expected isnotNone:
passed = False
feedback_parts.append(f"Test failed: factorial({input_val}) raised ValueError unexpectedly: {str(ve)}")
except Exception as e:
passed = False
feedback_parts.append(f"Test failed: factorial({input_val}) caused error: {str(e)}")
feedback = "All tests passed!"if passed else"\n".join(feedback_parts)
print(f"Iteration {state['iteration']} - Code Tester: Testing complete - {'Passed' if passed else 'Failed'}")
history = state["history"]
history.append({
"iteration": state["iteration"],
"code": code,
"feedback": feedback,
"passed": passed
})
return {
"passed": passed,
"feedback": feedback,
"history": history
}
except Exception as e:
print(f"Iteration {state['iteration']} - Code Tester: Failed")
return {"passed": False, "feedback": f"Error in testing: {str(e)}"}
def should_continue(state: EvaluationState) -> str:
if state["passed"] or state["iteration"] >= state["max_iterations"]:
print(f"Iteration {state['iteration']} - {'Loop stops: Tests passed' if state['passed'] else 'Loop stops: Max iterations reached'}")
return"end"
print(f"Iteration {state['iteration']} - Loop continues: Tests failed")
return"code_writer"
workflow = StateGraph(EvaluationState)
workflow.add_node("code_writer", code_writer_agent)
workflow.add_node("code_tester", code_tester_agent)
workflow.set_entry_point("code_writer")
workflow.add_edge("code_writer", "code_tester")
workflow.add_conditional_edges(
"code_tester",
should_continue,
"code_writer": "code_writer",
"end": END
)
app = workflow.compile()
def main():
initial_state = EvaluationState()
result = app.invoke(initial_state)
print("\n=== Evaluation Results ===")
print(f"Final Status: {'Passed' if result['passed'] else 'Failed'} after {result['iteration']} iteration(s)")
print(f"Final Code:\n{result['code']}")
print(f"Final Feedback:\n{result['feedback']}")
print("\nIteration History:")
for attempt in result["history"]:
print(f"Iteration {attempt['iteration']}:")
print(f" Code:\n{attempt['code']}")
print(f" Feedback: {attempt['feedback']}")
print(f" Passed: {attempt['passed']}\n")
if __name__ == "__main__":
main()
輸出:
Iteration 1 - Code Writer: Generating code
Iteration 1 - Code Writer: Received feedback:
Iteration 1 - Code Writer: Code generated
Iteration 1 - Code Tester: Testing code
Iteration 1 - Code Tester: Testing complete - Failed
Iteration 1 - Loop continues: Tests failed
Iteration 2 - Code Writer: Generating code
Iteration 2 - Code Writer: Received feedback: Test failed: factorial(-1) should raise an error.
Iteration 2 - Code Writer: Code generated
Iteration 2 - Code Tester: Testing code
Iteration 2 - Code Tester: Testing complete - Passed
Iteration 2 - Loop stops: Tests passed
=== Evaluation Results ===
Final Status: Passed after 2 iteration(s)
Final Code:
def factorial(n):
if n < 0:
raise ValueError("Factorial not defined for negative numbers")
if n == 0:
return 1
result = 1
for i in range(1, n + 1):
result *= i
return result
Final Feedback:
All tests passed!
Iteration History:
Iteration 1:
Code:
def factorial(n):
result = 1
for i in range(1, n + 1):
result *= i
return result
Feedback: Test failed: factorial(-1) should raise an error.
Passed: False
Iteration 2:
Code:
def factorial(n):
if n < 0:
raise ValueError("Factorial not defined for negative numbers")
if n == 0:
return 1
result = 1
for i in range(1, n + 1):
result *= i
return result
Feedback: All tests passed!
Passed: True
綜合反饋:代碼測試器現(xiàn)在會報告所有測試失敗的情況,確保代碼編寫器擁有逐步修復(fù)問題所需的信息。
正確的反饋處理:代碼編寫器優(yōu)先處理修復(fù)(先處理零的情況,然后是負輸入的情況),確保逐步改進。
循環(huán)終止:當測試通過時,循環(huán)會正確退出,而不會不必要地運行全部3次迭代。
2.1.4 路由器
一個中央路由器根據(jù)任務(wù)或輸入來決定調(diào)用哪些代理。
示例:客戶支持工單路由。
代碼:
from typing import Dict, Any, TypedDict, Literal
from langgraph.graph import StateGraph, END
from langchain_core.runnables import RunnableConfig
import re
import time
class TicketState(TypedDict):
ticket_text: str
category: str
resolution: str
processing_time: float
def router_agent(state: TicketState) -> Dict[str, Any]:
print("Router Agent: Analyzing ticket...")
start_time = time.time()
ticket_text = state["ticket_text"].lower()
if any(keyword in ticket_text for keyword in ["billing", "payment", "invoice", "charge"]):
category = "Billing"
elif any(keyword in ticket_text for keyword in ["technical", "bug", "error", "crash"]):
category = "Technical"
elif any(keyword in ticket_text for keyword in ["general", "question", "inquiry", "info"]):
category = "General"
else:
category = "Unknown"
processing_time = time.time() - start_time
print(f"Router Agent: Categorized as '{category}' in {processing_time:.2f} seconds")
return {
"category": category,
"processing_time": processing_time
}
def billing_team_agent(state: TicketState) -> Dict[str, Any]:
print("Billing Team Agent: Processing ticket...")
start_time = time.time()
ticket_text = state["ticket_text"]
resolution = f"Billing Team: Reviewed ticket '{ticket_text}'. Please check your invoice details or contact our billing department for further assistance."
processing_time = time.time() - start_time
time.sleep(1)
print(f"Billing Team Agent: Completed in {processing_time:.2f} seconds")
return {
"resolution": resolution,
"processing_time": state["processing_time"] + processing_time
}
def technical_team_agent(state: TicketState) -> Dict[str, Any]:
print("Technical Team Agent: Processing ticket...")
start_time = time.time()
ticket_text = state["ticket_text"]
resolution = f"Technical Team: Reviewed ticket '{ticket_text}'. Please try restarting your device or submit a detailed error log for further investigation."
processing_time = time.time() - start_time
time.sleep(1.5)
print(f"Technical Team Agent: Completed in {processing_time:.2f} seconds")
return {
"resolution": resolution,
"processing_time": state["processing_time"] + processing_time
}
def general_team_agent(state: TicketState) -> Dict[str, Any]:
print("General Team Agent: Processing ticket...")
start_time = time.time()
ticket_text = state["ticket_text"]
resolution = f"General Team: Reviewed ticket '{ticket_text}'. For more information, please refer to our FAQ or contact us via email."
processing_time = time.time() - start_time
time.sleep(0.8)
print(f"General Team Agent: Completed in {processing_time:.2f} seconds")
return {
"resolution": resolution,
"processing_time": state["processing_time"] + processing_time
}
def manual_review_agent(state: TicketState) -> Dict[str, Any]:
print("Manual Review Agent: Processing ticket...")
start_time = time.time()
ticket_text = state["ticket_text"]
resolution = f"Manual Review: Ticket '{ticket_text}' could not be categorized. Flagged for human review. Please assign to the appropriate team manually."
processing_time = time.time() - start_time
time.sleep(0.5)
print(f"Manual Review Agent: Completed in {processing_time:.2f} seconds")
return {
"resolution": resolution,
"processing_time": state["processing_time"] + processing_time
}
def route_ticket(state: TicketState) -> Literal["billing_team", "technical_team", "general_team", "manual_review"]:
category = state["category"]
print(f"Routing: Ticket category is '{category}'")
if category == "Billing":
return"billing_team"
elif category == "Technical":
return"technical_team"
elif category == "General":
return"general_team"
else:
return"manual_review"
def build_router_graph() -> StateGraph:
workflow = StateGraph(TicketState)
workflow.add_node("router", router_agent)
workflow.add_node("billing_team", billing_team_agent)
workflow.add_node("technical_team", technical_team_agent)
workflow.add_node("general_team", general_team_agent)
workflow.add_node("manual_review", manual_review_agent)
workflow.set_entry_point("router")
workflow.add_conditional_edges(
"router",
route_ticket,
"billing_team": "billing_team",
"technical_team": "technical_team",
"general_team": "general_team",
"manual_review": "manual_review"
)
workflow.add_edge("billing_team", END)
workflow.add_edge("technical_team", END)
workflow.add_edge("general_team", END)
workflow.add_edge("manual_review", END)
return workflow.compile()
def main():
test_tickets = [
"I have a billing issue with my last invoice. It seems I was overcharged.",
"My app keeps crashing with a technical error. Please help!",
"I have a general question about your services. Can you provide more info?",
"I need assistance with something unrelated to billing or technical issues."
]
for ticket_text in test_tickets:
initial_state: TicketState = {
"ticket_text": ticket_text,
"category": "",
"resolution": "",
"processing_time": 0.0
}
print(f"\n=== Processing Ticket: '{ticket_text}' ===")
app = build_router_graph()
start_time = time.time()
result = app.invoke(initial_state, cnotallow=RunnableConfig())
total_time = time.time() - start_time
print("\n=== Ticket Results ===")
print(f"Category: {result['category']}")
print(f"Resolution: {result['resolution']}")
print(f"Total Processing Time: {result['processing_time']:.2f} seconds")
print(f"Total Wall Clock Time: {total_time:.2f} seconds")
print("-" * 50)
if __name__ == "__main__":
main()
輸出:
=== Processing Ticket: 'I have a billing issue with my last invoice. It seems I was overcharged.' ===
Router Agent: Analyzing ticket...
Router Agent: Categorized as 'Billing'in 0.00 seconds
Routing: Ticket category is 'Billing'
Billing Team Agent: Processing ticket...
Billing Team Agent: Completed in 0.00 seconds
=== Ticket Results ===
Category: Billing
Resolution: Billing Team: Reviewed ticket 'I have a billing issue with my last invoice. It seems I was overcharged.'. Please check your invoice details or contact our billing department for further assistance.
Total Processing Time: 0.00 seconds
Total Wall Clock Time: 1.03 seconds
--------------------------------------------------
=== Processing Ticket: 'My app keeps crashing with a technical error. Please help!' ===
Router Agent: Analyzing ticket...
Router Agent: Categorized as 'Technical'in 0.00 seconds
Routing: Ticket category is 'Technical'
Technical Team Agent: Processing ticket...
Technical Team Agent: Completed in 0.00 seconds
=== Ticket Results ===
Category: Technical
Resolution: Technical Team: Reviewed ticket 'My app keeps crashing with a technical error. Please help!'. Please try restarting your device or submit a detailed error logfor further investigation.
Total Processing Time: 0.00 seconds
Total Wall Clock Time: 1.50 seconds
--------------------------------------------------
=== Processing Ticket: 'I have a general question about your services. Can you provide more info?' ===
Router Agent: Analyzing ticket...
Router Agent: Categorized as 'General'in 0.00 seconds
Routing: Ticket category is 'General'
General Team Agent: Processing ticket...
General Team Agent: Completed in 0.00 seconds
=== Ticket Results ===
Category: General
Resolution: General Team: Reviewed ticket 'I have a general question about your services. Can you provide more info?'. For more information, please refer to our FAQ or contact us via email.
Total Processing Time: 0.00 seconds
Total Wall Clock Time: 0.80 seconds
--------------------------------------------------
=== Processing Ticket: 'I need assistance with something unrelated to billing or technical issues.' ===
Router Agent: Analyzing ticket...
Router Agent: Categorized as 'Billing'in 0.00 seconds
Routing: Ticket category is 'Billing'
Billing Team Agent: Processing ticket...
Billing Team Agent: Completed in 0.00 seconds
=== Ticket Results ===
Category: Billing
Resolution: Billing Team: Reviewed ticket 'I need assistance with something unrelated to billing or technical issues.'. Please check your invoice details or contact our billing department for further assistance.
Total Processing Time: 0.00 seconds
Total Wall Clock Time: 1.00 seconds
--------------------------------------------------
動態(tài)路由:??router_agent?
?? 確定工單類別,??route_ticket?
?? 函數(shù)使用 ??add_conditional_edges?
? 將工作流程導(dǎo)向相應(yīng)的節(jié)點。
基于條件的流程:與并行模式(多個節(jié)點同時運行)不同,路由器模式根據(jù)條件(類別)僅執(zhí)行一條路徑。
可擴展性:您可以通過擴展節(jié)點并更新 ??route_ticket?
? 函數(shù)以處理新類別,來添加更多的支持團隊。
2.1.5 聚合器(或合成器)
多個智能體各自貢獻輸出,由一個聚合智能體收集這些輸出,并將其合成為最終結(jié)果。
示例:社交媒體情感分析聚合器
代碼:
from typing import Dict, Any, TypedDict, List
from langgraph.graph import StateGraph, END
from langchain_core.runnables import RunnableConfig
from textblob import TextBlob
import time
from typing_extensions import Annotated
from operator import add
# Step 1: Define the State
class SocialMediaState(TypedDict):
twitter_posts: List[str]
instagram_posts: List[str]
reddit_posts: List[str]
twitter_sentiment: Dict[str, float]
instagram_sentiment: Dict[str, float]
reddit_sentiment: Dict[str, float]
final_report: str
processing_time: Annotated[float, add]
# Step 2: Define the Post Collection Agents
def collect_twitter_posts(state: SocialMediaState) -> Dict[str, Any]:
print("Twitter Agent: Collecting posts...")
start_time = time.time()
posts = [
"Loving the new product from this brand! Amazing quality.",
"Terrible customer service from this brand. Very disappointed."
]
time.sleep(1) # Simulate processing time
processing_time = time.time() - start_time # Include time.sleep in processing_time
print(f"Twitter Agent: Completed in {processing_time:.2f} seconds")
return {
"twitter_posts": posts,
"processing_time": processing_time
}
def collect_instagram_posts(state: SocialMediaState) -> Dict[str, Any]:
print("Instagram Agent: Collecting posts...")
start_time = time.time()
posts = [
"Beautiful design by this brand! #loveit",
"Not impressed with the latest release. Expected better."
]
time.sleep(1.2) # Simulate processing time
processing_time = time.time() - start_time
print(f"Instagram Agent: Completed in {processing_time:.2f} seconds")
return {
"instagram_posts": posts,
"processing_time": processing_time
}
def collect_reddit_posts(state: SocialMediaState) -> Dict[str, Any]:
print("Reddit Agent: Collecting posts...")
start_time = time.time()
posts = [
"This brand is awesome! Great value for money.",
"Had a bad experience with their support team. Not happy."
]
time.sleep(0.8) # Simulate processing time
processing_time = time.time() - start_time
print(f"Reddit Agent: Completed in {processing_time:.2f} seconds")
return {
"reddit_posts": posts,
"processing_time": processing_time
}
# Step 3: Define the Sentiment Analysis Agents
def analyze_twitter_sentiment(state: SocialMediaState) -> Dict[str, Any]:
print("Twitter Sentiment Agent: Analyzing sentiment...")
start_time = time.time()
posts = state["twitter_posts"]
polarities = [TextBlob(post).sentiment.polarity for post in posts]
avg_polarity = sum(polarities) / len(polarities) if polarities else0.0
time.sleep(0.5) # Simulate processing time
processing_time = time.time() - start_time
print(f"Twitter Sentiment Agent: Completed in {processing_time:.2f} seconds")
return {
"twitter_sentiment": {"average_polarity": avg_polarity, "num_posts": len(posts)},
"processing_time": processing_time
}
def analyze_instagram_sentiment(state: SocialMediaState) -> Dict[str, Any]:
print("Instagram Sentiment Agent: Analyzing sentiment...")
start_time = time.time()
posts = state["instagram_posts"]
polarities = [TextBlob(post).sentiment.polarity for post in posts]
avg_polarity = sum(polarities) / len(polarities) if polarities else0.0
time.sleep(0.6) # Simulate processing time
processing_time = time.time() - start_time
print(f"Instagram Sentiment Agent: Completed in {processing_time:.2f} seconds")
return {
"instagram_sentiment": {"average_polarity": avg_polarity, "num_posts": len(posts)},
"processing_time": processing_time
}
def analyze_reddit_sentiment(state: SocialMediaState) -> Dict[str, Any]:
print("Reddit Sentiment Agent: Analyzing sentiment...")
start_time = time.time()
posts = state["reddit_posts"]
polarities = [TextBlob(post).sentiment.polarity for post in posts]
avg_polarity = sum(polarities) / len(polarities) if polarities else0.0
time.sleep(0.4) # Simulate processing time
processing_time = time.time() - start_time
print(f"Reddit Sentiment Agent: Completed in {processing_time:.2f} seconds")
return {
"reddit_sentiment": {"average_polarity": avg_polarity, "num_posts": len(posts)},
"processing_time": processing_time
}
# Step 4: Define the Aggregator Agent
def aggregate_results(state: SocialMediaState) -> Dict[str, Any]:
print("Aggregator Agent: Generating final report...")
start_time = time.time()
twitter_sentiment = state["twitter_sentiment"]
instagram_sentiment = state["instagram_sentiment"]
reddit_sentiment = state["reddit_sentiment"]
total_posts = (twitter_sentiment["num_posts"] +
instagram_sentiment["num_posts"] +
reddit_sentiment["num_posts"])
weighted_polarity = (
twitter_sentiment["average_polarity"] * twitter_sentiment["num_posts"] +
instagram_sentiment["average_polarity"] * instagram_sentiment["num_posts"] +
reddit_sentiment["average_polarity"] * reddit_sentiment["num_posts"]
) / total_posts if total_posts > 0else0.0
overall_sentiment = ("Positive"if weighted_polarity > 0else
"Negative"if weighted_polarity < 0else"Neutral")
report = (
f"Overall Sentiment: {overall_sentiment} (Average Polarity: {weighted_polarity:.2f})\n"
f"Twitter Sentiment: {twitter_sentiment['average_polarity']:.2f} (Posts: {twitter_sentiment['num_posts']})\n"
f"Instagram Sentiment: {instagram_sentiment['average_polarity']:.2f} (Posts: {instagram_sentiment['num_posts']})\n"
f"Reddit Sentiment: {reddit_sentiment['average_polarity']:.2f} (Posts: {reddit_sentiment['num_posts']})"
)
time.sleep(0.3) # Simulate processing time
processing_time = time.time() - start_time
print(f"Aggregator Agent: Completed in {processing_time:.2f} seconds")
return {
"final_report": report,
"processing_time": processing_time
}
# Step 5: Build the Graph with an Aggregator Pattern
def build_aggregator_graph() -> StateGraph:
workflow = StateGraph(SocialMediaState)
# Add nodes for collecting posts
workflow.add_node("collect_twitter", collect_twitter_posts)
workflow.add_node("collect_instagram", collect_instagram_posts)
workflow.add_node("collect_reddit", collect_reddit_posts)
# Add nodes for sentiment analysis
workflow.add_node("analyze_twitter", analyze_twitter_sentiment)
workflow.add_node("analyze_instagram", analyze_instagram_sentiment)
workflow.add_node("analyze_reddit", analyze_reddit_sentiment)
# Add node for aggregation
workflow.add_node("aggregate", aggregate_results)
# Add a branching node to trigger all collection nodes in parallel
workflow.add_node("branch", lambda state: state)
# Set the entry point to the branch node
workflow.set_entry_point("branch")
# Add edges from branch to collection nodes (parallel execution)
workflow.add_edge("branch", "collect_twitter")
workflow.add_edge("branch", "collect_instagram")
workflow.add_edge("branch", "collect_reddit")
# Add edges from collection to sentiment analysis
workflow.add_edge("collect_twitter", "analyze_twitter")
workflow.add_edge("collect_instagram", "analyze_instagram")
workflow.add_edge("collect_reddit", "analyze_reddit")
# Add edges from sentiment analysis to aggregator
workflow.add_edge("analyze_twitter", "aggregate")
workflow.add_edge("analyze_instagram", "aggregate")
workflow.add_edge("analyze_reddit", "aggregate")
# Add edge from aggregator to END
workflow.add_edge("aggregate", END)
return workflow.compile()
# Step 6: Run the Workflow
def main():
initial_state: SocialMediaState = {
"twitter_posts": [],
"instagram_posts": [],
"reddit_posts": [],
"twitter_sentiment": {"average_polarity": 0.0, "num_posts": 0},
"instagram_sentiment": {"average_polarity": 0.0, "num_posts": 0},
"reddit_sentiment": {"average_polarity": 0.0, "num_posts": 0},
"final_report": "",
"processing_time": 0.0
}
print("\nStarting social media sentiment analysis...")
app = build_aggregator_graph()
start_time = time.time()
config = RunnableConfig(parallel=True)
result = app.invoke(initial_state, cnotallow=config)
total_time = time.time() - start_time
print("\n=== Sentiment Analysis Results ===")
print(result["final_report"])
print(f"\nTotal Processing Time: {result['processing_time']:.2f} seconds")
print(f"Total Wall Clock Time: {total_time:.2f} seconds")
if __name__ == "__main__":
main()
輸出:
Starting social media sentiment analysis...
Instagram Agent: Collecting posts...
Reddit Agent: Collecting posts...
Twitter Agent: Collecting posts...
Reddit Agent: Completed in 0.80 seconds
Twitter Agent: Completed in 1.00 seconds
Instagram Agent: Completed in 1.20 seconds
Instagram Sentiment Agent: Analyzing sentiment...
Reddit Sentiment Agent: Analyzing sentiment...
Twitter Sentiment Agent: Analyzing sentiment...
Reddit Sentiment Agent: Completed in 0.40 seconds
Twitter Sentiment Agent: Completed in 0.50 seconds
Instagram Sentiment Agent: Completed in 0.60 seconds
Aggregator Agent: Generating final report...
Aggregator Agent: Completed in 0.30 seconds
=== Sentiment Analysis Results ===
Overall Sentiment: Positive (Average Polarity: 0.15)
Twitter Sentiment: -0.27 (Posts: 2)
Instagram Sentiment: 0.55 (Posts: 2)
Reddit Sentiment: 0.18 (Posts: 2)
Total Processing Time: 4.80 seconds
Total Wall Clock Time: 2.13 seconds
并行執(zhí)行
收集和分析節(jié)點并行運行,與各個處理時間的總和(3.8秒)相比,總耗時(2.1秒)有所減少。
聚合
聚合節(jié)點將情感分析結(jié)果整合到最終報告中,計算整體情感傾向,并按平臺進行細分。
2.1.6 網(wǎng)絡(luò)(或橫向)架構(gòu)
智能體之間以多對多的方式直接通信,形成一個去中心化的網(wǎng)絡(luò)。
這種架構(gòu)適用于不存在明確智能體層級關(guān)系,或?qū)χ悄荏w調(diào)用順序沒有特定要求的問題場景。
from typing import Literal
from langchain_openai import ChatOpenAI
from langgraph.graph import StateGraph, MessagesState, START, END
model = ChatOpenAI()
def agent_1(state: MessagesState) -> Command[Literal["agent_2", "agent_3", END]]:
response = model.invoke(...)
return Command(
goto=response["next_agent"],
update={"messages": [response["content"]]}
)
def agent_2(state: MessagesState) -> Command[Literal["agent_1", "agent_3", END]]:
response = model.invoke(...)
return Command(
goto=response["next_agent"],
update={"messages": [response["content"]]}
)
def agent_3(state: MessagesState) -> Command[Literal["agent_1", "agent_2", END]]:
...
return Command(
goto=response["next_agent"],
update={"messages": [response["content"]]}
)
builder = StateGraph(MessagesState)
builder.add_node(agent_1)
builder.add_node(agent_2)
builder.add_node(agent_3)
builder.add_edge(START, "agent_1")
network = builder.compile()
優(yōu)點:支持分布式協(xié)作和群體驅(qū)動的決策模式。即使部分智能體出現(xiàn)故障,系統(tǒng)仍能保持運行。
缺點:管理智能體之間的通信頗具挑戰(zhàn)。頻繁的通信可能導(dǎo)致效率降低,還可能出現(xiàn)智能體重復(fù)工作的情況。
2.1.7 交接
在多智能體架構(gòu)中,智能體可被視作圖的節(jié)點。每個智能體節(jié)點執(zhí)行自身步驟,并決定是結(jié)束執(zhí)行流程,還是路由到其他智能體,甚至有可能路由回自身(比如在循環(huán)中運行)。在多智能體交互里,一種常見的模式是交接,即一個智能體將控制權(quán)轉(zhuǎn)交給另一個智能體。交接過程中,你能夠指定:
- 目的地:要導(dǎo)航至的目標智能體(比如,要前往的節(jié)點名稱)
- 有效負載:傳遞給該智能體的信息(例如,狀態(tài)更新)
在LangGraph中實現(xiàn)交接功能時,智能體節(jié)點可以返回??Command?
?對象,通過它能同時實現(xiàn)控制流和狀態(tài)更新:
def agent(state) -> Command[Literal["agent", "another_agent"]]:
goto = get_next_agent(...)
return Command(
goto=goto,
update={"my_state_key": "my_state_value"}
)
在更為復(fù)雜的場景中,每個智能體節(jié)點自身就是一個圖(即子圖),某個智能體子圖中的節(jié)點可能需要導(dǎo)航到不同的智能體。例如,你有兩個智能體alice和bob(屬于父圖中的子圖節(jié)點),如果alice需要導(dǎo)航到bob,那么可以在??Command?
??對象中設(shè)置??graph=Command.PARENT?
?:
def some_node_inside_alice(state):
return Command(
goto="bob",
update={"my_state_key": "my_state_value"},
graph=Command.PARENT
)
注意如果你需要支持使用??Command(graph=Command.PARENT)?
??進行通信的子圖的可視化,就需要用帶有??Command?
?注釋的節(jié)點函數(shù)來包裝它們。例如,不要直接這樣寫:
builder.add_node(alice)
而是需要這樣做:
def call_alice(state) -> Command[Literal["bob"]]:
return alice.invoke(state)
builder.add_node("alice", call_alice)
交接作為工具
最常見的智能體類型之一是ReAct風(fēng)格的工具調(diào)用智能體。對于這類智能體而言,一種常見的模式是將交接操作包裝在工具調(diào)用中,例如:
def transfer_to_bob(state):
"""Transfer to bob."""
return Command(
goto="bob",
update={"my_state_key": "my_state_value"},
graph=Command.PARENT
)
這是通過工具更新圖狀態(tài)的一種特殊情況,除了進行狀態(tài)更新外,還涵蓋了控制流的變更。
重要提示
如果你想使用返回??Command?
??的工具,可以選擇使用預(yù)構(gòu)建的??create_react_agent?
??/??ToolNode?
??組件,或者自行實現(xiàn)一個工具執(zhí)行節(jié)點,該節(jié)點負責(zé)收集工具返回的??Command?
?對象,并返回這些對象的列表。例如:
def call_tools(state):
...
commands = [tools_by_name[tool_call["name"]].invoke(tool_call) for tool_call in tool_calls]
return commands
現(xiàn)在,讓我們進一步深入了解不同的多智能體架構(gòu)。
2.1.8 監(jiān)督者模式
在這種架構(gòu)中,我們將各個智能體定義為節(jié)點,并添加一個監(jiān)督者節(jié)點(由大語言模型構(gòu)成),它負責(zé)決定接下來應(yīng)該調(diào)用哪些智能體節(jié)點。我們依據(jù)監(jiān)督者的決策,使用??Command?
?將執(zhí)行過程路由到合適的智能體節(jié)點。這種架構(gòu)也很適合并行運行多個智能體,或者采用映射 - 歸約(map-reduce)模式。
from typing import Literal
from langchain_openai import ChatOpenAI
from langgraph.graph import StateGraph, MessagesState, START, END
model = ChatOpenAI()
def supervisor(state: MessagesState) -> Command[Literal["agent_1", "agent_2", END]]:
response = model.invoke(...)
return Command(goto=response["next_agent"])
def agent_1(state: MessagesState) -> Command[Literal["supervisor"]]:
response = model.invoke(...)
return Command(
goto="supervisor",
update={"messages": [response]}
)
def agent_2(state: MessagesState) -> Command[Literal["supervisor"]]:
response = model.invoke(...)
return Command(
goto="supervisor",
update={"messages": [response]}
)
builder = StateGraph(MessagesState)
builder.add_node(supervisor)
builder.add_node(agent_1)
builder.add_node(agent_2)
builder.add_edge(START, "supervisor")
supervisor = builder.compile()
2.1.9 監(jiān)督者(工具調(diào)用)模式
在這種監(jiān)督者架構(gòu)的變體中,我們將單個智能體定義為工具,并在監(jiān)督者節(jié)點中使用一個調(diào)用工具的大語言模型。這可以被實現(xiàn)為一個具有兩個節(jié)點的ReAct風(fēng)格的智能體——一個大語言模型節(jié)點(監(jiān)督者)和一個執(zhí)行工具(在這種情況下即智能體)的工具調(diào)用節(jié)點。
from typing import Annotated
from langchain_openai import ChatOpenAI
from langgraph.prebuilt import InjectedState, create_react_agent
model = ChatOpenAI()
def agent_1(state: Annotated[dict, InjectedState]):
response = model.invoke(...)
return response.content
def agent_2(state: Annotated[dict, InjectedState]):
response = model.invoke(...)
return response.content
tools = [agent_1, agent_2]
supervisor = create_react_agent(model, tools)
2.1.10 分層(或垂直)架構(gòu)
智能體按照樹狀結(jié)構(gòu)進行組織,較高級別的智能體(監(jiān)督智能體)負責(zé)管理較低級別的智能體。
隨著你向系統(tǒng)中添加越來越多的智能體,監(jiān)督智能體管理所有這些智能體可能會變得極為困難。監(jiān)督智能體可能會在決定下一步調(diào)用哪個智能體時做出欠佳決策,而且對于單個監(jiān)督智能體來說,上下文可能會變得過于復(fù)雜而難以追蹤。換句話說,最終你會面臨最初促使采用多智能體架構(gòu)的那些相同問題。
為解決這一問題,你可以對系統(tǒng)進行分層設(shè)計。例如,你可以創(chuàng)建由不同監(jiān)督智能體管理的獨立、專門的智能體團隊,再設(shè)置一個頂級監(jiān)督智能體來管理這些團隊。
from typing import Literal
from langchain_openai import ChatOpenAI
from langgraph.graph import StateGraph, MessagesState, START, END
from langgraph.types import Command
model = ChatOpenAI()
def team_1_supervisor(state: MessagesState) -> Command[Literal["team_1_agent_1", "team_1_agent_2", END]]:
response = model.invoke(...)
return Command(goto=response["next_agent"])
def team_1_agent_1(state: MessagesState) -> Command[Literal["team_1_supervisor"]]:
response = model.invoke(...)
return Command(goto="team_1_supervisor", update={"messages": [response]})
def team_1_agent_2(state: MessagesState) -> Command[Literal["team_1_supervisor"]]:
response = model.invoke(...)
return Command(goto="team_1_supervisor", update={"messages": [response]})
team_1_builder = StateGraph(Team1State)
team_1_builder.add_node(team_1_supervisor)
team_1_builder.add_node(team_1_agent_1)
team_1_builder.add_node(team_1_agent_2)
team_1_builder.add_edge(START, "team_1_supervisor")
team_1_graph = team_1_builder.compile()
class Team2State(MessagesState):
next: Literal["team_2_agent_1", "team_2_agent_2", "__end__"]
def team_2_supervisor(state: Team2State):
...
def team_2_agent_1(state: Team2State):
...
def team_2_agent_2(state: Team2State):
...
team_2_builder = StateGraph(Team2State)
...
team_2_graph = team_2_builder.compile()
builder = StateGraph(MessagesState)
def top_level_supervisor(state: MessagesState) -> Command[Literal["team_1_graph", "team_2_graph", END]]:
response = model.invoke(...)
return Command(goto=response["next_team"])
builder = StateGraph(MessagesState)
builder.add_node(top_level_supervisor)
builder.add_node("team_1_graph", team_1_graph)
builder.add_node("team_2_graph", team_2_graph)
builder.add_edge(START, "top_level_supervisor")
builder.add_edge("team_1_graph", "top_level_supervisor")
builder.add_edge("team_2_graph", "top_level_supervisor")
graph = builder.compile()
優(yōu)點:不同層級的智能體之間,角色和職責(zé)劃分明確。通信流程得到簡化,適合具有結(jié)構(gòu)化決策流的大型系統(tǒng)。
缺點:上層智能體出現(xiàn)故障可能會擾亂整個系統(tǒng)的運行。下層智能體的獨立性較為有限。
2.1.11 自定義多智能體工作流程
每個智能體僅與部分智能體進行通信。工作流的部分環(huán)節(jié)是確定的,只有部分智能體能夠決定接下來調(diào)用哪些其他智能體。
在這種架構(gòu)中,我們把單個智能體作為圖節(jié)點添加進來,并在自定義工作流中提前定義好智能體的調(diào)用順序。在LangGraph中,工作流有兩種定義方式:
- 顯式控制流(普通邊):LangGraph支持你通過普通圖邊,清晰明確地定義應(yīng)用程序的控制流(即智能體之間的通信順序)。這是上述架構(gòu)中最具確定性的一種變體——我們總能提前知曉下一個被調(diào)用的智能體是哪個。
- 動態(tài)控制流(Command):在LangGraph里,你可以讓大語言模型(LLM)參與決定應(yīng)用程序控制流的部分環(huán)節(jié)。這可以通過使用?
?Command?
?來實現(xiàn)。主管工具調(diào)用架構(gòu)就是一個典型例子,在這種情況下,為主管智能體提供支持的工具調(diào)用LLM會對工具(即智能體)的調(diào)用順序做出決策。
from langchain_openai import ChatOpenAI
from langgraph.graph import StateGraph, MessagesState, START
model = ChatOpenAI()
def agent_1(state: MessagesState):
response = model.invoke(...)
return {"messages": [response]}
def agent_2(state: MessagesState):
response = model.invoke(...)
return {"messages": [response]}
builder = StateGraph(MessagesState)
builder.add_node(agent_1)
builder.add_node(agent_2)
builder.add_edge(START, "agent_1")
builder.add_edge("agent_1", "agent_2")
3. 智能體之間的通信
構(gòu)建多智能體系統(tǒng)時,最重要的是確定智能體之間的通信方式。這涉及到幾個不同的考量因素:
- 智能體是通過圖狀態(tài)進行通信,還是通過工具調(diào)用進行通信?
- 如果兩個智能體的狀態(tài)模式不同,該如何處理?
- 如何通過共享消息列表進行通信?
3.1 圖狀態(tài)與工具調(diào)用
在智能體之間傳遞的 “有效載荷” 是什么呢?在上述討論的大多數(shù)架構(gòu)中,智能體是通過圖狀態(tài)進行通信的。而在主管工具調(diào)用架構(gòu)中,有效載荷則是工具調(diào)用的參數(shù)。
- 圖狀態(tài):要通過圖狀態(tài)進行通信,需要將單個智能體定義為圖節(jié)點。這些節(jié)點既可以作為函數(shù)添加,也可以作為完整的子圖添加。在圖執(zhí)行的每一步,智能體節(jié)點接收圖的當前狀態(tài),執(zhí)行智能體代碼,然后將更新后的狀態(tài)傳遞給下一個節(jié)點。通常情況下,智能體節(jié)點共享單一的狀態(tài)模式。不過,你可能也希望設(shè)計具有不同狀態(tài)模式的智能體節(jié)點。
3.2 不同的狀態(tài)模式
某個智能體可能需要與其他智能體擁有不同的狀態(tài)模式。比如,搜索智能體可能只需要跟蹤查詢內(nèi)容和檢索到的文檔。在LangGraph中,有兩種方式可以實現(xiàn)這一點:
- 定義具有獨立狀態(tài)模式的子圖智能體。如果子圖和父圖之間沒有共享的狀態(tài)鍵(通道),添加輸入/輸出轉(zhuǎn)換至關(guān)重要,這樣父圖才能知道如何與子圖進行通信。
- 定義具有私有輸入狀態(tài)模式的智能體節(jié)點函數(shù),該模式與整體圖狀態(tài)模式不同。這使得僅在執(zhí)行特定智能體時所需的信息得以傳遞。
3.3 共享消息列表
智能體之間最常見的通信方式是通過共享狀態(tài)通道,通常是一個消息列表。這基于一個前提,即狀態(tài)中始終至少存在一個被所有智能體共享的通道(鍵)。當通過共享消息列表進行通信時,還有一個額外的考量因素:智能體應(yīng)該共享其完整的思考過程,還是僅共享最終結(jié)果呢?
共享完整思考過程
智能體可以與其他所有智能體共享其完整的思考過程(即 “便簽本”),這個 “便簽本” 通常呈現(xiàn)為一個消息列表的形式。共享完整思考過程的好處在于,它有助于其他智能體做出更優(yōu)決策,進而提升整個系統(tǒng)的推理能力。然而,其弊端是,隨著智能體數(shù)量的增多以及復(fù)雜性的增加,“便簽本” 的規(guī)模會迅速膨脹,這可能需要額外的內(nèi)存管理策略來應(yīng)對。
共享最終結(jié)果
智能體可以擁有自己的私有 “便簽本”,僅將最終結(jié)果與其他智能體共享。這種方式對于包含大量智能體或智能體較為復(fù)雜的系統(tǒng)可能更為適用。在這種情況下,你需要定義具有不同狀態(tài)模式的智能體。
對于作為工具被調(diào)用的智能體,主管會依據(jù)工具模式來確定其輸入。此外,LangGraph允許在運行時將狀態(tài)傳遞給各個工具,因此,如果有需要,從屬智能體可以訪問父狀態(tài)。
4. 結(jié)論
正如本文所探討的,多智能體大語言模型(LLM)系統(tǒng)通過利用諸如并行、順序、路由和聚合器工作流等多樣化的架構(gòu)模式,為處理復(fù)雜任務(wù)提供了強大的范例。
通過對共享狀態(tài)、消息列表和工具調(diào)用等通信機制的詳細研究,我們了解到智能體是如何協(xié)作以實現(xiàn)無縫協(xié)調(diào)的。
本文轉(zhuǎn)載自??柏企閱文??,作者:柏企
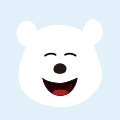